Meta Reinforcement Learning for Strategic IoT Deployments Coverage in Disaster-Response UAV Swarms
GLOBECOM 2023 - 2023 IEEE Global Communications Conference(2024)
摘要
In the past decade, Unmanned Aerial Vehicles (UAVs) have grabbed the
attention of researchers in academia and industry for their potential use in
critical emergency applications, such as providing wireless services to ground
users and collecting data from areas affected by disasters, due to their
advantages in terms of maneuverability and movement flexibility. The UAVs'
limited resources, energy budget, and strict mission completion time have posed
challenges in adopting UAVs for these applications. Our system model considers
a UAV swarm that navigates an area collecting data from ground IoT devices
focusing on providing better service for strategic locations and allowing UAVs
to join and leave the swarm (e.g., for recharging) in a dynamic way. In this
work, we introduce an optimization model with the aim of minimizing the total
energy consumption and provide the optimal path planning of UAVs under the
constraints of minimum completion time and transmit power. The formulated
optimization is NP-hard making it not applicable for real-time decision making.
Therefore, we introduce a light-weight meta-reinforcement learning solution
that can also cope with sudden changes in the environment through fast
convergence. We conduct extensive simulations and compare our approach to three
state-of-the-art learning models. Our simulation results prove that our
introduced approach is better than the three state-of-the-art algorithms in
providing coverage to strategic locations with fast convergence.
更多查看译文
关键词
Optimization,QoS,UAVs positions,energy consumption,meta-reinforcement learning,reinforcement learning,UAVs,strategic locations
AI 理解论文
溯源树
样例
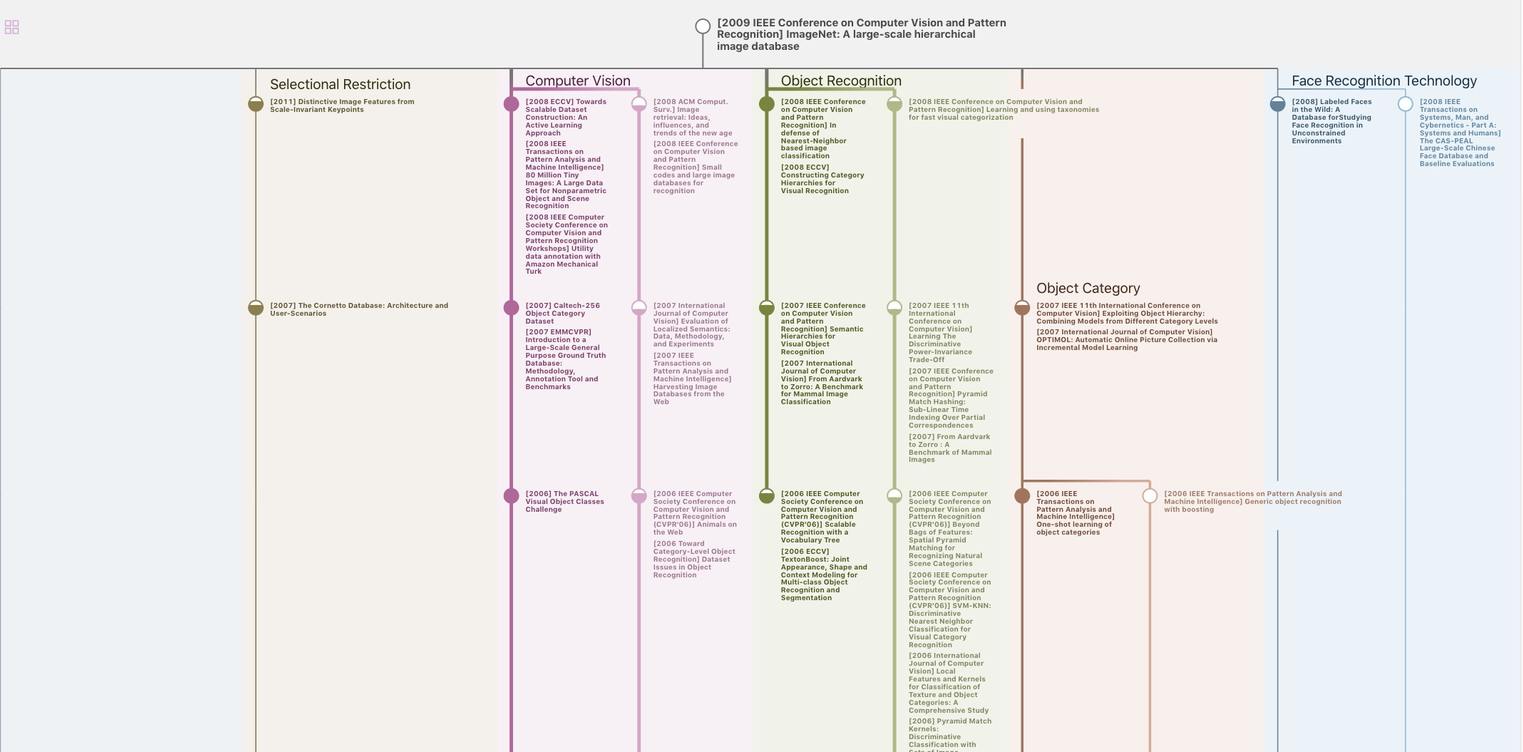
生成溯源树,研究论文发展脉络
Chat Paper
正在生成论文摘要