Use of Exposure Data to Establish Causality in Drug-Adverse Event Relationships: An Example with Desvenlafaxine
PHARMACEUTICALS(2024)
摘要
Causality algorithms help establish relationships between drug use and adverse event (AE) occurrence. High drug exposure leads to a higher likelihood of an AE being classified as an adverse drug reaction (ADR). However, there is a knowledge gap regarding what concentrations are predictive of ADRs, as this has not been systematically studied. In this work, the Spanish Pharmacovigilance System (SEFV) algorithm was used to define the relationship between the AE occurrence and drug administration in 178 healthy volunteers participating in five desvenlafaxine single-dose clinical trials, a selective serotonin and norepinephrine reuptake inhibitor that may cause dizziness, headache, nausea, dry mouth, constipation and hyperhidrosis. Eighty-three subjects presented 172 AEs that were classified as possible (101), conditional (31), unrelated (24) and probable (16). AUC infinity and Cmax were significantly higher in volunteers with vs. without ADRs (5981.24 ng center dot h/mL and 239.06 ng/mL and 4770.84 ng center dot h/mL and 200.69 ng/mL, respectively). Six of 19 subjects with conditional AEs with an SEFV score of 3 points presented an AUC infinity >= 6500 ng center dot h/mL or a Cmax >= 300 ng/mL (i.e., above percentile 75) and were summed one point on their SEFV score and classified as "possible" (4 points), improving the capacity of ADR detection.
更多查看译文
关键词
adverse event,adverse drug reactions,causality algorithms,safety,pharmacokinetics
AI 理解论文
溯源树
样例
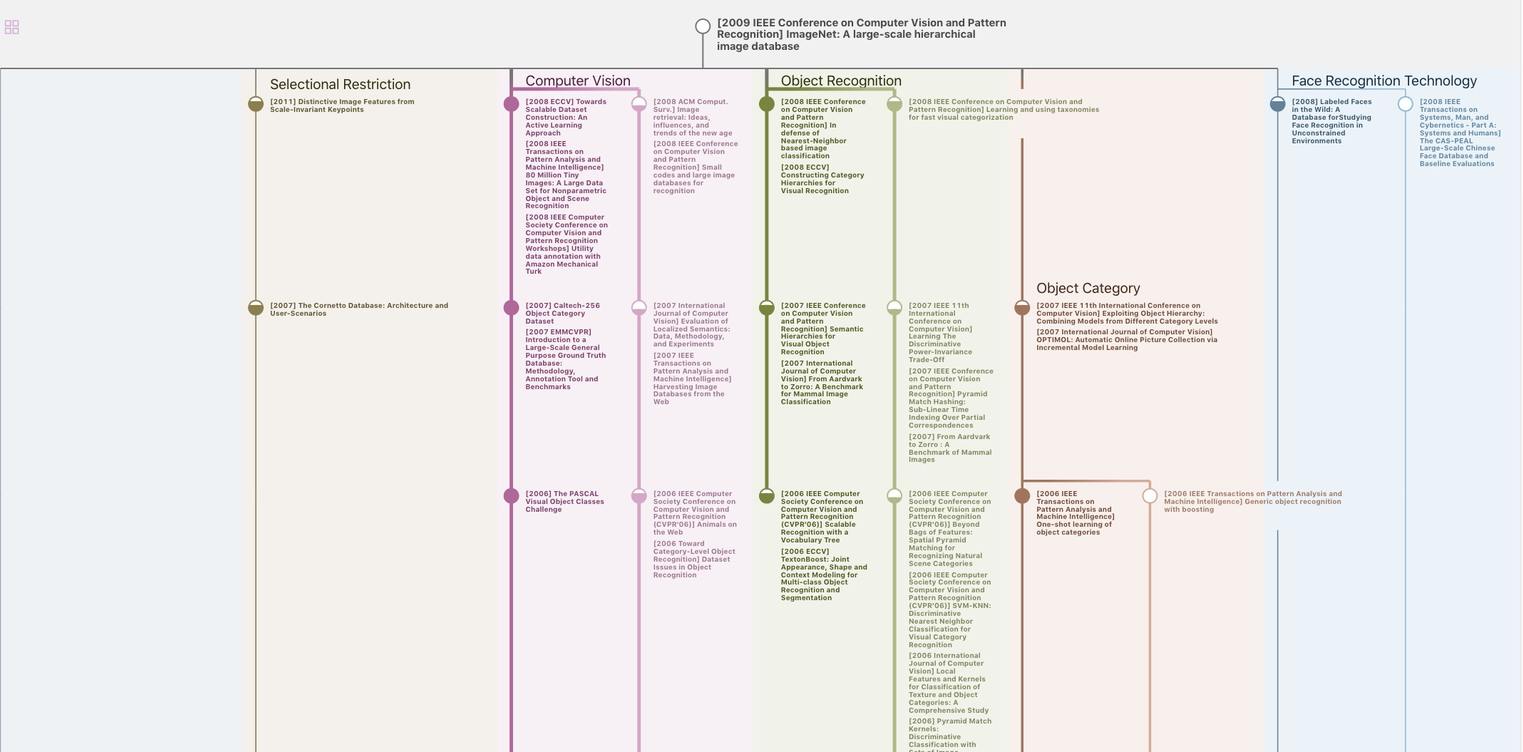
生成溯源树,研究论文发展脉络
Chat Paper
正在生成论文摘要