Machine Learning Based Prediction of Polaron-Vacancy Patterns on the TiO_2(110) Surface
arxiv(2024)
摘要
The multifaceted physics of oxides is shaped by their composition and the
presence of defects, which are often accompanied by the formation of polarons.
The simultaneous presence of polarons and defects, and their complex
interactions, pose challenges for first-principles simulations and experimental
techniques. In this study, we leverage machine learning and a first-principles
database to analyze the distribution of surface oxygen vacancies (V_ O)
and induced small polarons on rutile TiO_2(110), effectively disentangling
the interactions between polarons and defects. By combining neural-network
supervised learning and simulated annealing, we elucidate the inhomogeneous
V_ O distribution observed in scanning probe microscopy (SPM). Our
innovative approach allows us to understand and predict defective surface
patterns at previously inaccessible length scales, identifying the specific
role of individual types of defects. Specifically, surface-polaron-stabilizing
V_ O-configurations are identified, which could have consequences for
surface reactivity.
更多查看译文
AI 理解论文
溯源树
样例
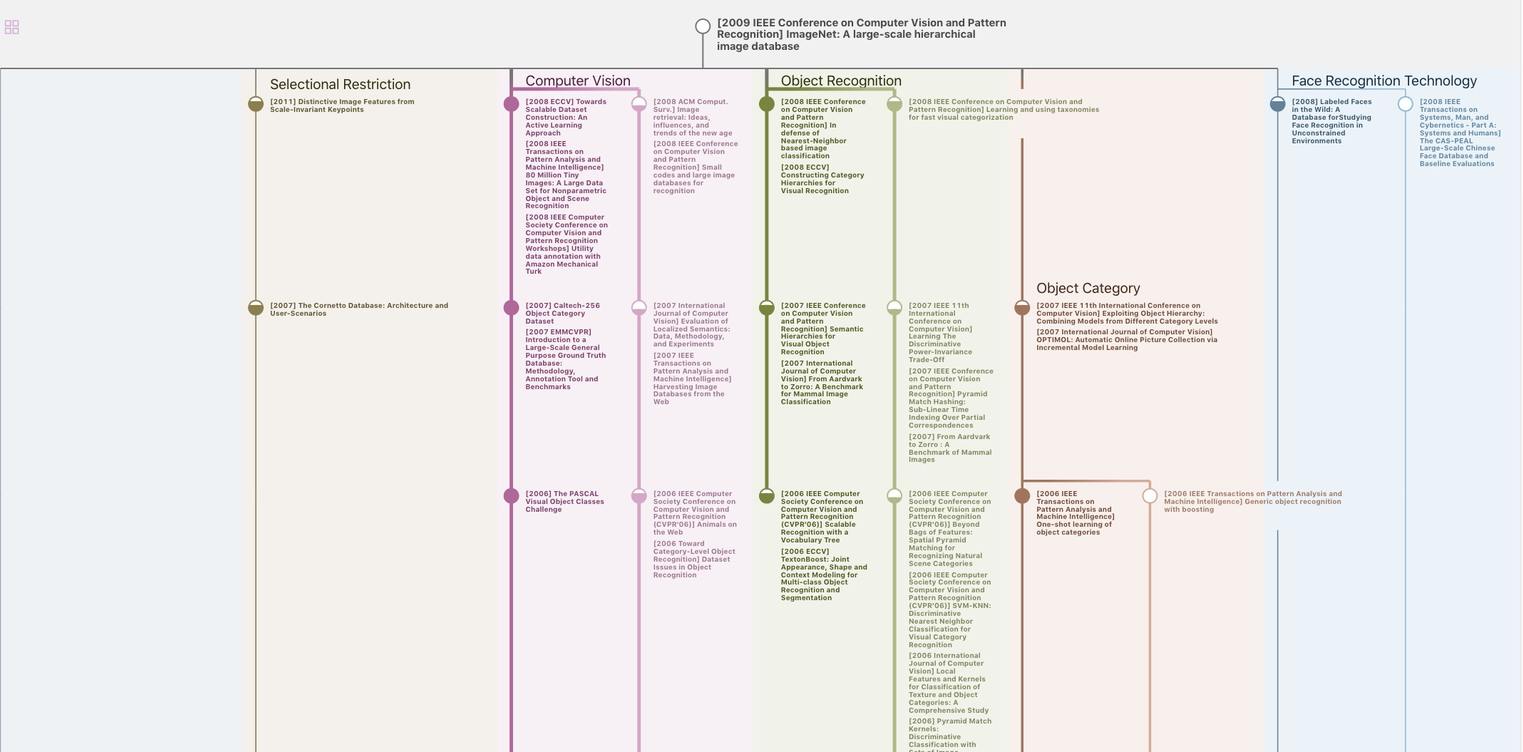
生成溯源树,研究论文发展脉络
Chat Paper
正在生成论文摘要