Empirical martingale projections via the adapted Wasserstein distance
arxiv(2024)
摘要
Given a collection of multidimensional pairs {(X_i,Y_i):1 ≤ i≤ n},
we study the problem of projecting the associated suitably smoothed empirical
measure onto the space of martingale couplings (i.e. distributions satisfying
𝔼[Y|X]=X) using the adapted Wasserstein distance. We call the
resulting distance the smoothed empirical martingale projection distance
(SE-MPD), for which we obtain an explicit characterization. We also show that
the space of martingale couplings remains invariant under the smoothing
operation. We study the asymptotic limit of the SE-MPD, which converges at a
parametric rate as the sample size increases if the pairs are either i.i.d. or
satisfy appropriate mixing assumptions. Additional finite-sample results are
also investigated. Using these results, we introduce a novel consistent
martingale coupling hypothesis test, which we apply to test the existence of
arbitrage opportunities in recently introduced neural network-based generative
models for asset pricing calibration.
更多查看译文
AI 理解论文
溯源树
样例
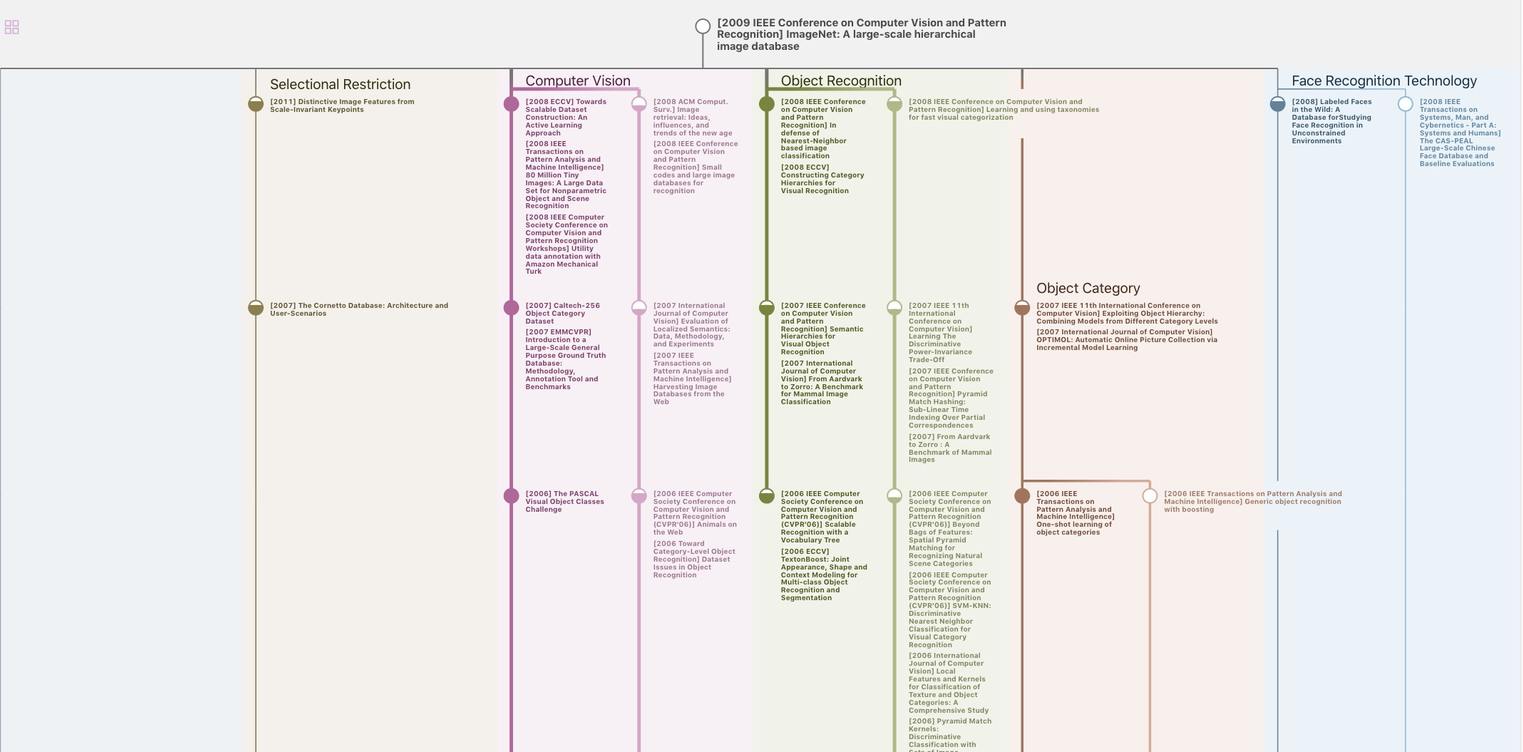
生成溯源树,研究论文发展脉络
Chat Paper
正在生成论文摘要