Unveiling the Power of Visible-Thermal Video Object Segmentation
IEEE Transactions on Circuits and Systems for Video Technology(2023)
摘要
Despite recent progress, Video Object Segmentation (VOS) remains challenging in complex situations such as low light and dark scenes. In this paper, we tackle the visibility limitations by introducing thermal information as auxillary for VOS. Specifically, we generate a hybrid benchmark dataset for Visible-Thermal VOS, named VisT300, which contains 300 challenging videos with visible light and thermal frames and corresponding object mask annotations. Besides, a Visible-Thermal integration Network, named as VTiNet, is proposed to use both cross-modal and cross-frame propagation for accurate video object segmentation. It is advantageous in two aspects: 1) effective cross-modal feature fusion and propagation for strong expressions on visible, thermal, and fused modalities; 2) effective modality-sensitive memory bank enables preserving the most valuable historical contexts in each modality. Extensive experiments demonstrate our VTiNet outperforms the state-of-the-art VOS works by a large margin (over 5% than RGB SotAs in Mean J&F). Our preliminary research clearly recovers that importing complementary modalities can effectively increase the strength of models to achieve robust segmentation in challenging scenarios. Data and code are released at https://github.com/yjybuaa/vtinet, and we hope this work will promote the progress of visible-thermal VOS.
更多查看译文
关键词
Multi-modal learning,Video object segmentation,Visible-thermal fusion
AI 理解论文
溯源树
样例
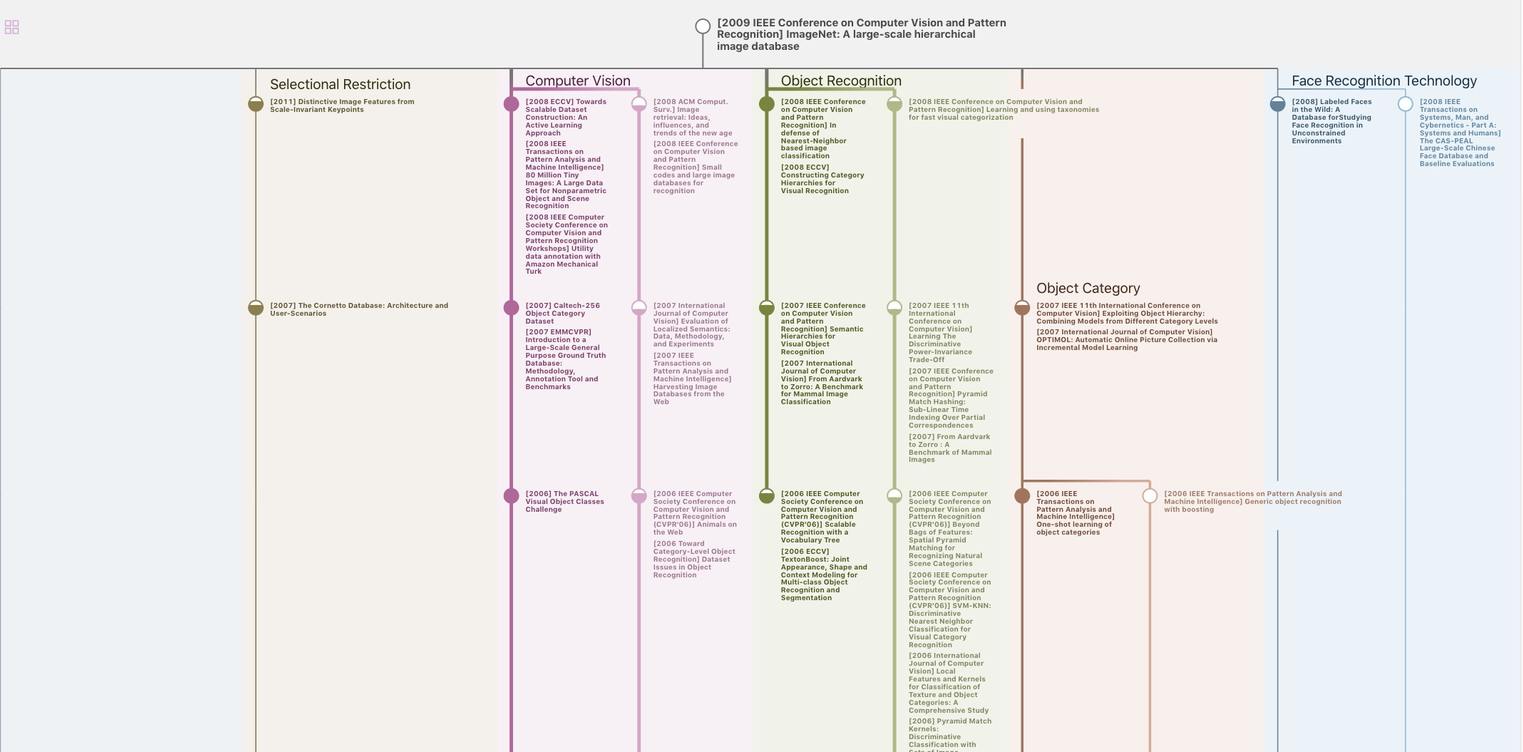
生成溯源树,研究论文发展脉络
Chat Paper
正在生成论文摘要