Detecting Semantic Errors in Tables using Textual Evidence.
2023 IEEE International Conference on Big Data (BigData)(2023)
摘要
Tables can contain various types of errors, including both syntactic and semantic errors. Semantic errors relate to the meaning of the data and can be detrimental for downstream applications. The existing approaches for semantic error detection use structured knowledge sources such as Wikidata and DBpedia, but the coverage of such sources is quite limited. There is much more information available in free text to validate the contents of tables. In this paper, we present a novel semantic-error-detection approach that exploits open-domain textual data to verify the semantic correctness of tables. Our approach leverages contrastive learning, table linearization, and pre-trained language models to implement the error detection process. We implement our approach in a system called SEED and show in the evaluation that it significantly outperforms the other competing approaches.
更多查看译文
关键词
Semantic error detection,contrastive learning,language models,textual evidence
AI 理解论文
溯源树
样例
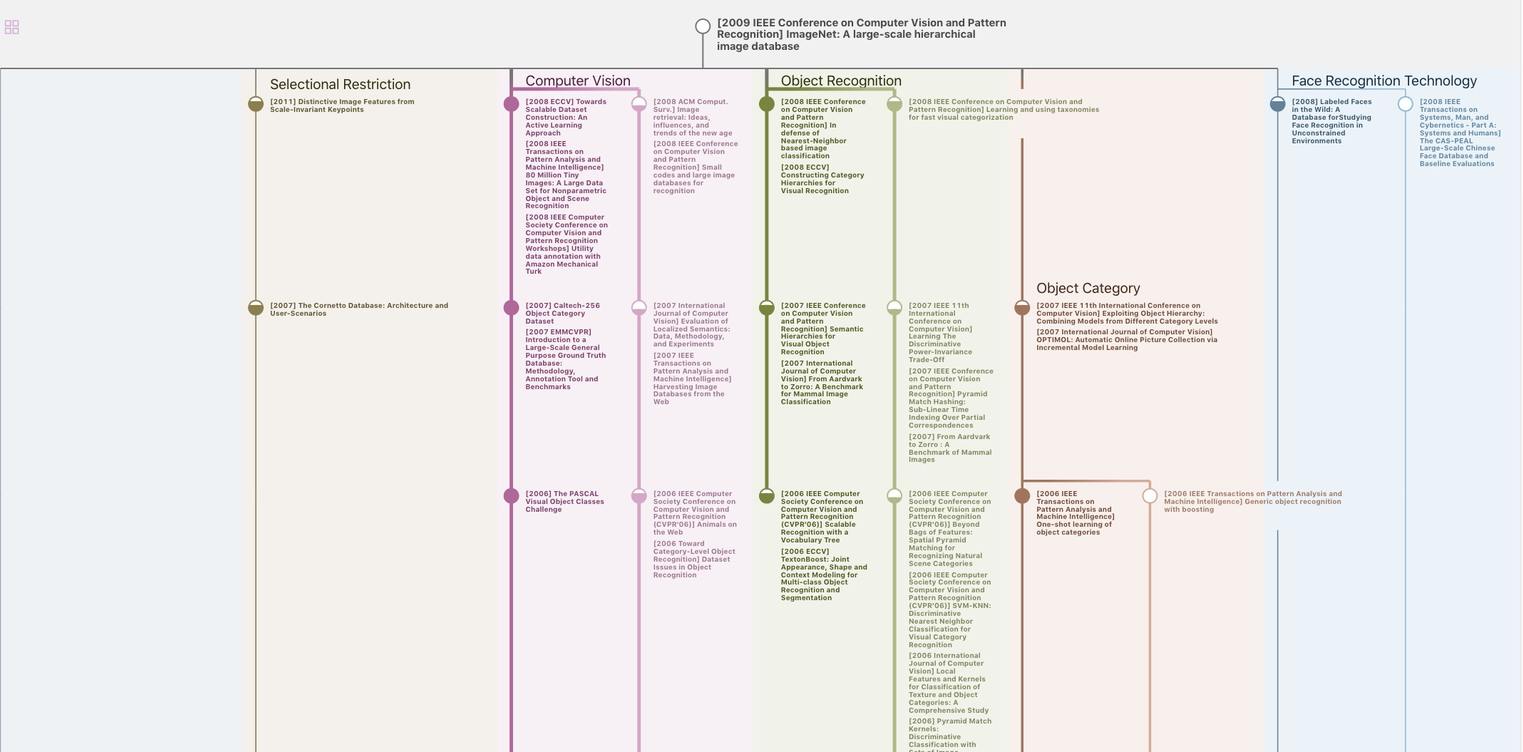
生成溯源树,研究论文发展脉络
Chat Paper
正在生成论文摘要