Kalman Filter Auto-Tuning With Consistent and Robust Bayesian Optimization.
IEEE Trans. Aerosp. Electron. Syst.(2024)
摘要
The nonlinear and stochastic relationship between noise covariance parameter values and state estimator performance makes optimal filter tuning a very challenging problem. Popular optimization-based tuning approaches can easily get trapped in local minima, leading to poor noise parameter identification and suboptimal state estimation. Recently, black box techniques based on Bayesian optimization with Gaussian processes (GPBO) have been shown to overcome many of these issues, using normalized estimation error squared (NEES) and normalized innovation error (NIS) statistics to derive cost functions for Kalman filter auto-tuning. While reliable noise parameter estimates are obtained in many cases, GPBO solutions obtained with these conventional cost functions do not always converge to optimal filter noise parameters and lack robustness to parameter ambiguities in time-discretized system models. This paper addresses these issues by making two main contributions. First, new cost functions are developed to determine if an estimator has been tuned correctly. It is shown that traditional chi-square tests are inadequate for correct auto-tuning because they do not accurately model the distribution of innovations when the estimator is incorrectly tuned. Second, the new metrics (formulated over multiple time discretization intervals) is combined with a Student-t processes Bayesian Optimization (TPBO) to achieve robust estimator performance for time discretized state space models. The robustness, accuracy, and reliability of our approach are illustrated on classical state estimation problems.
更多查看译文
关键词
Bayesian optimization,filter tuning,kalman filter,nonparametric regression
AI 理解论文
溯源树
样例
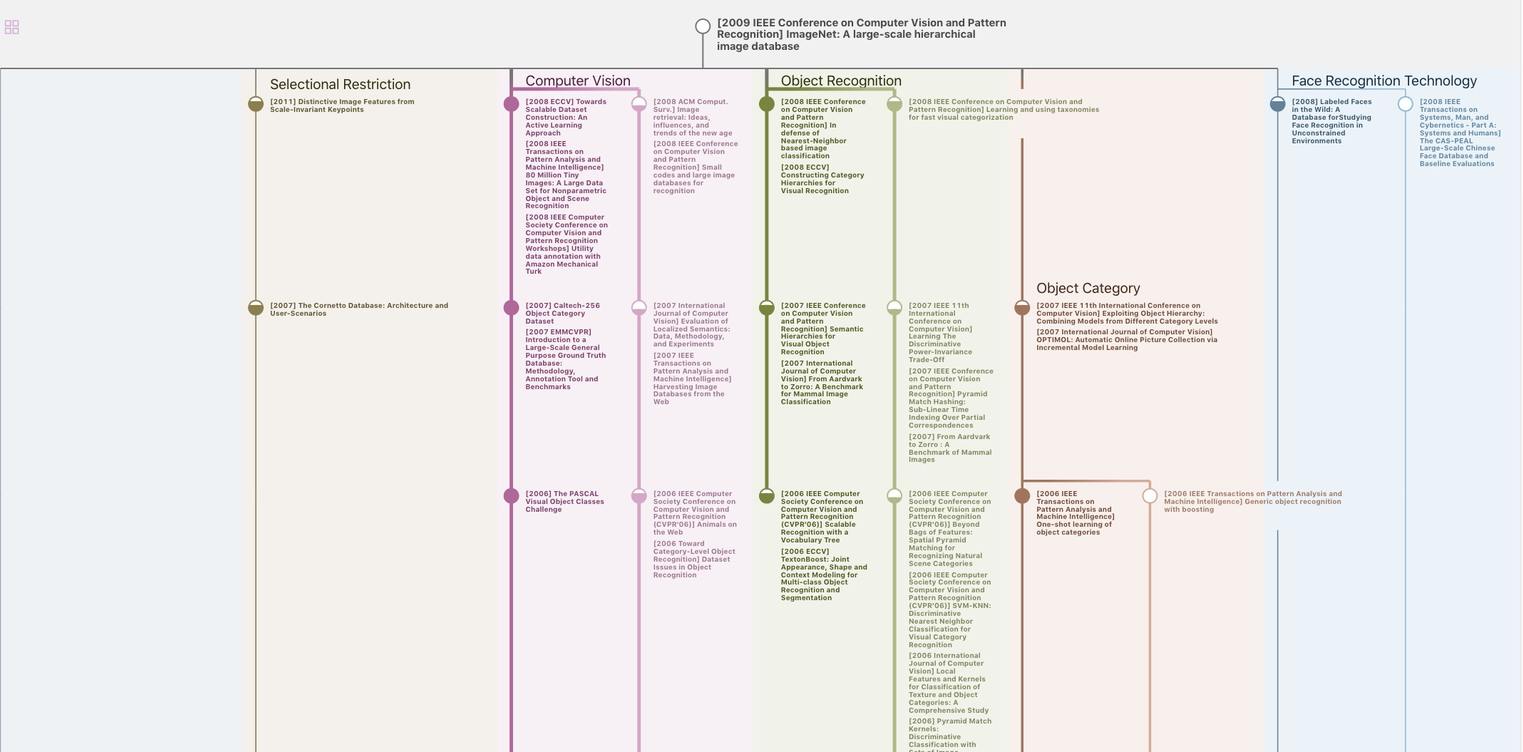
生成溯源树,研究论文发展脉络
Chat Paper
正在生成论文摘要