A Transformer Encoder and Convolutional Neural Network Combined Method for Classification of Error-related Potentials.
2023 IEEE Biomedical Circuits and Systems Conference (BioCAS)(2023)
摘要
Background and Objectives. As an inherent feedback mechanism in humans, Error-related Potentials (ErrPs) signals have received increasing attention for their potential to improve the accuracy and efficiency of Brain-computer interface (BCI) systems. However, the successful detection of ErrPs signals in a single trial is a great challenge due to the low signal-to-noise ratio (SNR) and the high individual differences. In this study, we propose a novel method that combines convolutional layers and transformer encoder modules to classify ErrPs from electroencephalogram (EEG) signals. Methods. The raw data from all electrode channels were chosen as the inputs of the proposed method and two different dataset partition ways, which include within-session and cross-subject, were used to test the proposed model. The dataset used for evaluation is the ‘Monitoring error-related potentials’ dataset of the BNCI Horizon 2020. Results. For within-session classification, a mean accuracy of 88.50%, an AUROC of 0.95, and a F1-score of 0.82 using stratified 10-fold cross-validation were achieved. For cross-subject classification, the average accuracy, AUROC, and Fl-score have reached 82.89%, 0.90, and 0.71 respectively. The results obtained outperformed the state-of-the-art methods. Conclusions. The results showed the ability of transformer-based deep learning (DL) method in detecting ErrPs signals from low SNR EEG data and shed light on improving the performance of BCI systems.
更多查看译文
关键词
Error-related potential,brain-computer interface,transformer encoder,convolutional neural network
AI 理解论文
溯源树
样例
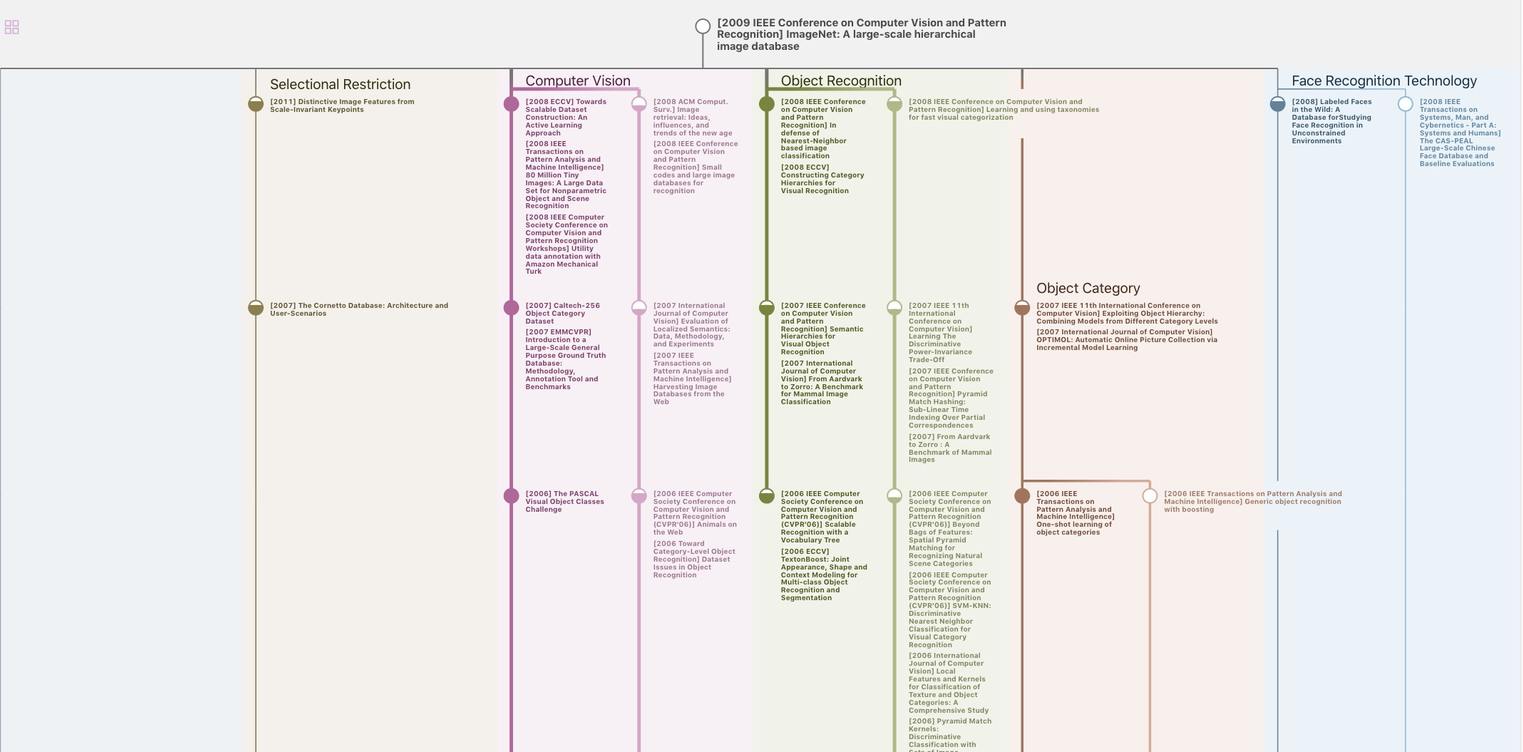
生成溯源树,研究论文发展脉络
Chat Paper
正在生成论文摘要