A Lump Coal Detection Method Fusion of Lightweight and Attention Mechanism
IEEE Journal of Emerging and Selected Topics in Industrial Electronics(2024)
摘要
During the transfer process for coal with the utilization of mining conveyor belts, large pieces of coal often affect the safety of transportation, so real-time monitoring of the transport process in lump coal is essential. Therefore, a real-time monitoring method GSB YOLOv5 is proposed. Firstly, the dataset's contrast is enhanced by adaptive histogram equalization, while its richness is improved by combining Mosaic multi-data enhancement. Secondly, it proposes the utilization of Ghost Net, which is a neural network with low computational requirements for lightweight extraction and fusion of features. This approach effectively minimizes the model computation. In addition, the combination of Squeeze-Excitation mechanism improves the extraction of model's feature capabilities. Finally, a feature pyramid with weighted bidirectional is employed to accomplish multi-source information fusion by effectively merging features at varying resolutions. The experimental findings demonstrate that the enhanced GSB YOLOv5 algorithm achieves a 35.256% significant reduction in network layers, while it has substantial reductions of 63.023% and 68.582% in parameters and floating point operations, respectively. Furthermore, the compressed model size is decreased from 92.7MB to 34.4MB. In addition, there are improvements of 1.421% and 1.460% in the detection precision and recall rate of the model, respectively, while the detection efficiency of the real-time has a significant boost from 68.34 FPS to 107.91 FPS. Automatic, fast and high precision monitoring of lump coal objects on conveyor belts in underground coal mines can be realized.
更多查看译文
关键词
Real-time monitoring,Lightweight,Lump coal,Deep learning,Attentional Mechanisms
AI 理解论文
溯源树
样例
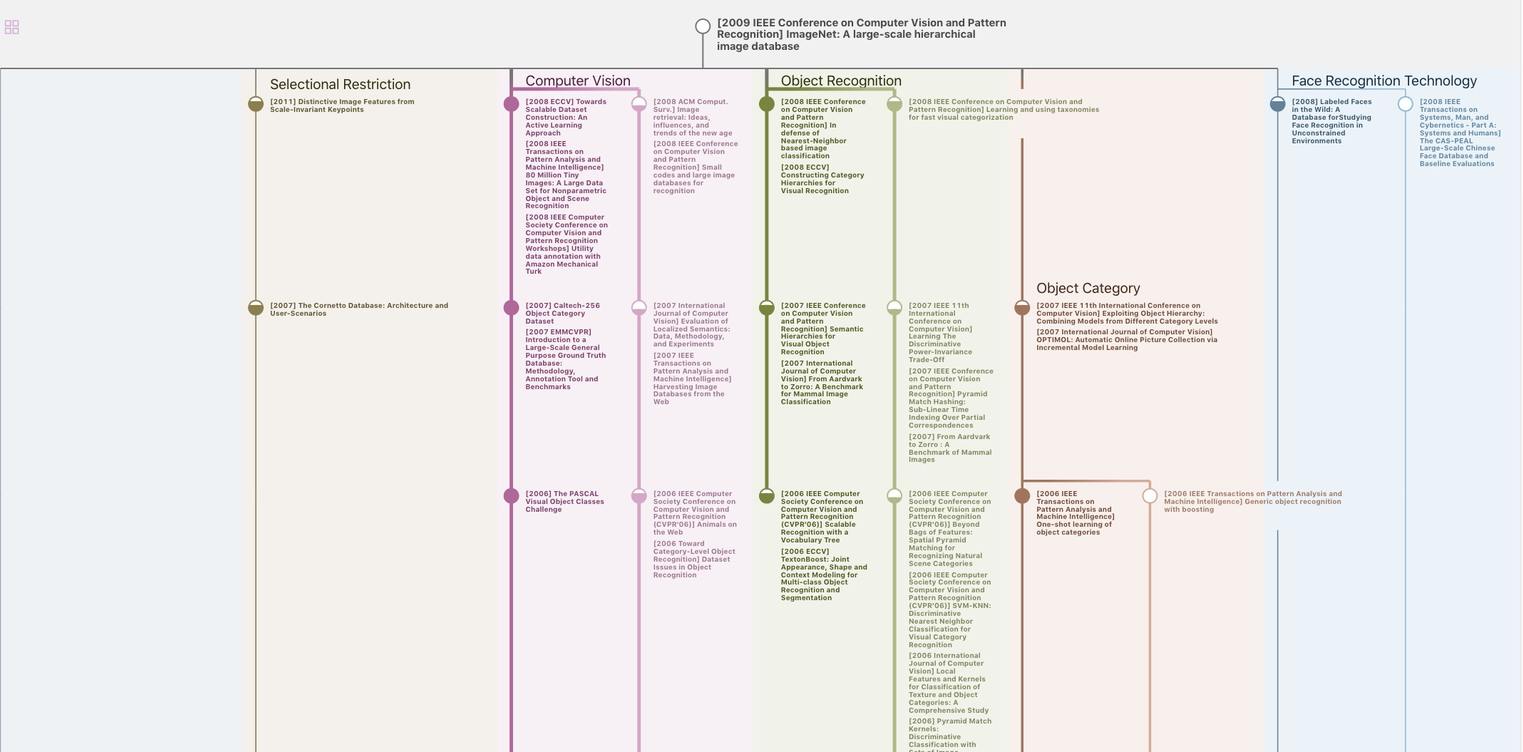
生成溯源树,研究论文发展脉络
Chat Paper
正在生成论文摘要