Active Learning-Based Sample Selection for Label-Efficient Blind Image Quality Assessment
IEEE Transactions on Circuits and Systems for Video Technology(2023)
摘要
Despite the considerable effort devoted to high-generalizable blind image quality assessment (BIQA), the generalization performance of the state-of-the-art metrics remains limited when facing new visual scenes. A straightforward way to address the dilemma is labeling a great number of images from the new scene and subsequently training a new model, which is quite labor-intensive and cost-expensive. Hence, there is an urgent need to mitigate the dependency on labeled samples by designing a data-efficient BIQA algorithm. Motivated by the above facts, this paper presents an Active Learning-based IQA (AL-IQA) framework, which reduces the requirement for training samples by selecting representative images from two perspectives, including distortion and content. Specifically, in terms of distortion, we design distortion prompts and adopt Contrastive Language-Image Pre-Training (CLIP) to predict image distortion in a zero-shot manner. Then, we employ curriculum learning-inspired strategy to select samples with gradually increasing difficulty (measured by prediction uncertainty of CLIP), in order to facilitate model training. Meantime, in terms of content, we adopt distribution matching-based dataset distillation to distill unlabeled images into several high-density informative synthetic images. Then, feature distances between unlabeled images and distilled images are compared to identify images with the most representative content. Finally, Borda count is adopted to capture a consensus of both distortion and content through weighted counting, and prompt tuning is utilized for adapting the model to the IQA task. Extensive experiments are conducted on five IQA datasets, and the results demonstrate that the proposed AL-IQA not only effectively reduces the number of training samples but also achieves state-of-the-art prediction accuracy and generalization performance. The source code is available at https://github.com/esnthere/AL-IQA.
更多查看译文
关键词
image quality assessment,active learning,generalization,data-efficient
AI 理解论文
溯源树
样例
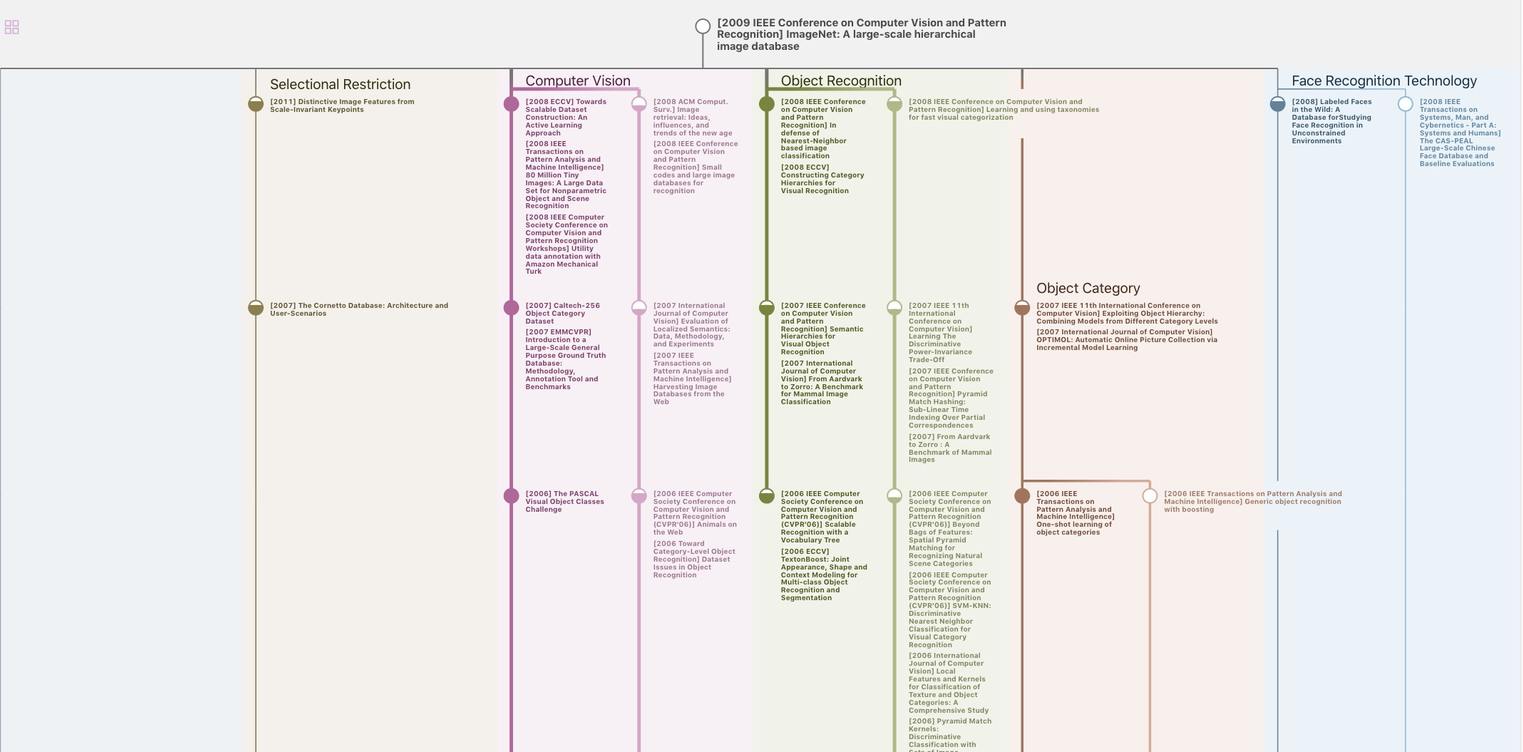
生成溯源树,研究论文发展脉络
Chat Paper
正在生成论文摘要