AdaPyramid: Adaptive Pyramid for Accelerating High-resolution Object Detection on Edge Devices
IEEE Transactions on Mobile Computing(2023)
摘要
Deep convolutional neural network (NN)-based object detectors are not appropriate for straightforward inference on high-resolution videos at edge devices, as maintaining high accuracy often brings about prohibitively long latency. Although existing solutions have attempted to reduce on-device inference latency by selecting a cheaper configuration (e.g., choosing a more lightweight NN or scaling a frame to a smaller size before inference) or eliminating a background containing no object, they often ignore various high-resolution features and fail to optimize for those videos. We thus present AdaPyramid, a framework to reduce as much on-device inference latency as possible, especially for high-resolution videos, while achieving the accuracy demand approximately. We observe that the cheapest configuration to achieve the accuracy demand varies significantly across both different frames and different regions in a frame. The underlying reason is that object features (e.g., the location, size and category of objects) are more uneven in high-resolution videos, both temporally and spatially. Moreover, we observe that the object size presents a prominent hierarchical distribution in high-resolution frames. AdaPyramid thus partitions each frame hierarchically just like a pyramid and chooses a content-aware configuration for each region, which is adapted online based on the feedback. We evaluate the performance of AdaPyramid on a public dataset and our collected real-world videos. The obtained results show that under comparable accuracy to the state-of-the-art solutions, AdaPyramid can decrease inference latency by 40% on average, with up to 2.5× speed-up.
更多查看译文
关键词
Edge computing,neural networks,video analytics,object detection,online adaptation
AI 理解论文
溯源树
样例
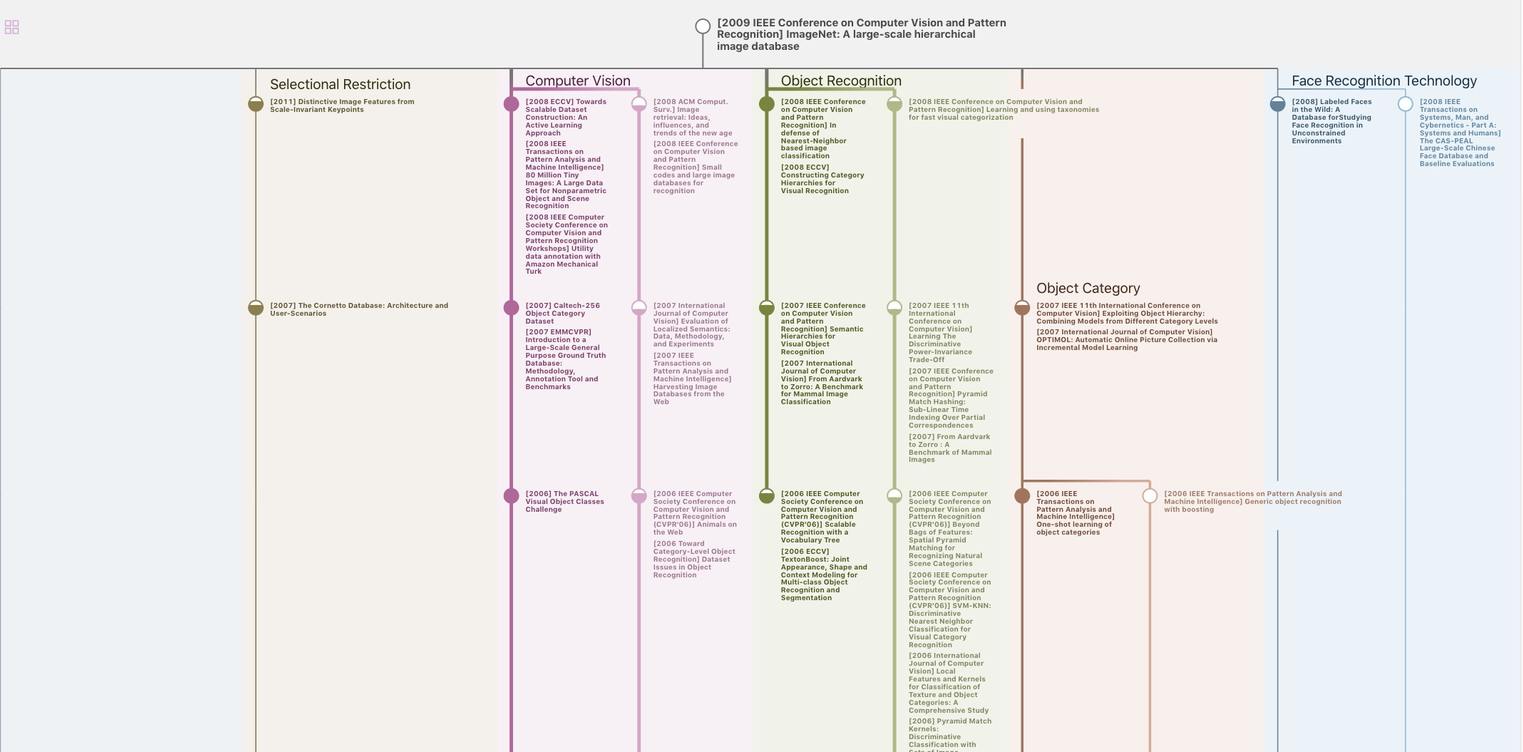
生成溯源树,研究论文发展脉络
Chat Paper
正在生成论文摘要