MetaGON: A Lightweight Pedestrian Re-Identification Domain Generalization Model Adapted to Edge Devices
IEEE OPEN JOURNAL OF THE COMMUNICATIONS SOCIETY(2024)
摘要
Pedestrian re-identification (Re-ID) leverages cross-camera data acquired by the Internet of Things (IoT) devices and sensors to identify, monitor, and analyze pedestrians, allowing IoT applications to provide more intelligent, secure, and tailored services. Current pedestrian Re-ID research faces many challenges, such as low image resolution, perspective changes, posture changes, light changes, and occlusions, resulting in models trained on other datasets being unable to be directly applied and showing poor generalization capabilities. In addition, IoT edge devices are often limited by processing power and memory capacity and cannot withstand complex and large deep learning models. Therefore, designing a lightweight and generalizable pedestrian Re-ID model is better suited for implementation on edge devices. Considering these issues, this study presents MetaGON, a lightweight model with cross-domain generalization capabilities, which combines the lightweight omni-scale network (OSNet) with the meta-learning method and Cycle Generative Adversarial Network (CycleGAN) to perform domain generalization. The model's generalization is enhanced through the simulation of the two stages of domain generalization in the meta-learning pipeline, where the obtained losses from meta-training and meta-testing are utilized for model optimization. Moreover, CycleGAN is employed to enhance and introduce style variations to the source data. The proposed MetaGON model is tested on a railway station re-identification dataset, and the model is deployed to edge devices for evaluation, which verifies the effectiveness of the algorithm.
更多查看译文
关键词
Domain generalization,meta-learning,pedestrian re-identification,Internet of Things
AI 理解论文
溯源树
样例
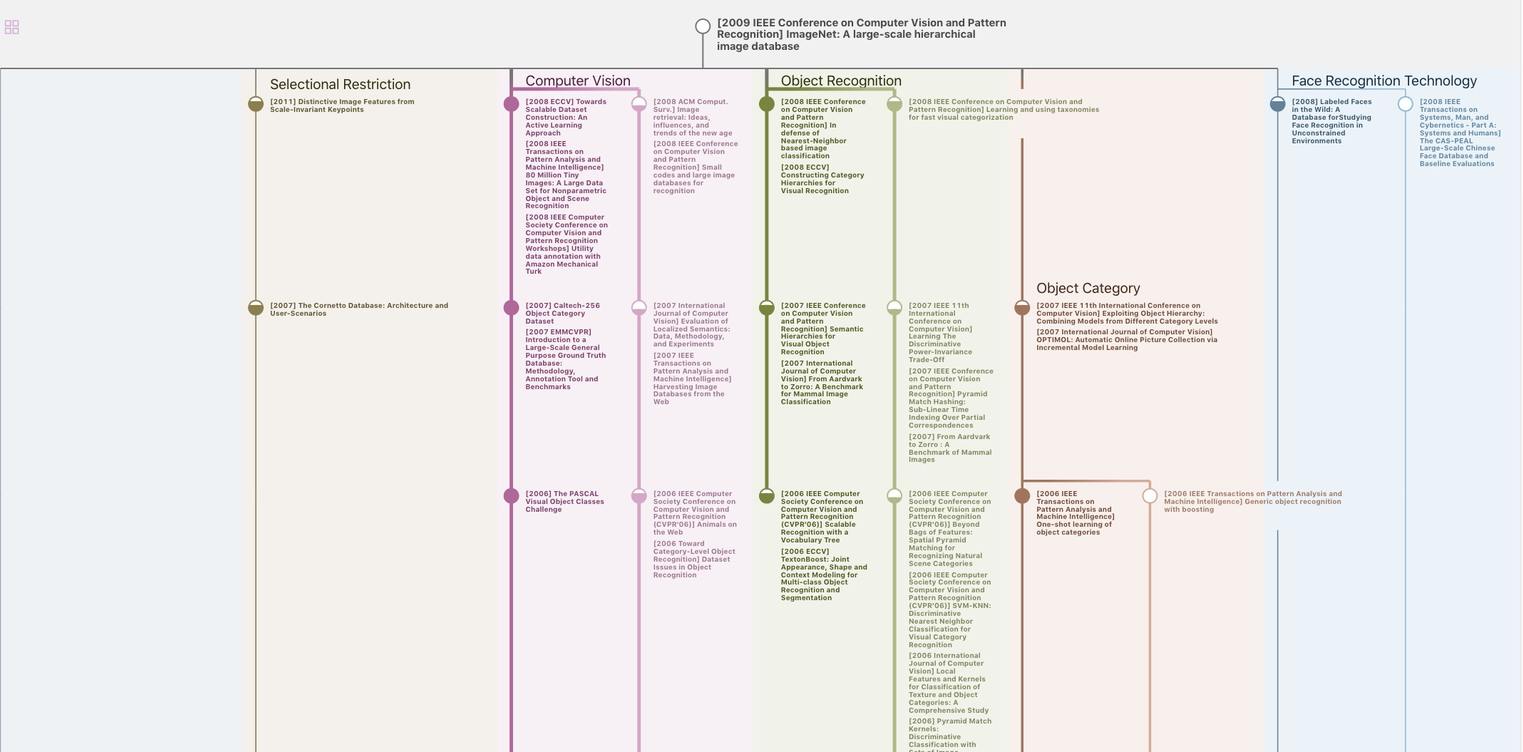
生成溯源树,研究论文发展脉络
Chat Paper
正在生成论文摘要