Multi-View Interactive Representations for Multimodal Sentiment Analysis
IEEE Transactions on Consumer Electronics(2024)
摘要
Multimodal Sentiment Analysis (MSA) technology, prevalent in consumer applications and mobile edge computing (MEC), enables sentiment examination through user data collected by smart devices. Despite the focus on representation learning in MSA, current methods often prioritize recognition performance through modality interaction and fusion. However, they struggle to capture multi-view sentiment cues across different interaction states, limiting multimodal sentiment representations’ expressiveness. This paper develops an innovative MSA framework, MVIR, learning multi-view interactive representations in diverse interaction states. Multilple meticulously designed sentiment tasks and an introduced self-supervised label generation algorithm (SSLGM) enable a comprehensive understanding of multi-view sentiment tendencies. The dual-view attention weighted fusion (DVAWF) module is designed to facilitate inter-modality information exchange in different interaction states. Extensive experiments on three MSA datasets affirm the efficacy and superiority of MVIR, showcasing its ability to capture sentiment information from multimodal data across various interaction states.
更多查看译文
关键词
Representation learning,dual-view attention weighted fusion,multi-task learning,multimodal sentiment analysis
AI 理解论文
溯源树
样例
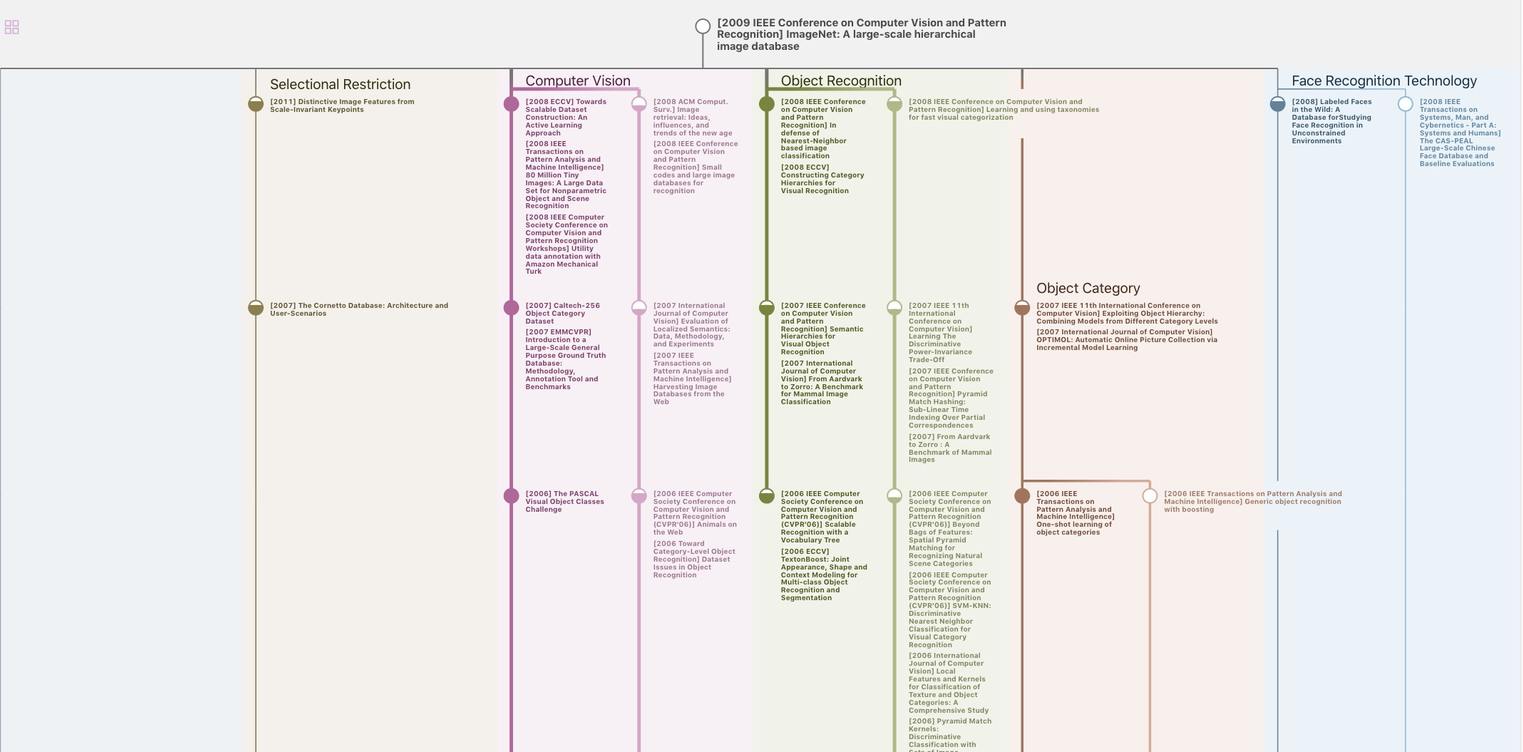
生成溯源树,研究论文发展脉络
Chat Paper
正在生成论文摘要