Efficiently Mining Frequent Representative Motifs in Large Collections of Time Series.
2023 IEEE International Conference on Big Data (BigData)(2023)
摘要
The discovery of repeated structures in time series, known as motifs, is an important data mining task. Various techniques exist to mine motifs in either a database of time series or within one or two individual time series, either for a user-defined motif length or a range of lengths. However, mining frequent motifs of variable length in large time series databases remains an unsolved task that is computationally expensive. We propose FRM-Miner, an efficient algorithm for discovering more informative patterns in time series data, i.e., motifs of different length that are non-overlapping, occur frequently and where the euclidean distance between the motif and its various occurrences is minimal. Unlike current state-of-the-art approaches, FRM-Miner can efficiently find variable motif lengths in large time series databases. Through extensive experimentation, we show desirable properties of FRM-Miner, such as robustness to noise and expressive power, thereby discovering motifs that remain undetected using state-of-the-art methods. Additionally, our method is highly scalable, taking only 2.95 hours to discover informative sets of motifs on all 128 time series data sets of the UCR Time Series Archive, where related state-of-the-art algorithms such as Ostinato require several days.
更多查看译文
AI 理解论文
溯源树
样例
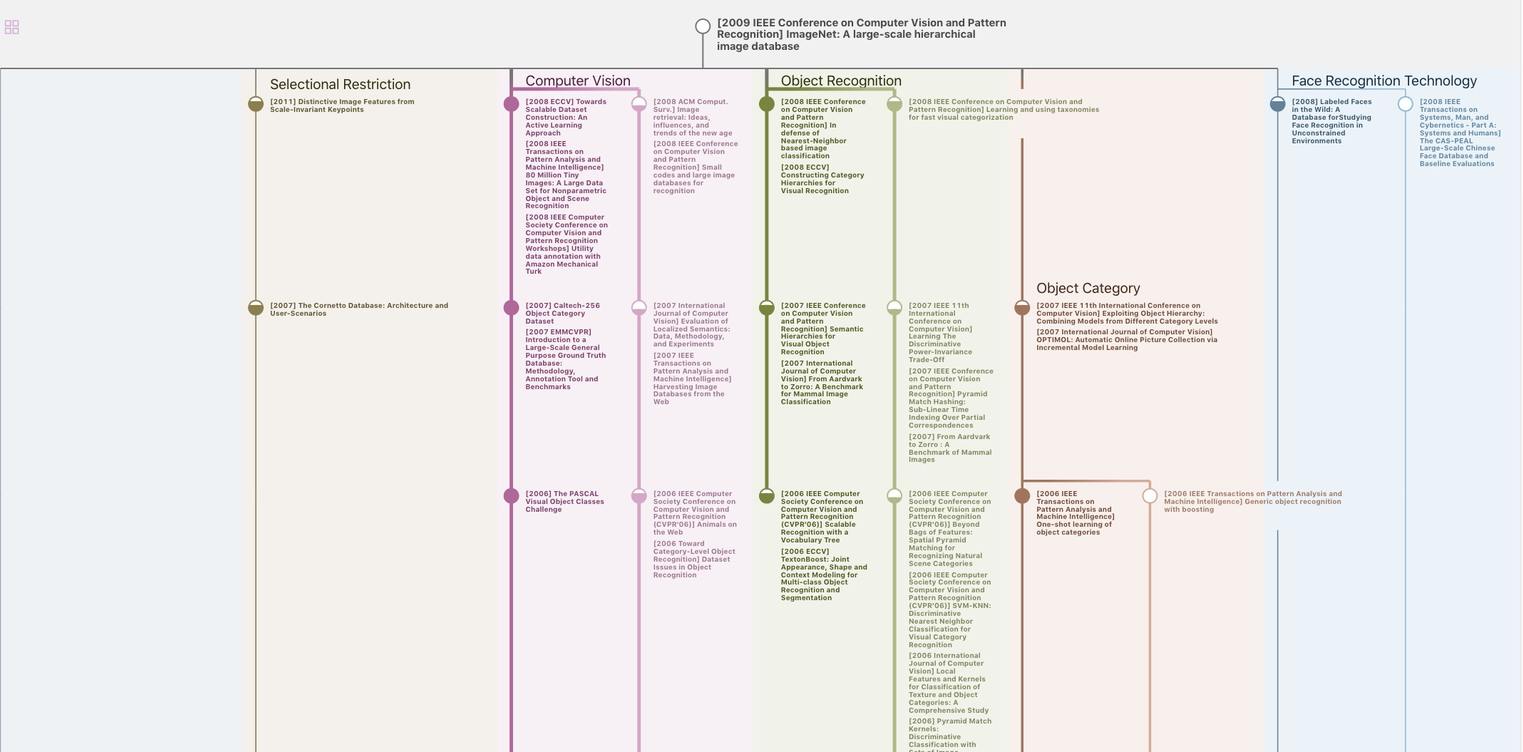
生成溯源树,研究论文发展脉络
Chat Paper
正在生成论文摘要