Thickness Estimation of Biological Tissue Sections from Structural Deformation
2023 6th International Conference on Information Communication and Signal Processing (ICICSP)(2023)
摘要
Microscopic neural circuit reconstruction is a critical approach to understanding the mechanism of brain function. The mainstream method for acquiring microscopic brain images involves volume electron microscopy (EM) imaging based on serial sections. The thickness of these serial sections, representing the size of voxels in the Z-axis, plays a crucial role in the reconstruction process, as accurate thickness information generates high-fidelity reconstructions. However, the accuracy of thickness estimation using pixel-based measurements remains limited. In this paper, we propose a novel two-stage thickness estimation process based on tissue structural deformation. In the first stage, a Convolutional Neural Network (CNN) captures the deformation between sections. In the second stage, a Multi-Layer Perceptron (MLP) is utilized to estimate the thickness based on the extracted deformation field. To evaluate our approach, we constructed a dataset of electron microscopy images with different thickness labels based on public Focused Ion Beam Scanning Electron Microscopy (FIB-SEM) images and conducted comparative experiments based on this dataset. We achieved a mean percentage error of 0.85% and a mean absolute percentage error of 8.03% in the testing dataset.
更多查看译文
关键词
section thickness estimation,biological tissue sections,structural deformation field,electron microscopy imaging
AI 理解论文
溯源树
样例
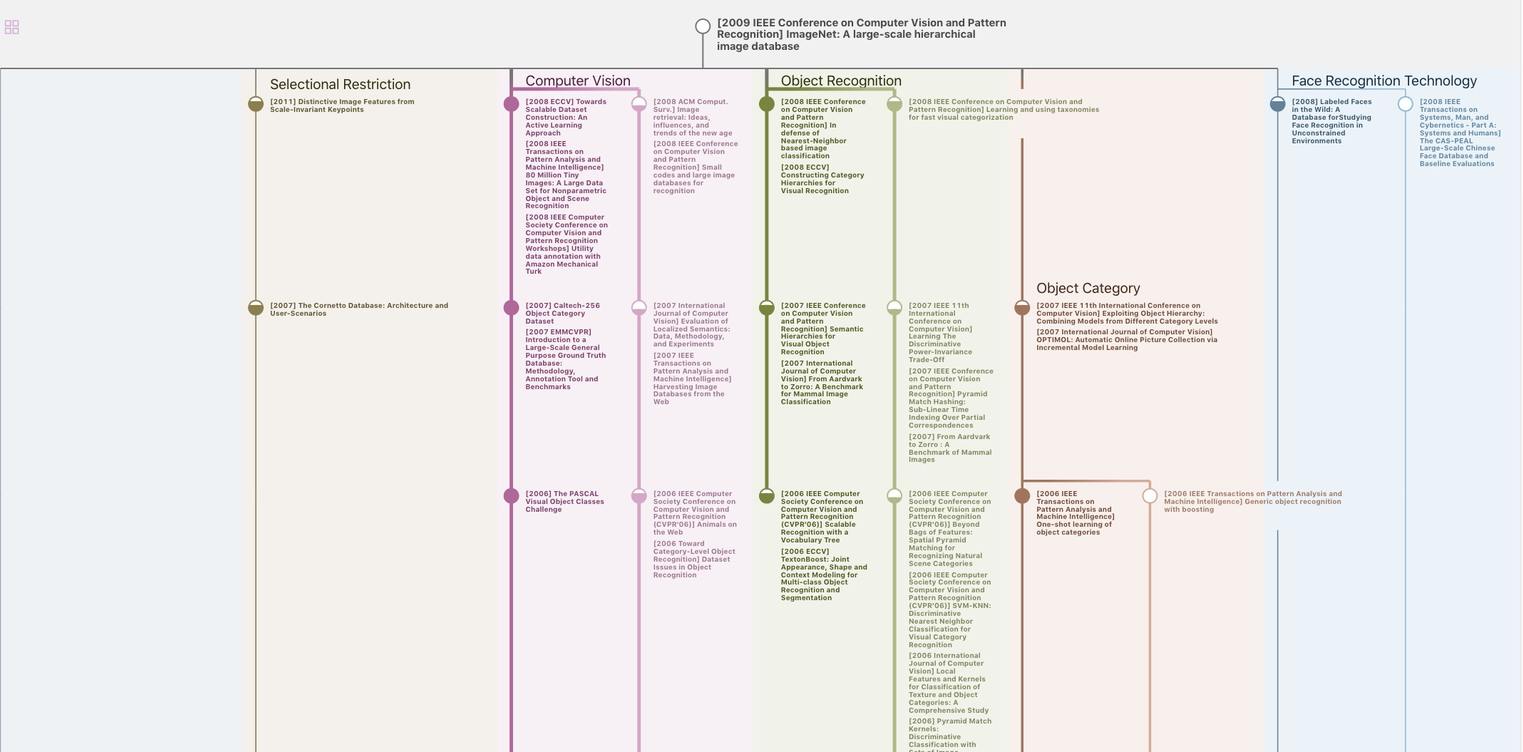
生成溯源树,研究论文发展脉络
Chat Paper
正在生成论文摘要