Two-Phase On-Line Joint Scheduling for Welfare Maximization of Charging Station
IEEE Transactions on Automation Science and Engineering(2023)
摘要
The widespread adoption of EVs brings practical interest to the operation optimization of the charging station. This paper considers the joint scheduling of pricing and charging control to enhance the operational capability of the station. The following contributions are made. First, a joint scheduling model of pricing and charging control is developed to maximize the expected social welfare of the charging station considering the quality of service and the price fluctuation sensitivity of EV drivers. It is formulated as a Markov decision process with a variance criterion to capture uncertainties during operation. Second, a two-phase on-line policy learning algorithm is proposed to solve this joint scheduling problem. In the first phase, it implements event-based policy iteration to find the optimal pricing scheme. In the second phase, it implements scenario-based model predictive control for smart charging under the updated pricing scheme. Third, by leveraging the performance difference theory, the optimality of the proposed algorithm is theoretically analyzed. The feasibility of the proposed method and the improved social welfare of the charging station are numerically demonstrated based on a typical charging station with distributed generation and storage.
Note to Practitioners
—The popularization of EVs requires the high-efficiency operation of the charging station. The joint scheduling of pricing and charging control can provide a promising way to achieve the balance between EV drivers’ satisfaction and the profit maximization of the charging station. However, it suffers from EV drivers’ uncertain responses to the pricing scheme and the coupled relationship between pricing and charging control. This multi-stage stochastic programming is non-trivial to solve. In this paper, we propose a two-phase on-line policy learning method for this joint scheduling problem. This algorithm can be implemented in the controller of the charging station. In the first phase, the event-based policy iteration can be implemented to iteratively improve the current best pricing scheme until convergence. In the second phase, the scenario-based model predictive control, which is reformulated as a mixed integer linear programming, can be quickly solved for smart charging. Case studies demonstrate the operation enhancement of the station.
更多查看译文
关键词
Electric vehicle,Markov decision process,discrete event dynamic systems,event-based optimization
AI 理解论文
溯源树
样例
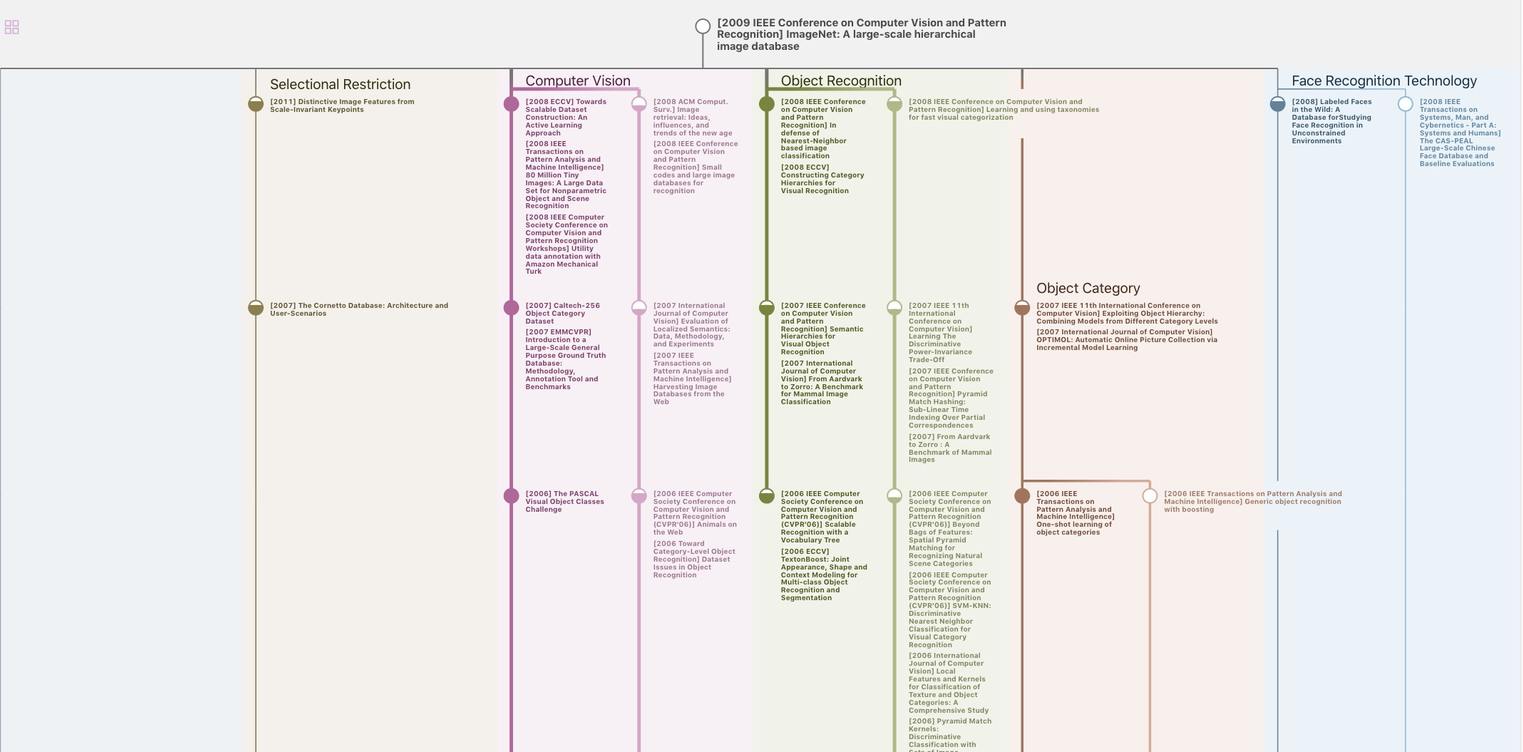
生成溯源树,研究论文发展脉络
Chat Paper
正在生成论文摘要