Optimization for Dynamic Laser Inter-Satellite Link Scheduling with Routing: A Multi-Agent Deep Reinforcement Learning Approach
IEEE Transactions on Communications(2023)
摘要
Laser inter-satellite links (LISLs) have greatly extended communication distance between satellites, allowing for establishment of dynamic links to reduce communication delay. However, a closed-loop control is required for LISL, which causes high energy consumption. Proper scheduling of dynamic LISLs can effectively reduce energy consumption and communication delay. In this study, a satellite link mode with three fixed LISLs and one dynamic LISL is designed, and its feasibility is analyzed. The optimization problem is formulated and transformed into a Markov decision process (MDP) by modeling it as a sequential decision. By decomposing states, actions, and reward functions, the MDP is divided into the proposed multi-agent deep reinforcement learning (MADRL). Moreover, compressed sensing is utilized to cut down state information to reduce communication, storage, and computation overhead. Furthermore, network parameters and experience sharing, and prioritized experience replay have been adopted to improve stability and convergence speed of network training with a large number of agents. Experimental results show that under different routing strategies, the proposed MADRL can reduce energy consumption by over 15% and delay by approximately two hops compared to fixed LISLs scenario within several iterations.
更多查看译文
关键词
Laser inter-satellite link,dynamic link,multi-agent deep reinforcement learning,link scheduling,routing strategy
AI 理解论文
溯源树
样例
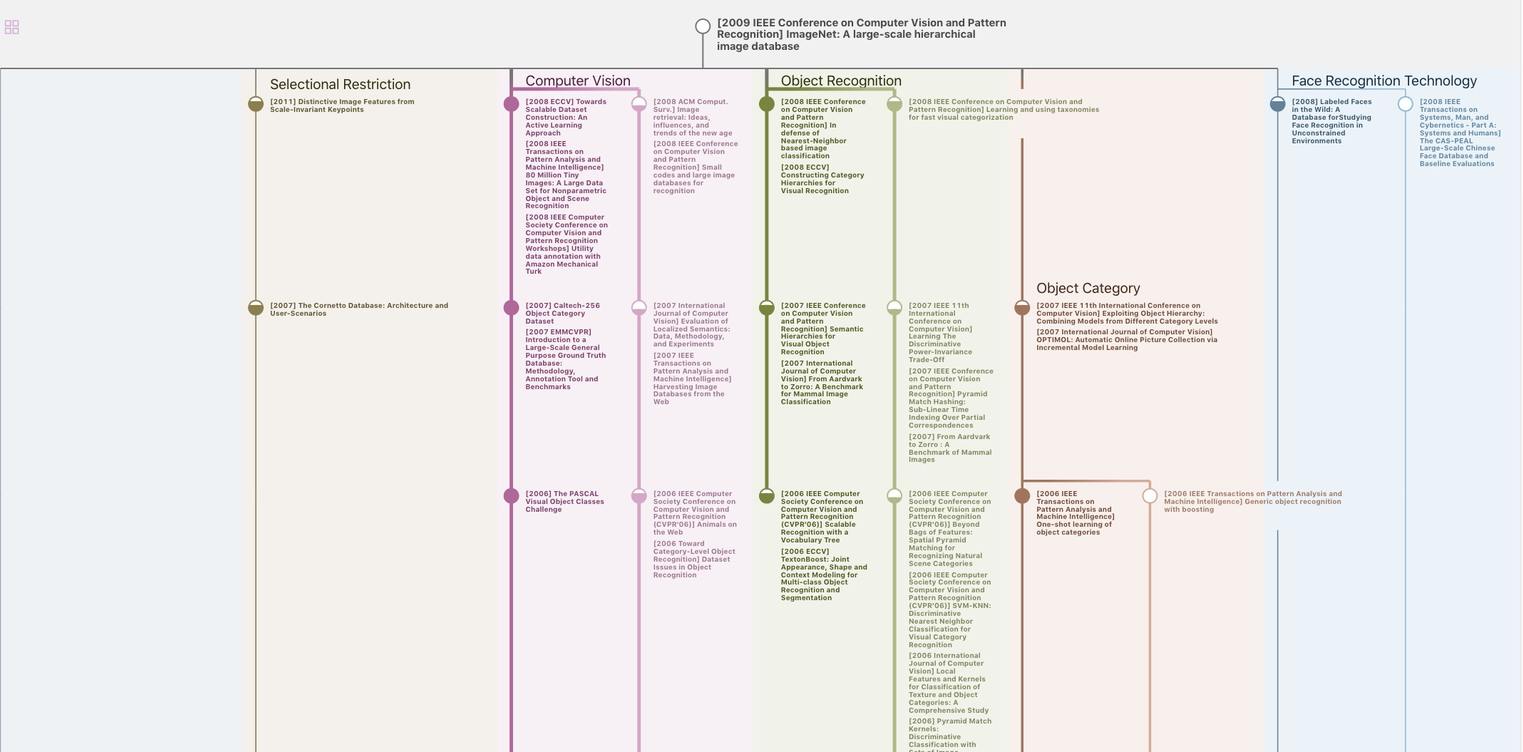
生成溯源树,研究论文发展脉络
Chat Paper
正在生成论文摘要