Wavelet and Adaptive Coordinate Attention Guided Fine-grained Residual Network for Image Denoising
IEEE Transactions on Circuits and Systems for Video Technology(2023)
摘要
Convolutional neural networks (CNN) have achieved remarkable performance in image denoising. However, most existing CNNs cannot accurately capture and remove tiny noises during the denoising process and lose edge detail information easily. In this paper, we propose a fine-grained residual network guided by wavelet and adaptive coordinate attention (WACAFRN) for image denoising. Firstly, we propose an adaptive coordinate attention mechanism and combine it with cascaded Res2Net residual blocks to form an encoder network for more accurate noise removal. Secondly, we propose a wavelet attention mechanism that combines global and local residual blocks to form a decoder network, aiming to address the problem of edge detail information loss. At last, we complement the noise information through a noise estimation block to further enhance the model’s ability to adapt to noise. Extensive experiment results demonstrate that our proposed method outperforms existing denoising methods in both qualitative and quantitative aspects. Notably, our method significantly improves real-world noise removal tasks on the CC dataset, with an average increase of 2.08 dB in PSNR and 0.0264 in SSIM over the state-of-the-art methods. Additionally, WACAFRN exhibits faster inference speeds, underscoring its efficiency in real-world applications.
更多查看译文
关键词
Image denoising,wavelet attention,adaptive coordinate attention,noise estimation,residual connection
AI 理解论文
溯源树
样例
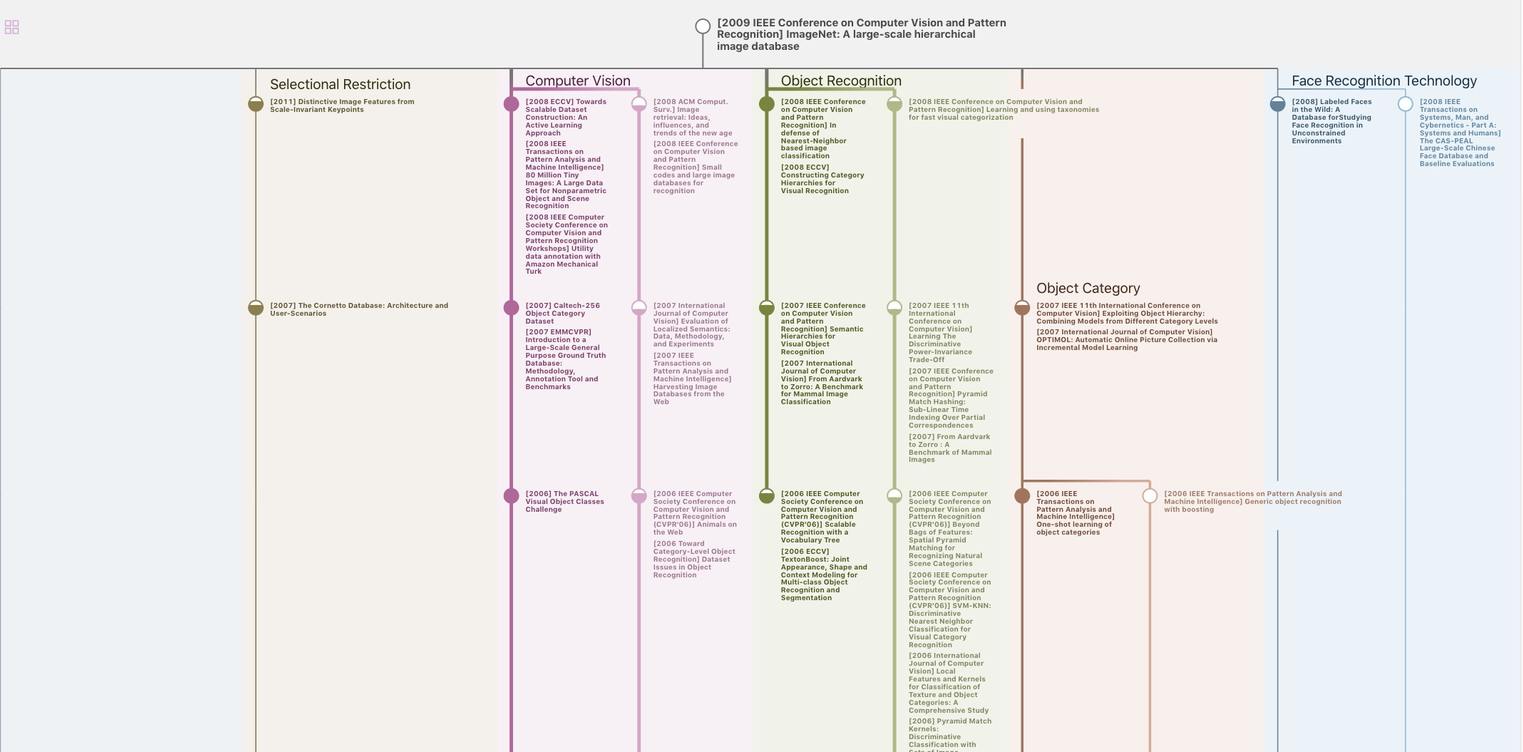
生成溯源树,研究论文发展脉络
Chat Paper
正在生成论文摘要