Development and Evaluation of Artificial Neural Networks for Real-World Data-Driven Virtual Sensors in Vehicle Suspension
IEEE ACCESS(2024)
摘要
Vehicle comfort, handling, and stability can be improved by a semi-active suspension with advanced control algorithms using the vertical velocities of the sprung mass (SM) and the unsprung masses (UMs) as inputs. Displacement and acceleration sensors are often used to estimate vertical velocities of UMs. However, these sensors are expensive and are susceptible to degradation. Virtual sensors (VS) have been proposed as a solution, and previous research using simulation data has shown that artificial neural networks (ANNs) can provide usable UM vertical velocity estimates. This study aims at finding ANNs structure and input sample window size to achieve best performance on real-world data. Novel dataset was created and used to test VSs based on eight structures of ANNs combining multilayer perceptron, convolutional neural network, long-term short-term memory (LSTM), and bidirectional LSTM layers. This article presents the results of 104 combinations of ANN structure and sample window size, which required 6240 training sessions. A Bayesian search was used to tune the hyperparameters of ANNs' layers minimizing the root-mean-square error (RMSE) of estimations on the validation data, while a grid search was used to select the sample window size that minimizes RMSE of estimation on test data, ensuring that selected combinations are well generalized. The VS based on ANN with convolutional layers, achieved the lowest RMSE of 0.0210 m/s, and processing time of 0.421 ms for a window size of 23 samples while estimating vertical velocities of vehicle UMs from real-world data.
更多查看译文
关键词
Suspensions (mechanical systems),Soft sensors,Stability analysis,Power system stability,Multilayer perceptrons,Degradation,Convolutional neural networks,Bayes methods,Artificial neural networks,Data models,Vehicle dynamics,data-driven modeling,virtual sensor,velocity estimation,vehicle suspension
AI 理解论文
溯源树
样例
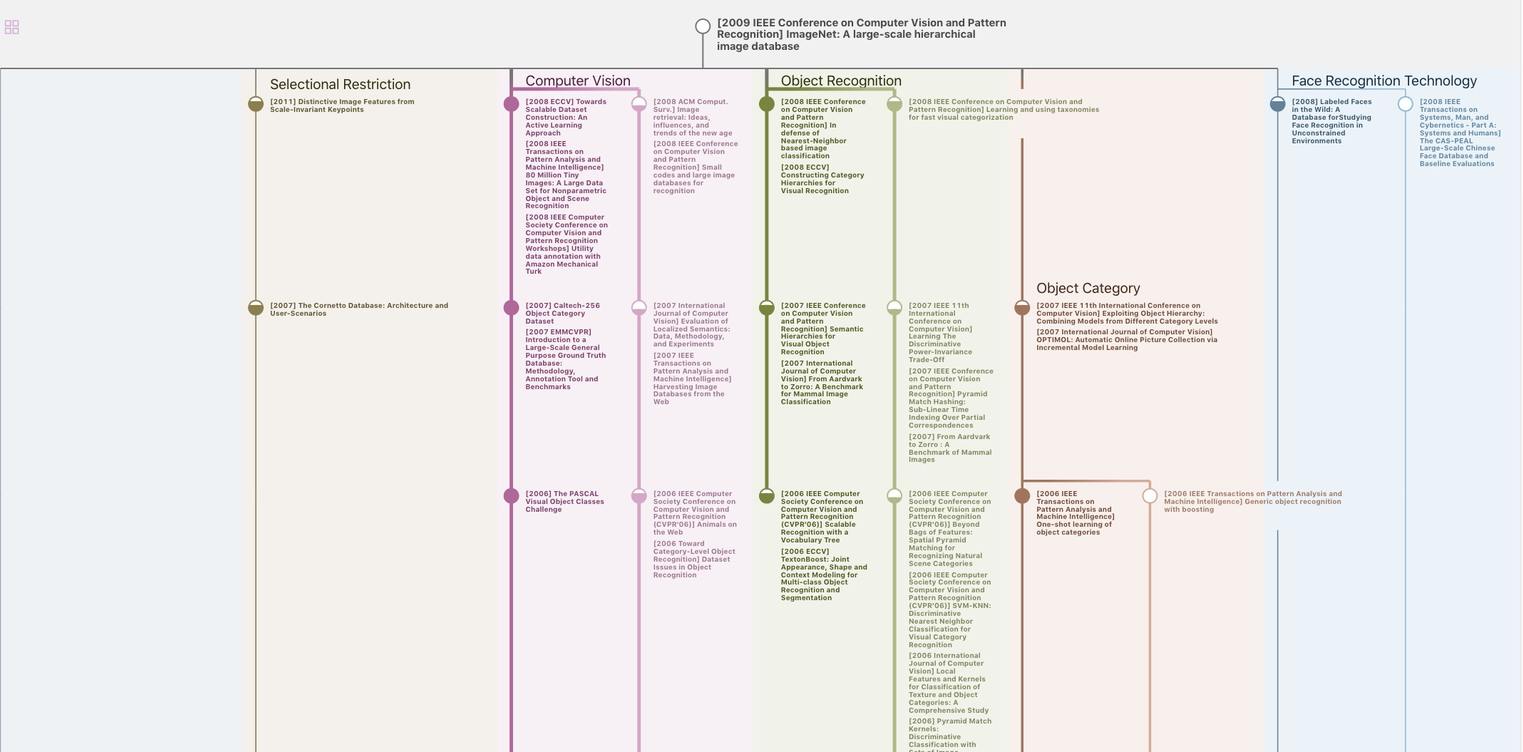
生成溯源树,研究论文发展脉络
Chat Paper
正在生成论文摘要