AutoDDI: Drug-Drug Interaction Prediction With Automated Graph Neural Network
IEEE JOURNAL OF BIOMEDICAL AND HEALTH INFORMATICS(2024)
摘要
Drug-drug interaction (DDI) has attracted widespread attention because when incompatible drugs are taken together, DDI will lead to adverse effects on the body, such as drug poisoning or reduced drug efficacy. The adverse effects of DDI are closely determined by the molecular structures of the drugs involved. To represent drug data effectively, researchers usually treat the molecular structure of drugs as a molecule graph. Then, previous studies can use the handcrafted graph neural network (GNN) model to learn the molecular graph representations of drugs for DDI prediction. However, in the field of bioinformatics, manually designing GNN architectures for specific molecular structure datasets is time-consuming and depends on expert experience. To address this problem, we propose an automatic drug-drug interaction prediction method named AutoDDI that can efficiently and automatically design the GNN architecture for drug-drug interaction prediction without manual intervention. To this end, we first design an effective search space for drug-drug interaction prediction by revisiting various handcrafted GNN architectures. Then, to efficiently and automatically design the optimal GNN architecture for each drug dataset from the search space, a reinforcement learning search algorithm is adopted. The experiment results show that AutoDDI can achieve the best performance on two real-world datasets. Moreover, the visual interpretation results of the case study show that AutoDDI can effectively capture drug substructure for drug-drug interaction prediction.
更多查看译文
关键词
Drugs,Graph neural networks,Task analysis,Convolution,Estimation,Computer architecture,Predictive models,Drug-drug interaction,graph neural network,graph neural architecture search,reinforcement learning
AI 理解论文
溯源树
样例
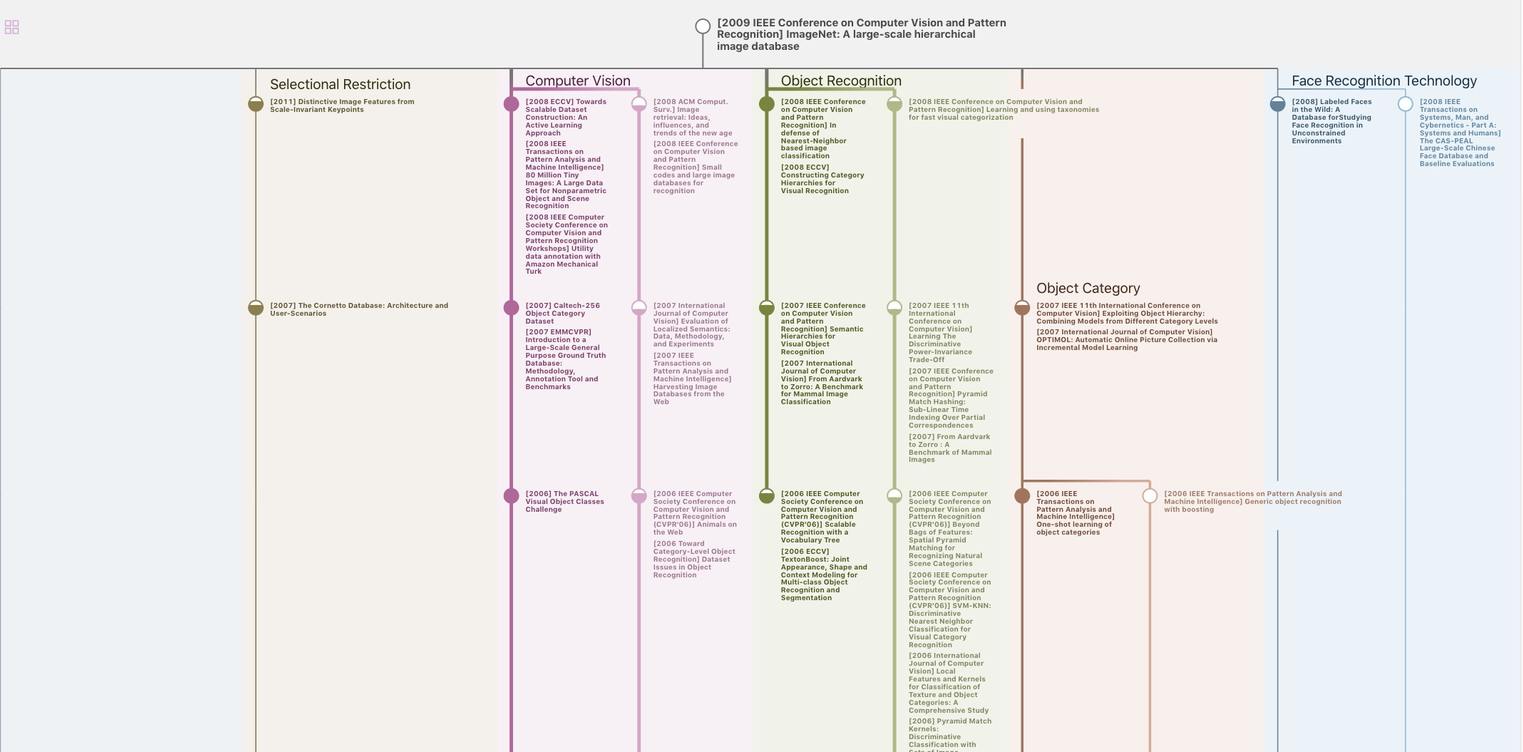
生成溯源树,研究论文发展脉络
Chat Paper
正在生成论文摘要