Deep Learning Model for Interpretability and Explainability of Aspect-Level Sentiment Analysis Based on Social Media
IEEE TRANSACTIONS ON COMPUTATIONAL SOCIAL SYSTEMS(2024)
摘要
The interactive attention graph convolution network (IAGCN), a novel model proposed in this article, will revolutionize aspect-level sentiment analysis (SA). IAGCN effectively addresses these key features, in contrast to prior research that ignored the meaning of aspect terms and their relationship with context. The model combines a modified dynamic weighting layer with bidirectional long short-term memory (BiLSTM) to accurately acquire context. It takes use of graph convolutional networks (GCNs) to encrypt syntactic information from the syntactic dependency tree. Furthermore, a method for interactive attention is employed to discover the intricate relationships between context and aspect terms, which results in the reconstruction of those terms' representations. Comparing the proposed IAGCN model to baseline models, impressive gains are made. Across five datasets, the model beats previous methods with an amazing improvement in F1 scores that ranges from 1.34% to 4.04% and an impressive improvement in accuracy that ranges from 0.56% to 1.75%. Additionally, the IAGCN model outperforms the global vectors (GloVe)-based strategy when the potent pretrained model bidirectional encoder representations from transformers (BERT) is included in the challenge, resulting in even greater improvements. The F1 score considerably increases from 2.59% to 7.55%, and accuracy increases from 1.47% to 3.95%, making the IAGCN model a standout performer in aspect-level SA.
更多查看译文
关键词
Context modeling,Semantics,Syntactics,Sentiment analysis,Deep learning,Analytical models,Image color analysis,Artificial intelligence,aspect-level sentiment analysis,global vectors,interactive attention graph convolution network,social networks
AI 理解论文
溯源树
样例
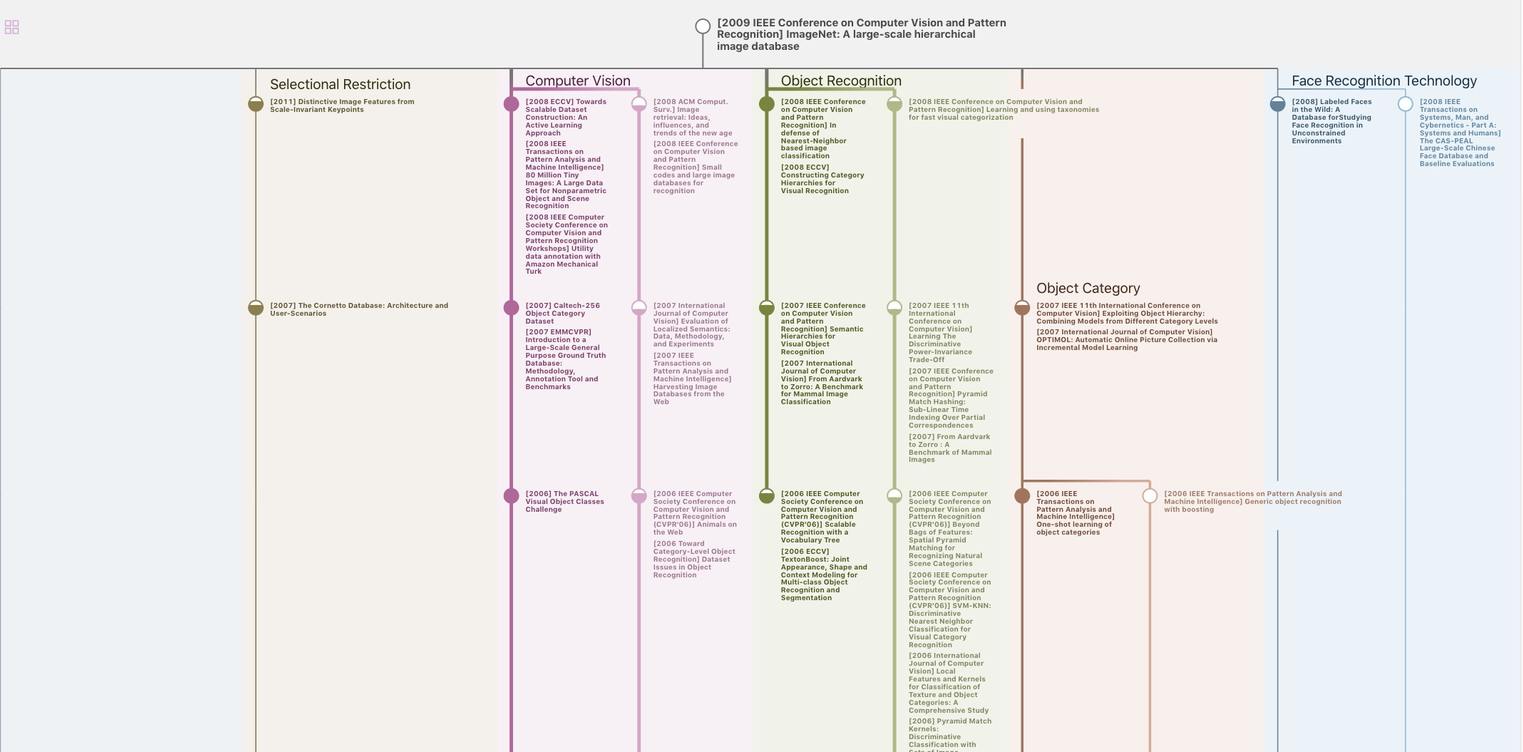
生成溯源树,研究论文发展脉络
Chat Paper
正在生成论文摘要