Two3-AnoECG: ECG anomaly detection with two-stream networks and two-stage training using two double-throw switches
KNOWLEDGE-BASED SYSTEMS(2024)
摘要
The electrocardiogram (ECG) is a highly cost-effective and convenient diagnostic tool that can aid in the diagnosis of a wide range of cardiovascular conditions, such as arrhythmias, myocardial ischemia, myocardial infarction, and heart failure. Its usefulness lies in its ability to provide information about the electrical activity and rhythm of the heart, making it an essential component of the diagnostic process for many cardiac conditions. However, the interpretation of ECG signals often requires a high level of expertise from medical professionals. When reading complex ECGs, noncardiologists often need to consult with cardiologists. Even cardiologists may make errors in their judgments after prolonged reading of ECGs. Therefore, the accurate and timely diagnosis of cardiovascular diseases using ECG signals is a complex task. In this paper, we categorize this problem as a multilabel, multidimensional time-series data anomaly detection task. We propose a method called Two3-AnoECG for ECG anomaly detection, which improves upon existing ECG feature extraction and data segmentation methods and introduces a two-stream network that is trained in two stages using two double-throw switches to control the input and model structure of each stage. We further propose Two3- EnsECG, which combines the anomaly score generated by Two3-AnoECG and multiple baseline methods, to improve the overall performance of anomaly detection in ECG signals. The experimental results demonstrate the effectiveness of our proposed method.
更多查看译文
关键词
ECG signals,Deep learning,Anomaly detection,12-lead R wave extraction,Two-stream networks,Two-stage training,Double-throw switches,Ensemble learning
AI 理解论文
溯源树
样例
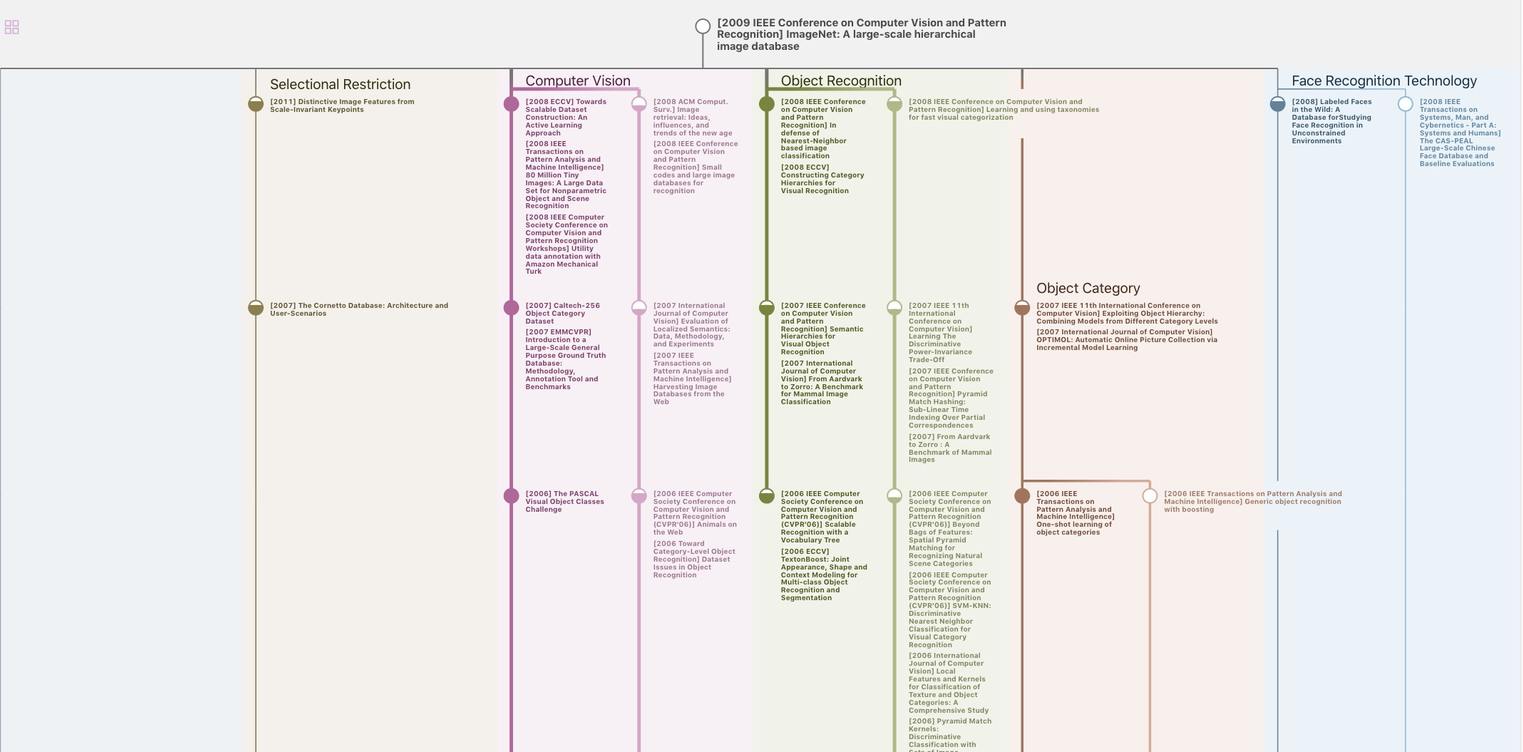
生成溯源树,研究论文发展脉络
Chat Paper
正在生成论文摘要