An Improvement Method of Target Tracking Based on Broad Learning System with Scale and Drift Correction
IEEE Transactions on Cognitive and Developmental Systems(2023)
摘要
Target tracking is difficult to achieve high accurate and efficient results due to the cases of occlusion, scale variation, and fast motion in the tracking process. In recent years, feature fusion and bounding box refinement are used as an extent to improve the accuracy of tracking. However, these new methods generally require multi-level linkage and off-line training, which need long training time, and affects the portability of the algorithm. In this paper, a novel method is proposed to improve the tracking accuracy using broad learning system (BLS). The proposed method trains Intersection over Union (IoU) network based on BLS, which is called BLIoU for scale and drift correction in target tracking. BLIoU learns target features and IoU discrimination ability of bounding box through network training, which can be combined with any baseline tracking methods. BLIoU performs scale correction in the case of accurate positioning of the baseline tracker and drift correction in the case of inaccurate positioning. In Experiments, several benchmark datasets are used, BLIoU improves the tracking performance of the baseline tracker through scale and drift correction, with short training time and strong portability.
更多查看译文
关键词
Target tracking,broad learning system,IoU discriminant,scale and drift correction
AI 理解论文
溯源树
样例
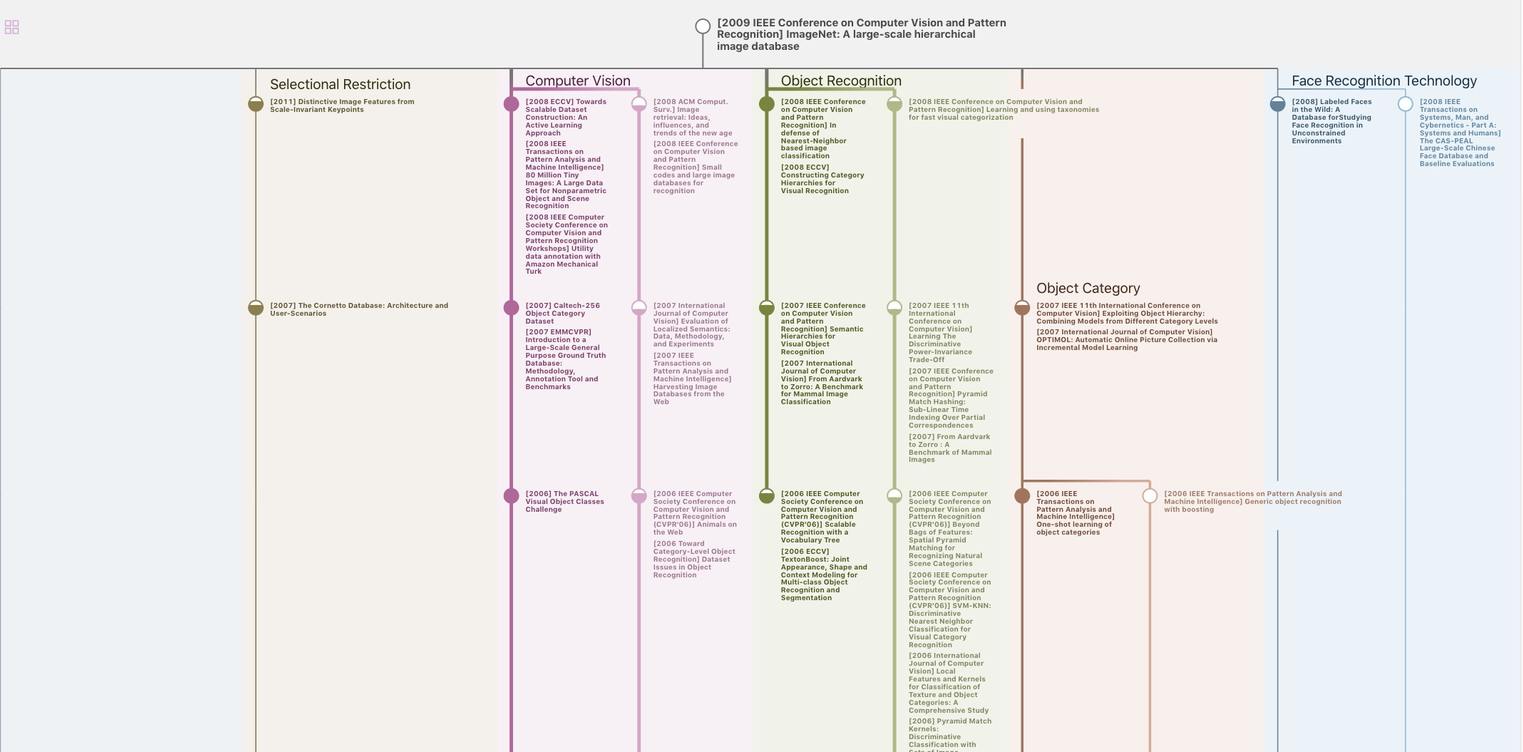
生成溯源树,研究论文发展脉络
Chat Paper
正在生成论文摘要