Sequential Datum–wise Feature Acquisition and Classifier Selection
IEEE Transactions on Artificial Intelligence(2023)
摘要
We present a supervised machine learning framework for sequential datum–wise feature acquisition and classifier selection. The presented method sequentially acquires features during testing until it determines that additional features will not improve label assignment. At that stage, easy–to–classify examples are handled by a simple classifier, which assigns labels based on the lowest expected misclassification cost. On the contrary, difficult–to–classify examples are assigned a label using the acquired features along with one of a number of available complex classifiers. As more features are acquired, the presented framework continually assesses the difficulty of classifying each example. It controls both the feature acquisition and classifier selection processes through a carefully constructed optimization problem. We use eleven publicly available datasets to evaluate the presented framework with respect to accuracy and average number of acquired features, and obtain results when two and three complex classifiers are available, respectively. We compare the performance of the presented framework with both sequential feature aquisition methods and dynamic classifier selection methods, and observe improvements in accuracy as well as acquisition of less number of features on average. Moreover, we conduct experiments with popular ensemble classification methods and assess the performance of the proposed framework.
更多查看译文
关键词
supervised classification,instance–wise feature selection,inaccurate oracles,dynamic classifier selection,classifier pool
AI 理解论文
溯源树
样例
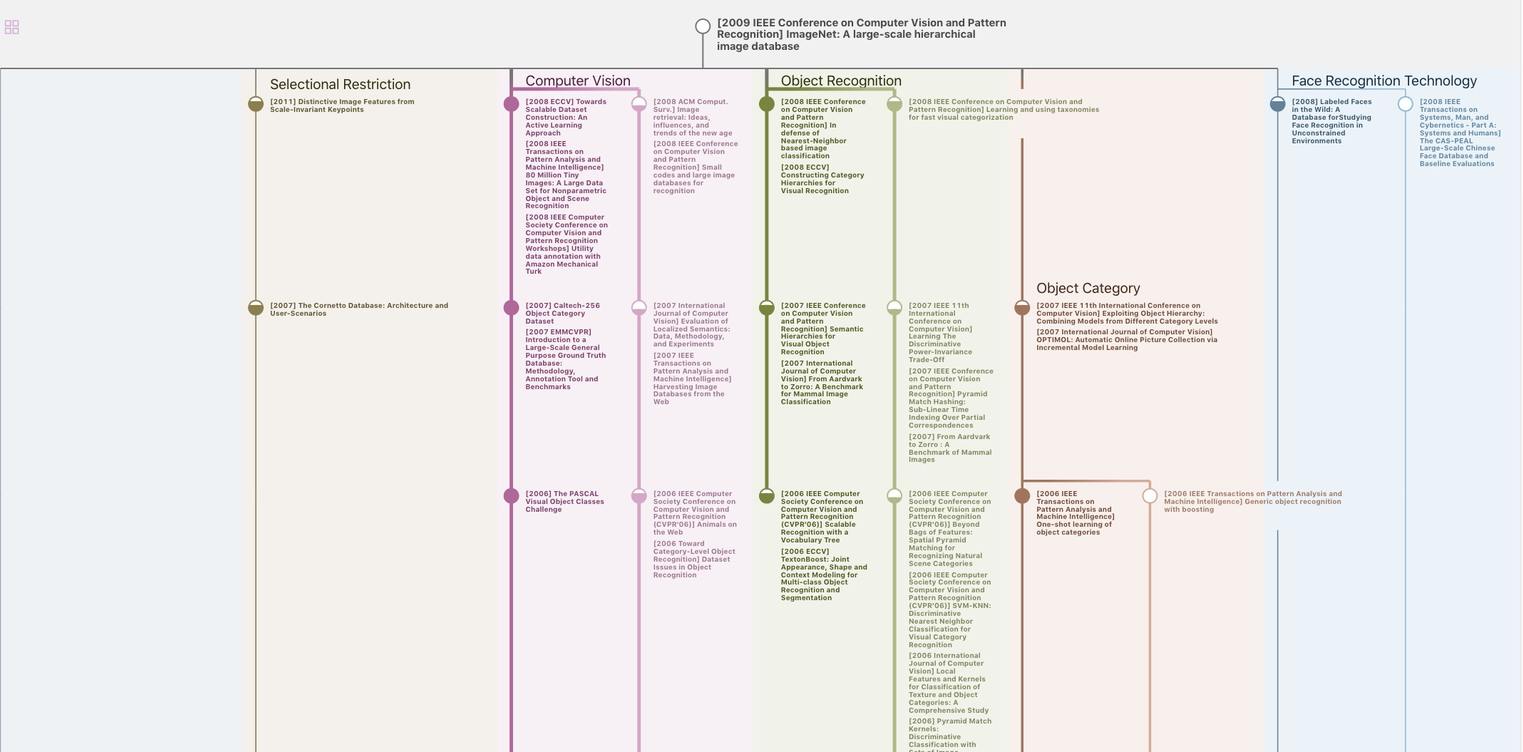
生成溯源树,研究论文发展脉络
Chat Paper
正在生成论文摘要