DSJA: Distributed Server-Driven Joint Route Scheduling and Streaming Adaptation for Multi-Party Realtime Video Streaming
IEEE Transactions on Mobile Computing(2023)
摘要
The widespread availability of convenient wireless network connection and video capture have fueled the development of multi-party realtime video streaming (MRVS) services, such as Zoom or Microsoft Teams. These services have transformed the generation and distribution of realtime streaming content and offer a new way of online communication, striving to provide high Quality-of-Experience (QoE) for individuals. However, delivering high QoE in MRVS is more challenging than in traditional video scenarios due to the stringent delay requirements and complex multi-party interactive architectures. In this paper, we propose DSJA, a distributed server-driven multi-party realtime video streaming framework that conquers the challenges. We first design an appropriate QoE model for MRVS services to capture the interplay among perceptual quality, variations, bitrate mismatch, loss damage, and streaming delay. We then model the QoE maximization problem in MRVS as a route scheduling and streaming adaptation problem. Afterward, we design DSJA which seamlessly integrates multiple selective forwarding units (SFU) architecture and server-driven approaches based on a two-step solution of route scheduling and streaming adaptation. DSJA first determines the most suitable SFU and streaming routes for each video session based on SFUs' job queuing delay and path latency. Then, the server conducts joint loss and bitrate adaptation decisions to optimize the streaming configuration of all clients, considering network conditions and QoE preferences. Our evaluations show that our framework outperforms state-of-the-art solutions by
$23.1\% \sim 41.7\%$
from the perspective of QoE, and reduces the backbone network transmission by
$14.0\% \sim 36.6\%$
.
更多查看译文
关键词
Adaptive bitrate control,multi-party realtime video streaming,quality of experience
AI 理解论文
溯源树
样例
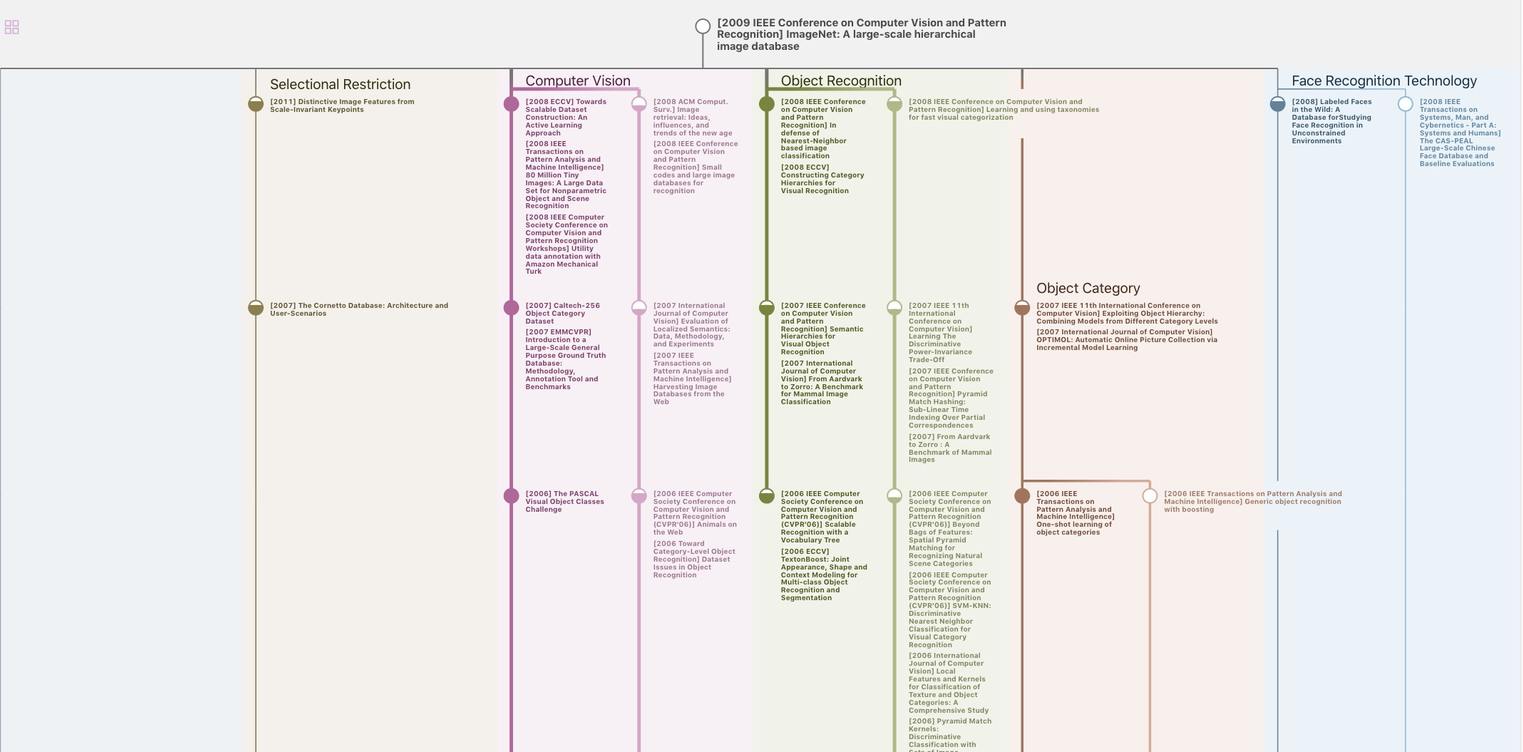
生成溯源树,研究论文发展脉络
Chat Paper
正在生成论文摘要