Low-Delay Ultra-Small Packet Transmission with In-Network Aggregation via Distributed Stochastic Learning
IEEE Transactions on Communications(2024)
摘要
In-network aggregation is a fundamental operation for massive packets in the Internet of Things (IoT). By aggregating ultra-small packets, the energy consumption for data transmission is not related to the packet number, while the average delay performance depends on the delay of all packets even if they are aggregated. In this paper, we propose a low-delay ultra-small packet transmission scheme with in-network aggregation in energy-harvesting multi-hop networks, where each device periodically transmits packets in a collect-wait-forward relaying manner. Considering the resulting extra waiting time during relaying, we first drive the tractable form of the average end-to-end delay by problem transformation. By characterizing the two-dimensional evolution property from the perspective of both hops and time, the delay minimization problem is reformulated as an infinite-horizon average-cost Markov decision process with a two-dimensional optimality equation. To deal with the curse of dimensionality, we decompose the global Bellman equation into several per-device local relay selection problems. Based on the problem decomposition, we propose a distributed ultra-Small Packet Aggregation Relay SElection (SPARSE) algorithm via stochastic learning. The convergence is further proved theoretically and verified by simulation. Simulation results reveal that the proposed scheme achieves significant performance gain over the baselines for ultra-small packets.
更多查看译文
关键词
Relay selection,delay optimization,in-network aggregation,Markov decision process
AI 理解论文
溯源树
样例
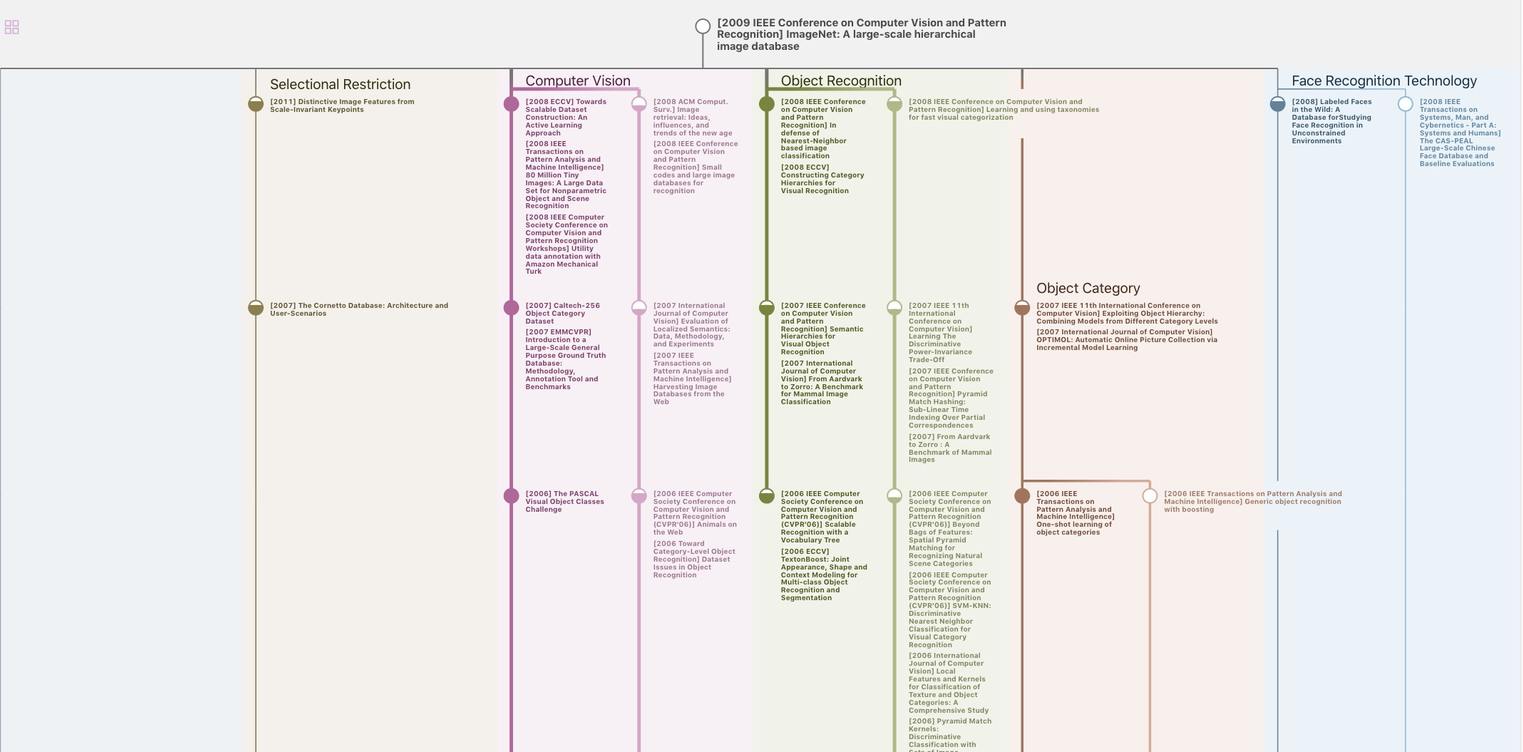
生成溯源树,研究论文发展脉络
Chat Paper
正在生成论文摘要