Kernel Ridge Regression-based Randomized Network for Brain Age Classification and Estimation
IEEE Transactions on Cognitive and Developmental Systems(2024)
摘要
Accelerated brain aging and abnormalities are associated with variations in brain patterns. Effective and reliable assessment methods are required to accurately classify and estimate brain age. In this study, a brain age classification and estimation framework is proposed using structural magnetic resonance imaging (sMRI) scans, a 3D convolutional neural network (3D-CNN), and a kernel ridge regression-based random vector functional link (KRR-RVFL) network. We used 480 brain MRI images from the publicly available IXI database and segmented them into gray matter (GM), white matter (WM), and cerebrospinal fluid (CSF) images to show age-related associations by region. Features from MRI images are extracted using 3D-CNN and fed into the wavelet KRR-RVFL network for brain age classification and prediction. The proposed algorithm achieved high classification accuracy, 97.22%, 99.31%, and 95.83% for GM, WM, and CSF regions, respectively. Moreover, the proposed algorithm demonstrated excellent prediction accuracy with a mean absolute error (MAE) of 3.89 years, 3.64 years, and 4.49 years for GM, WM, and CSF regions, confirming that changes in WM volume are significantly associated with normal brain aging. Additionally, voxel-based morphometry (VBM) examines age-related anatomical alterations in different brain regions in GM, WM, and CSF tissue volumes.
更多查看译文
关键词
cerebrospinal fluid (CSF),gray matter (GM),magnetic resonance imaging (MRI),kernel ridge regressionrandom vector functional link (KRR-RVFL),white matter (WM)
AI 理解论文
溯源树
样例
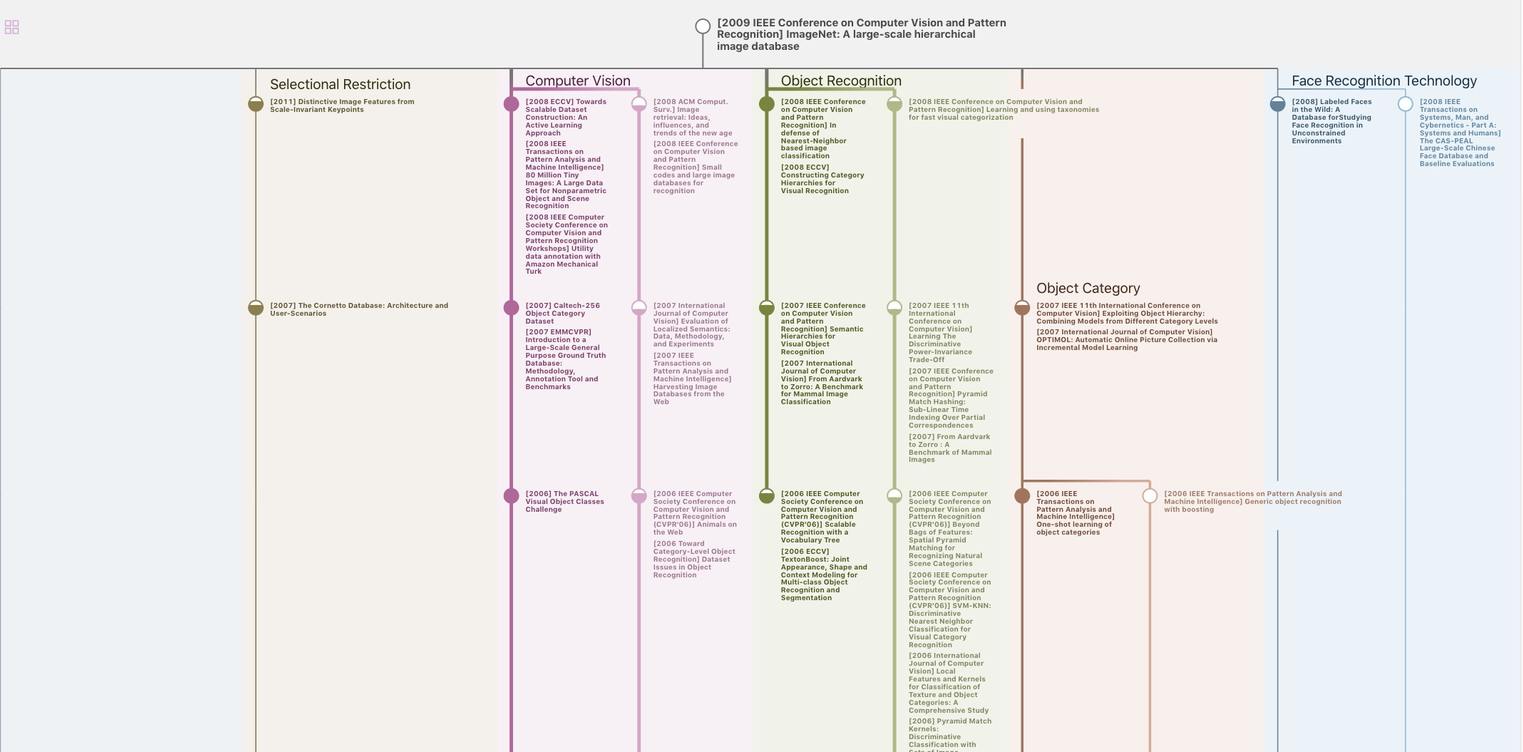
生成溯源树,研究论文发展脉络
Chat Paper
正在生成论文摘要