Unvisited Out-Of-Town POI Recommendation with Simultaneous Learning of Multiple Regions.
2023 IEEE International Conference on Big Data (BigData)(2023)
摘要
In recent years, Point Of Interest (POI) recommendations have been actively studied because of the widespread use of location-based social network services. Out-Of-Town POI recommendation methods recommend POIs outside a user’s residence, such as travel and business trip destinations. Most existing methods require a certain number of past visit sequences at destination regions or can be used only between two specific unidirectional regions. In this study, we propose an Unvisited Out-Of-Town POI Recommendation framework (UOPR), which can recommend POIs for Out-Of-Town regions that have not yet been visited by users. In addition, UOPR can learn users’ visit patterns for multiple regions simultaneously. UOPR takes into account the fact that user’s Out-Of-Town visiting tendencies vary depending on the geographical distance from their residences. It therefore adopts an approach that calculates two scores, one determined by user’s Home-Town visiting tendencies and the other by their Out-Of-Town visiting tendencies, and uses them differently. Furthermore, we propose a mask-input learning and user-embedding creation method that enables learning with enhanced interaction between POI embeddings in an Out-Of-Town POI recommendation environment, where the data are often sparse. UOPR achieves higher recommendation accuracy than existing methods on a dataset collected on a real-world service.
更多查看译文
AI 理解论文
溯源树
样例
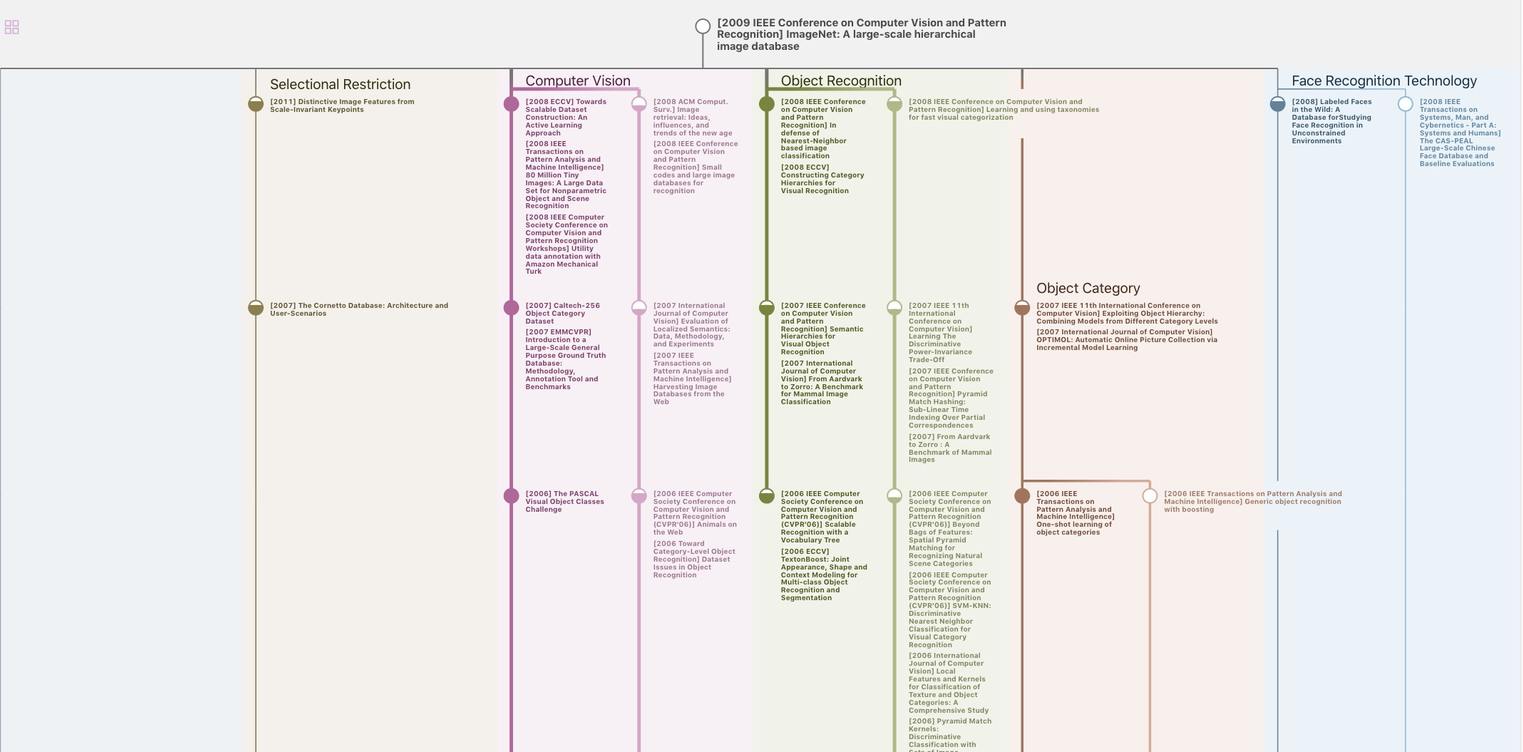
生成溯源树,研究论文发展脉络
Chat Paper
正在生成论文摘要