SolPredictor: Predicting Solubility with Residual Gated Graph Neural Network
INTERNATIONAL JOURNAL OF MOLECULAR SCIENCES(2024)
摘要
Computational methods play a pivotal role in the pursuit of efficient drug discovery, enabling the rapid assessment of compound properties before costly and time-consuming laboratory experiments. With the advent of technology and large data availability, machine and deep learning methods have proven efficient in predicting molecular solubility. High-precision in silico solubility prediction has revolutionized drug development by enhancing formulation design, guiding lead optimization, and predicting pharmacokinetic parameters. These benefits result in considerable cost and time savings, resulting in a more efficient and shortened drug development process. The proposed SolPredictor is designed with the aim of developing a computational model for solubility prediction. The model is based on residual graph neural network convolution (RGNN). The RGNNs were designed to capture long-range dependencies in graph-structured data. Residual connections enable information to be utilized over various layers, allowing the model to capture and preserve essential features and patterns scattered throughout the network. The two largest datasets available to date are compiled, and the model uses a simplified molecular-input line-entry system (SMILES) representation. SolPredictor uses the ten-fold split cross-validation Pearson correlation coefficient R2 0.79 +/- 0.02 and root mean square error (RMSE) 1.03 +/- 0.04. The proposed model was evaluated using five independent datasets. Error analysis, hyperparameter optimization analysis, and model explainability were used to determine the molecular features that were most valuable for prediction.
更多查看译文
关键词
molecular solubility,drug discovery,ADMET,artificial intelligence,regression,graph neural network,residual gated graph neural network,simplified molecular-input line-entry system
AI 理解论文
溯源树
样例
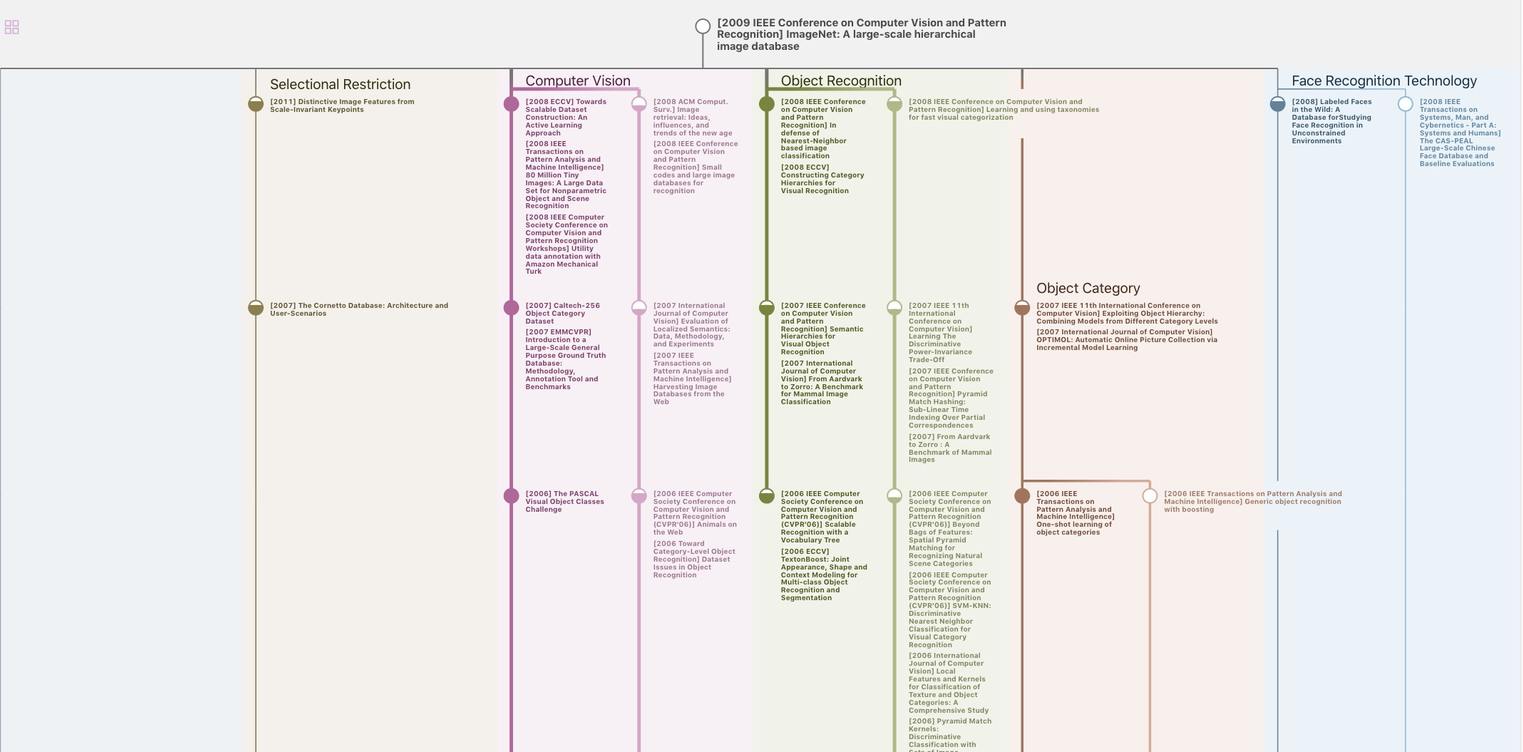
生成溯源树,研究论文发展脉络
Chat Paper
正在生成论文摘要