Learning Adaptive Horizon Maps Based on Error Forecast for Model Predictive Control
2023 62ND IEEE CONFERENCE ON DECISION AND CONTROL, CDC(2023)
摘要
We present a model predictive control framework that uses varying prediction horizons according to the current forecasted uncertainties and estimated distance of the terminal state from its desired state. Our results suggest that the space of such optimal horizons, which we call horizon maps, is well structured for linear systems, meaning that it can be easily learned using tools from machine learning. Our approach is well suited for real-time control and can scale to higher dimensional systems. We also perform an analysis on the required quality of the datasets used to learn the horizon maps and conclude with results of this framework using an externally-driven, constrained linear quadratic regulator problem.
更多查看译文
AI 理解论文
溯源树
样例
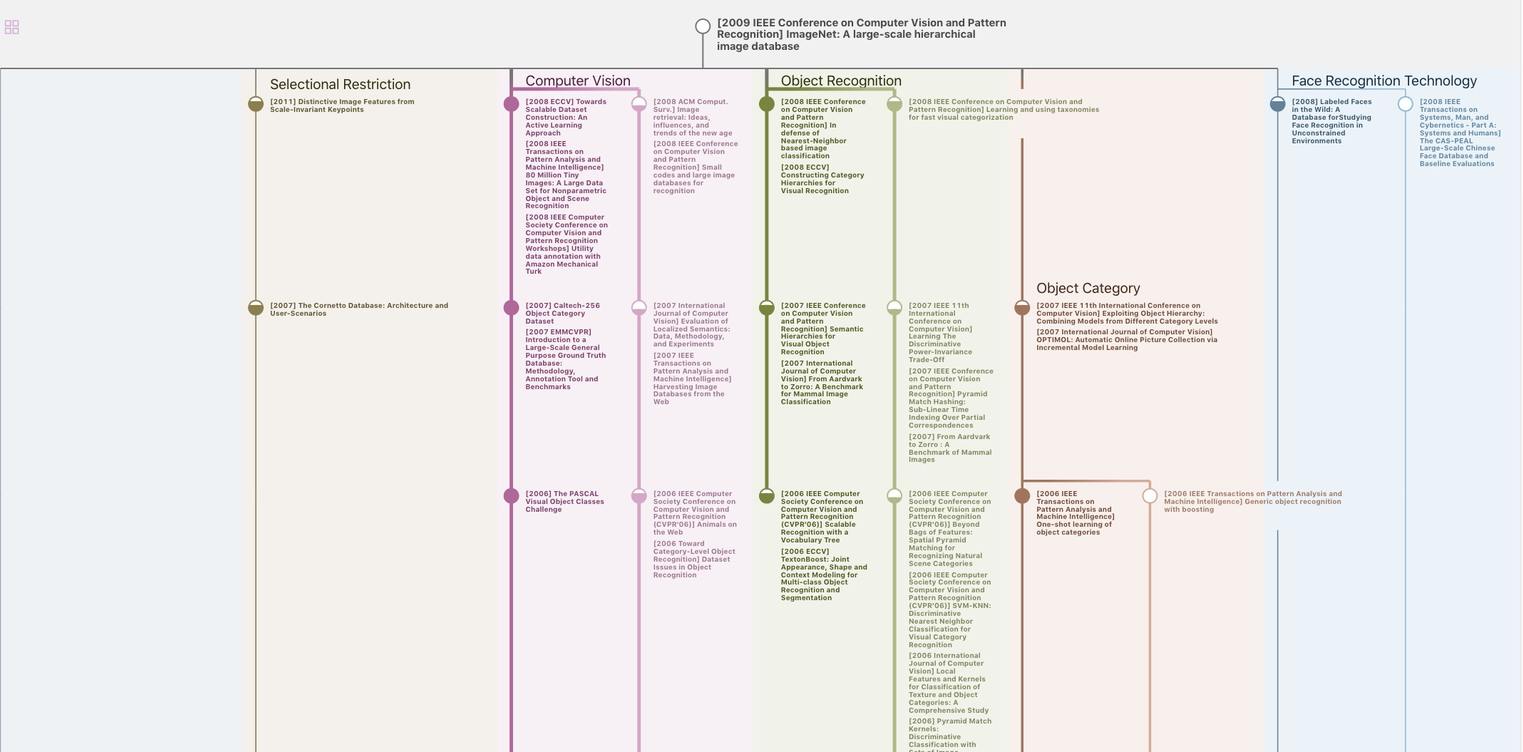
生成溯源树,研究论文发展脉络
Chat Paper
正在生成论文摘要