Comprehensive Benchmarking of CNN-Based Tumor Segmentation Methods Using Multimodal MRI Data
medrxiv(2024)
摘要
Magnetic resonance imaging (MRI) is become an essential and a frontline technique in the detection of brain tumor. However, manual segmentation of tumor from MRI scans is a time-consuming and labour-intensive process. There is a prevalent trend in employing fully automated methods for accurate tumor segmentation using MRI scans. The precision in brain tumor segmentation is essential for the better diagnosis, treatment and prognosis. This study focuses on benchmarking and evaluating the performance of four widely used convolutional neural network (CNN) based brain tumor segmentation methods CaPTk, 2DVNet, EnsembleUNets, and ResNet50. We used 1251 multimodal MRI scans from the BraTS2021 dataset, which encompasses T1, T2, T1ce, and Flair imaging modalities. We compared the performance of these CNN-based methods against a reference set of previously segmented images obtained with the assistance of radiologists. The evaluation encompasses direct utilization of segmented outputs and also by employing radiomic features. Performance evaluation with direct segments method using Dice Similarity Coefficient score (DSC) and Hausdorff Distance (HD) suggested better performance of EnsembleUNets with DSC and HD of 0.93 and 18 respectively, outperforming the other methods. Comparative analysis using radiomics features also revealed that EnsembleUNets is the most precise segmentation method as compared to CaPTk, 2DVNet, and ResNet50. EnsembleUNets achieved Concordance Correlation Coefficient (CCC), Total Deviation Index (TDI), and Root Mean Square Error (RMSE) of 0.79, 1.14 and 0.53 respectively and outperformed its counterparts. These findings contribute valuable insight into the comparative efficacy of EnsembleUNets, facilitating informed decision for accurate brain tumor segmentation.
### Competing Interest Statement
The authors have declared no competing interest.
### Funding Statement
This study did not receive any funding.
### Author Declarations
I confirm all relevant ethical guidelines have been followed, and any necessary IRB and/or ethics committee approvals have been obtained.
Yes
I confirm that all necessary patient/participant consent has been obtained and the appropriate institutional forms have been archived, and that any patient/participant/sample identifiers included were not known to anyone (e.g., hospital staff, patients or participants themselves) outside the research group so cannot be used to identify individuals.
Yes
I understand that all clinical trials and any other prospective interventional studies must be registered with an ICMJE-approved registry, such as ClinicalTrials.gov. I confirm that any such study reported in the manuscript has been registered and the trial registration ID is provided (note: if posting a prospective study registered retrospectively, please provide a statement in the trial ID field explaining why the study was not registered in advance).
Yes
I have followed all appropriate research reporting guidelines, such as any relevant EQUATOR Network research reporting checklist(s) and other pertinent material, if applicable.
Yes
The dataset analysed in this study is available in BraTS2021 dataset: http://braintumorsegmentation.org/
更多查看译文
AI 理解论文
溯源树
样例
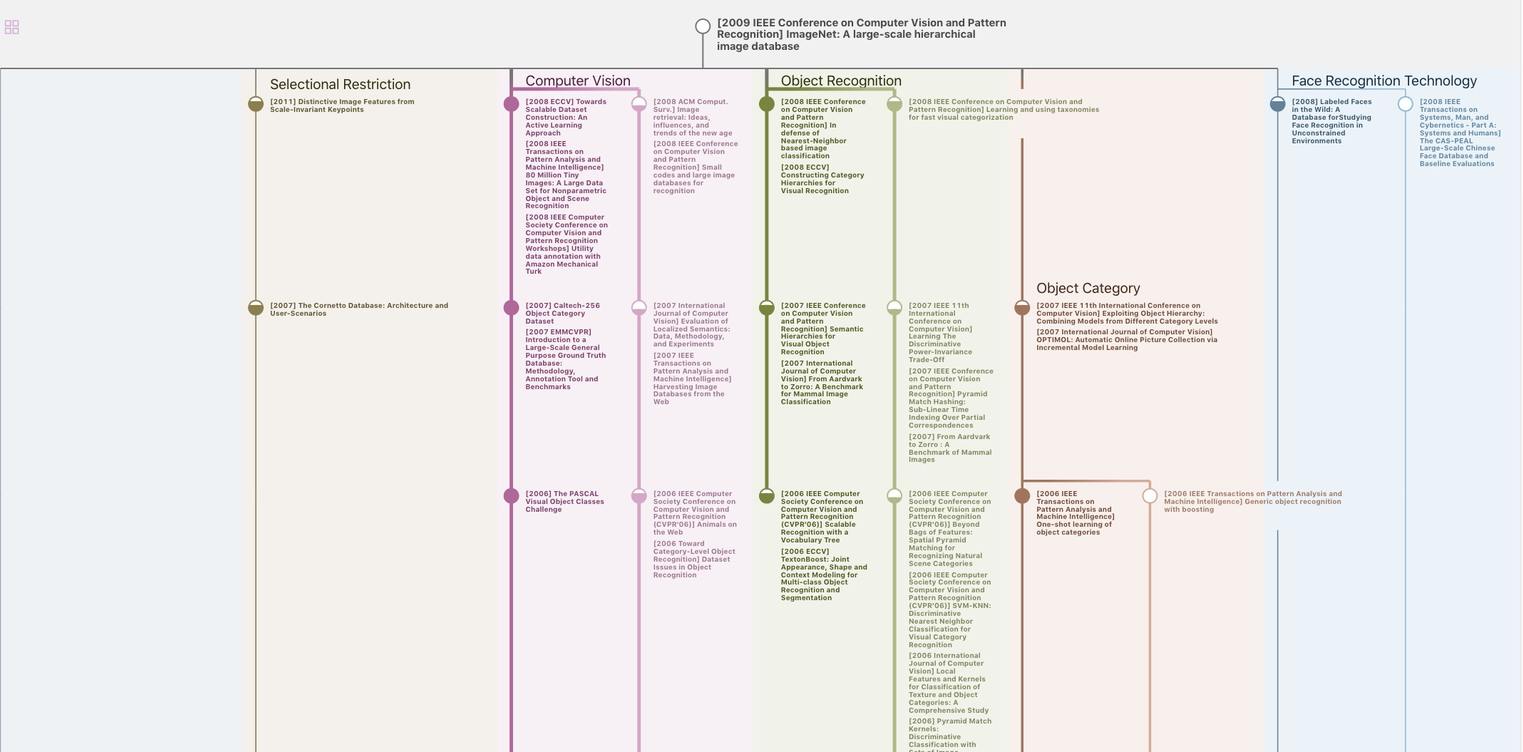
生成溯源树,研究论文发展脉络
Chat Paper
正在生成论文摘要