SRT-Net: Scattering Region Topology Network for Oriented Ship Detection in Large-Scale SAR Images.
IEEE Transactions on Geoscience and Remote Sensing(2024)
摘要
Synthetic aperture radar (SAR) ship detection plays an important role in the field of maritime security. However, certain unique imaging properties make it challenging to extract the shape features of ships, such as speckle noise and strong scattering interference from irrelevant objects. These factors result in inaccurate ship localization and obvious false alarms under complex large-scale inshore scenes. To address this issue, we propose the scattering region topology network (SRT-Net), which can dynamically capture the comprehensive global context and enhance the ship saliency. This is achieved through two key modules, namely the scattering region topological structure pyramid (SRTP) and the ship saliency enhancement (SSE) module. The former provides richer semantic information to distinguish the object from the background, while the latter offers an extra pixel-level classification task to guide accurate bounding box regression. Thanks to the guidance of richer information, the proposed method can achieve fewer false alarms and enhance location accuracy. Additionally, we introduce a scale feature adaptive (SFA) loss to balance the attention to ships with various scales, which improves the robustness of multiscale ship detection. The proposed method achieves state-of-the-art performance under complex inshore scenes, and its effectiveness is verified by experiments on a large-scale SAR ship detection dataset (LSSDD) and a public SAR ship detection dataset (SSDD+).
更多查看译文
关键词
Deep learning,graph convolution network,oriented ship detection,strong scattering interference,synthetic aperture radar (SAR),topological structure
AI 理解论文
溯源树
样例
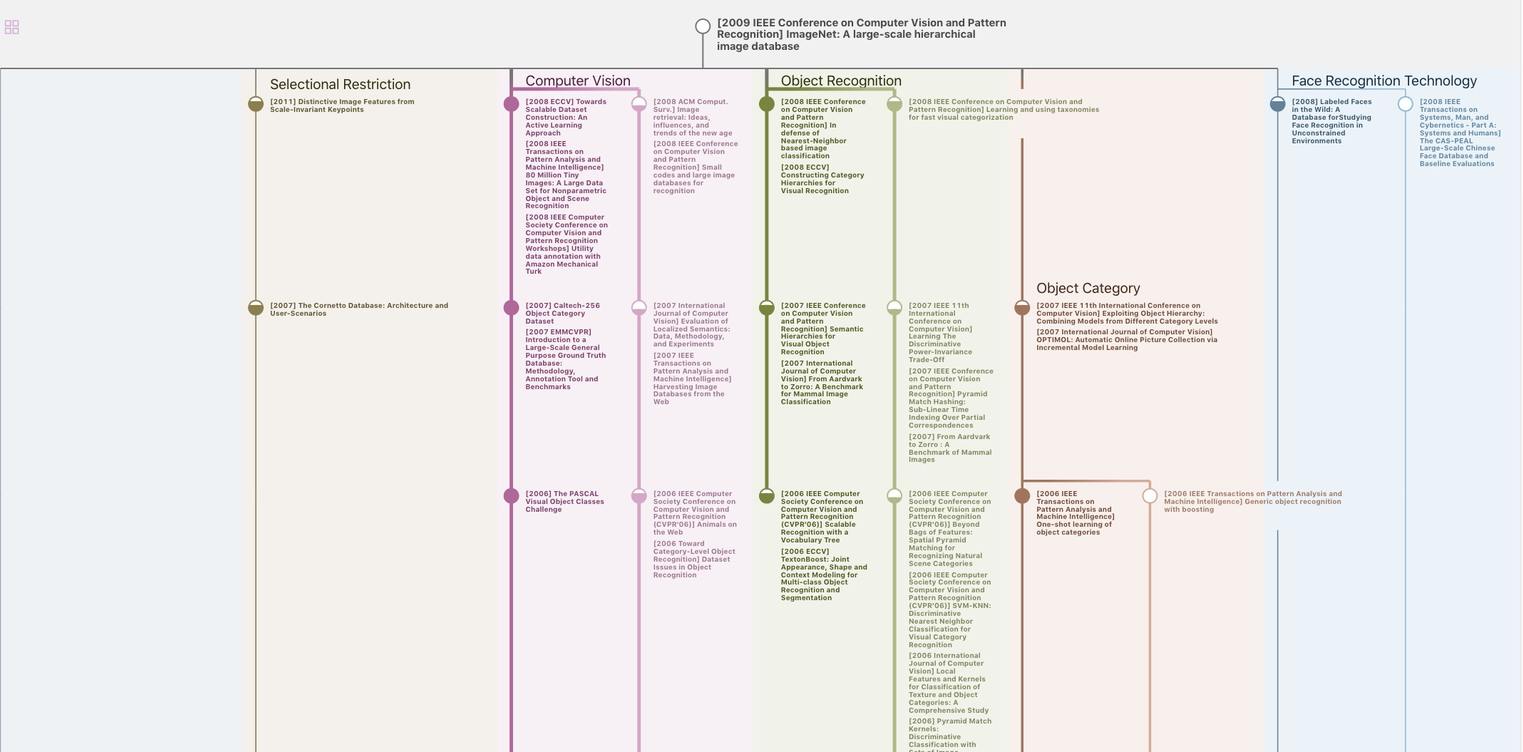
生成溯源树,研究论文发展脉络
Chat Paper
正在生成论文摘要