DNN-Based Optimization to Significantly Speed Up and Increase the Accuracy of Electronic Circuit Design
IEEE TRANSACTIONS ON CIRCUITS AND SYSTEMS I-REGULAR PAPERS(2024)
摘要
Efficient design and optimization of flip-flops can significantly affect overall circuit performance as they have many applications in digital systems which can impact the overall power consumption and timings of the emerging system on chips (SOCs). In this paper, modeling, design, and optimization of transmission gate-based master-slave positive-edge-triggered flip-flop (TGFF) in 16 nm complementary metal-oxide semiconductor (CMOS) is proposed. The proposed deep neural network (DNN)-based optimization method first generates an accurate model for different performance metrics by using the training data obtained from transistor-level models which are over 100 times faster than them. Then, these accurate DNN-based models are used to optimize design goals such as dynamic and static power, setup time, and propagation delay (Data to Output). Using these fast, accurate models significantly speed up the design procedure and leads to a considerably more optimized design. Additionally, as the DNN is a universal approximator that can catch any nonlinear input-output relationship, the proposed method can be used to optimize circuits for any performance metric, even if no analytical formula is available. Additionally, circuit design based on the proposed method is automated which, facilitates the tasks of circuit designers.
更多查看译文
关键词
Optimization,Measurement,Flip-flops,Integrated circuit modeling,Logic gates,Mathematical models,Delays,Computer-aided design,circuit design optimization,circuit simulation,deep neural network (DNN),flip-flop circuits,gate sizing,nanometer regime technologies
AI 理解论文
溯源树
样例
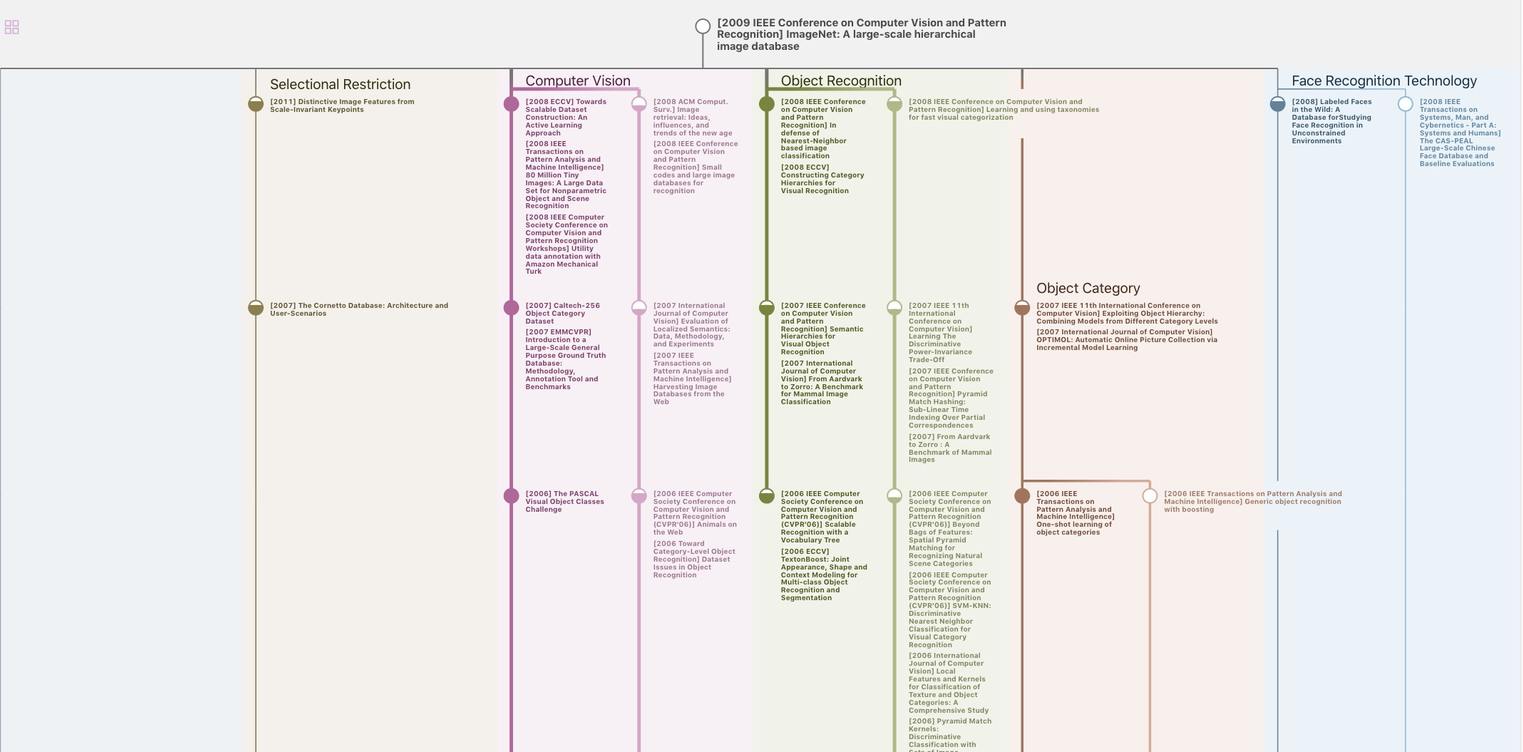
生成溯源树,研究论文发展脉络
Chat Paper
正在生成论文摘要