Personalized Federated Aggregation Algorithm based on Local Attention Mechanism.
2023 IEEE 14th International Symposium on Parallel Architectures, Algorithms and Programming (PAAP)(2023)
摘要
Federated learning is a distributed learning approach that balances data privacy and collaborative learning. To address the impact of non-IID (non-Independently and Identically Distributed) data on the performance of the global model, this study delved into personalized federated learning. First, we introduced a local network with a self-attention mechanism, enhancing the personalization of local models. Next, we proposed a weighted average aggregation method, assigning weights based on the distance of the client model parameters and variations in contributions, mitigating the adverse effects of uneven data distribution. Using a dataset constructed from non-IID data partitions simulated via the Dirichlet distribution, we experimented, integrating the self-attention mechanism of the local model and the personalized aggregation strategy. Experimental results indicate that this approach significantly improves the test accuracy of the MNIST and SVHN datasets while reducing generalization error. In addition, it also reduces the oscillation during training, showing good robustness. In conclusion, this study offers fresh insights into handling non-IID data in federated learning and empirically validates the method’s effectiveness.
更多查看译文
关键词
Federated learning,Personalized aggregation,Self-attention mechanism,Non-IID Data
AI 理解论文
溯源树
样例
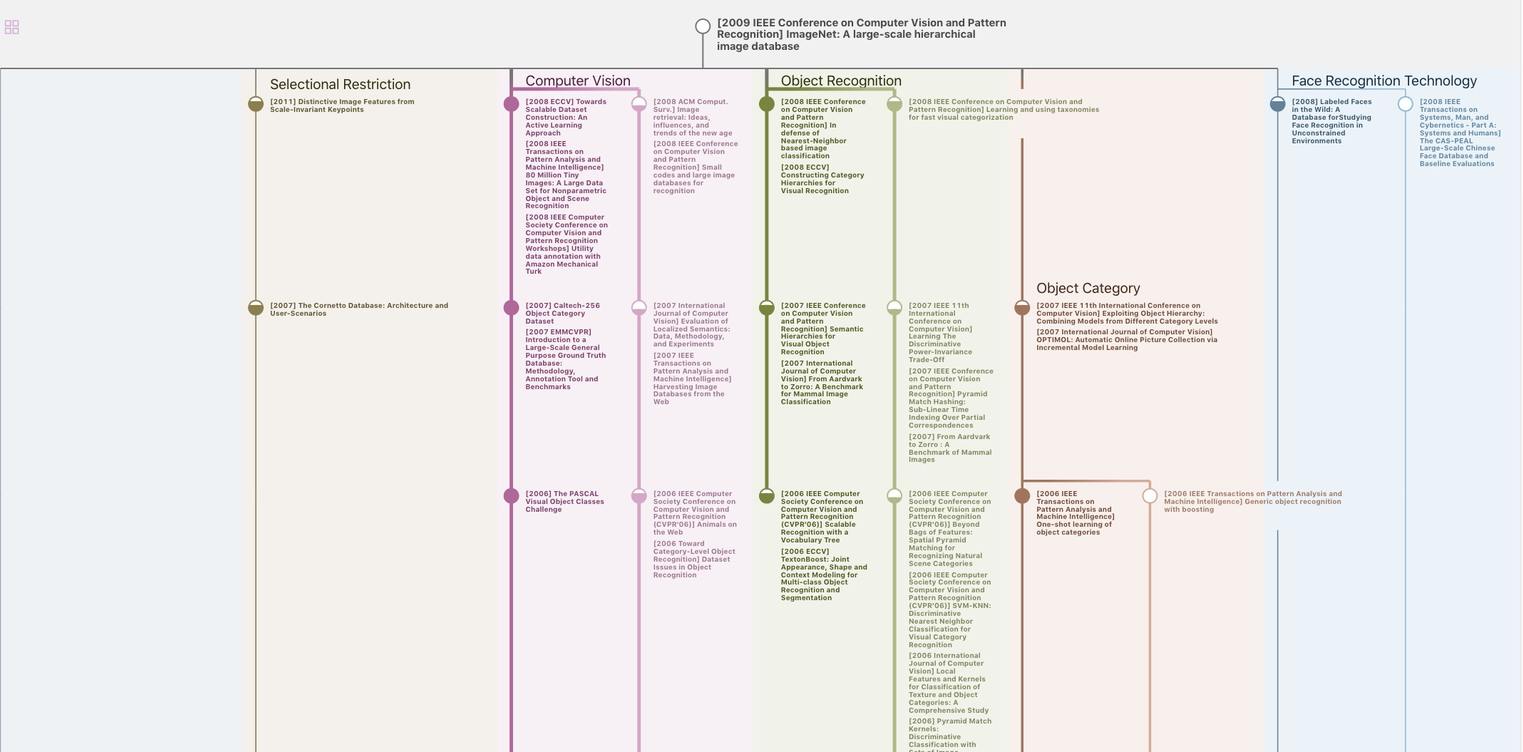
生成溯源树,研究论文发展脉络
Chat Paper
正在生成论文摘要