Learning the Error Features of Approximate Multipliers for Neural Network Applications
IEEE TRANSACTIONS ON COMPUTERS(2024)
摘要
Approximate multipliers (AMs) have widely been investigated to pursue high-performance and energy-efficient hardware designs for error-tolerant applications, such as neural networks (NNs). The computing accuracy of an AM has been evaluated by using statistical error features; however, it is difficult to estimate the quality of a specific application using AMs. Thus, it is a great challenge to select or design appropriate AMs for an accuracy-constrained application. This paper proposes an application-oriented error evaluation framework for AMs with the aim of exploring the correlation between statistical error features of AMs and the accuracy degradation in AM-based NN applications. Specifically, based on the Dropout Feature Ranking technique, statistical error features of AMs are extensively studied and ranked by their importance to the accuracy of AM-based NN applications. The three most informative features are obtained to construct error models to predict the accuracy loss of AM-based NN applications. The constructed classification models show a probability higher than 96% for correctly classifying the AMs into three categories in accordance with the induced accuracy loss in AM-based NN applications. Furthermore, regression models can predict the accuracy of NN applications using an AM with a deviation as low as 6%. These results show that the proposed error evaluation framework can guide an efficient selection of AMs for NN applications by using just several AM error features, instead of running time-consuming and complicated hardware simulation. The obtained statistical error features can also provide a guidance for the design or generation of application-oriented AMs. Moreover, the proposed framework is applicable for quickly analyzing and selecting other approximate circuits for error-tolerant applications.
更多查看译文
关键词
Hardware,Integrated circuit modeling,Predictive models,Degradation,Computational modeling,Training,Prediction algorithms,Approximate multiplier,statistical error feature,error model,accuracy degradation,machine learning
AI 理解论文
溯源树
样例
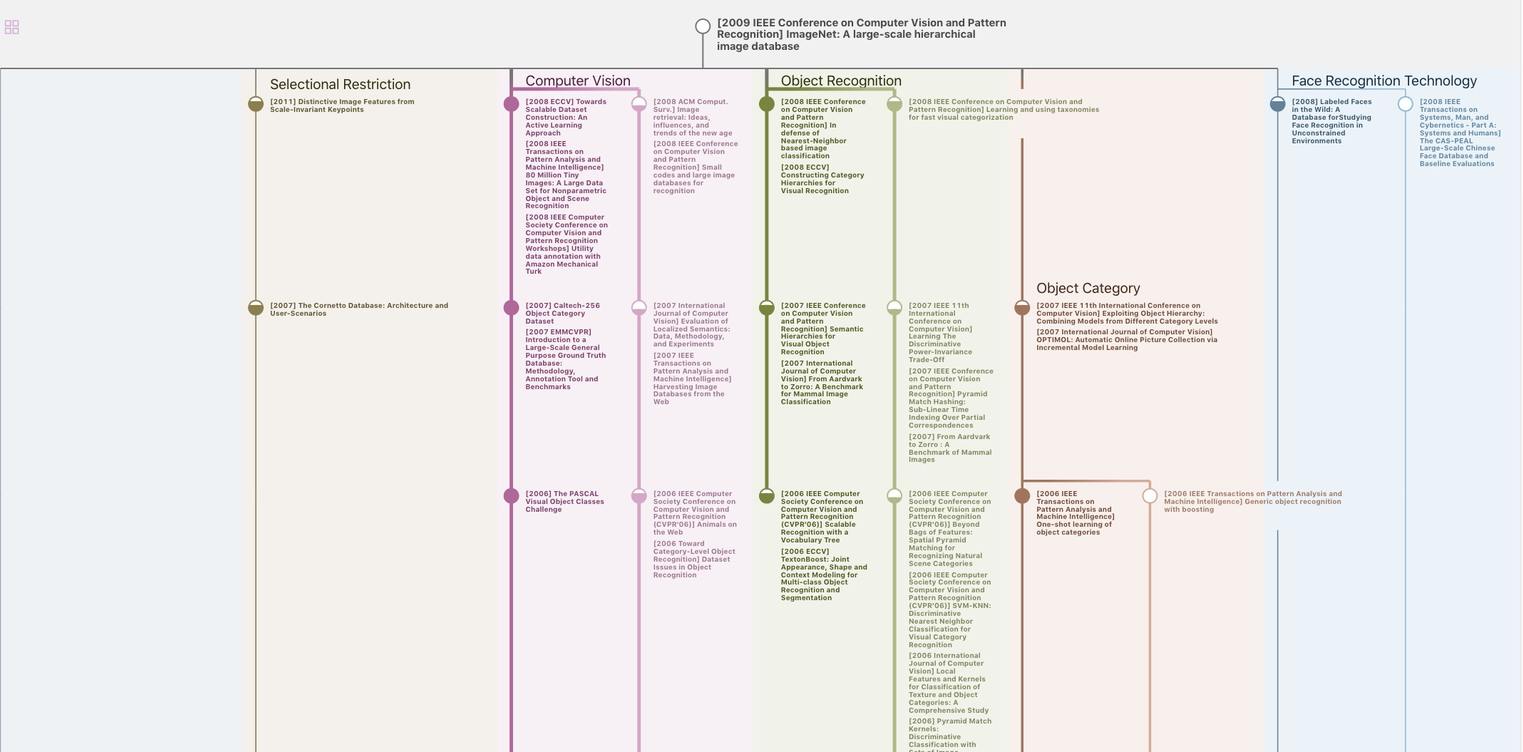
生成溯源树,研究论文发展脉络
Chat Paper
正在生成论文摘要