A Family of Hyper-parameter Estimators for Regularized Linear System Identification
2023 62ND IEEE CONFERENCE ON DECISION AND CONTROL, CDC(2023)
摘要
Hyper-parameter estimation is one of the fundamental issues for kernel-based regularized system identification methods. Empirical Bayes (EB) estimator and Stein's unbiased risk estimator (SURE) are two popular hyper-parameter estimators, but they both have advantages and disadvantages. Specifically, EB is not asymptotically optimal in the mean squared error (MSE) sense but SURE is, while SURE is more sensitive to ill-conditioned regression matrix but EB is more robust. In this paper, to find a better estimator by combining their strength and mitigating their weakness, we propose a family of hyper-parameter estimators by linking EB and SURE estimators together through an index. The finite sample and asymptotic properties of this family of estimators have been established. The Monte Carlo simulation results show that there does exist a 'middle' hyper-parameter estimator in this family that is superior to the EB and SURE.
更多查看译文
关键词
Linear system identification,Kernel-based regularization method,hyper-parameter estimator,empirical Bayes estimator,Stein's unbiased risk estimator
AI 理解论文
溯源树
样例
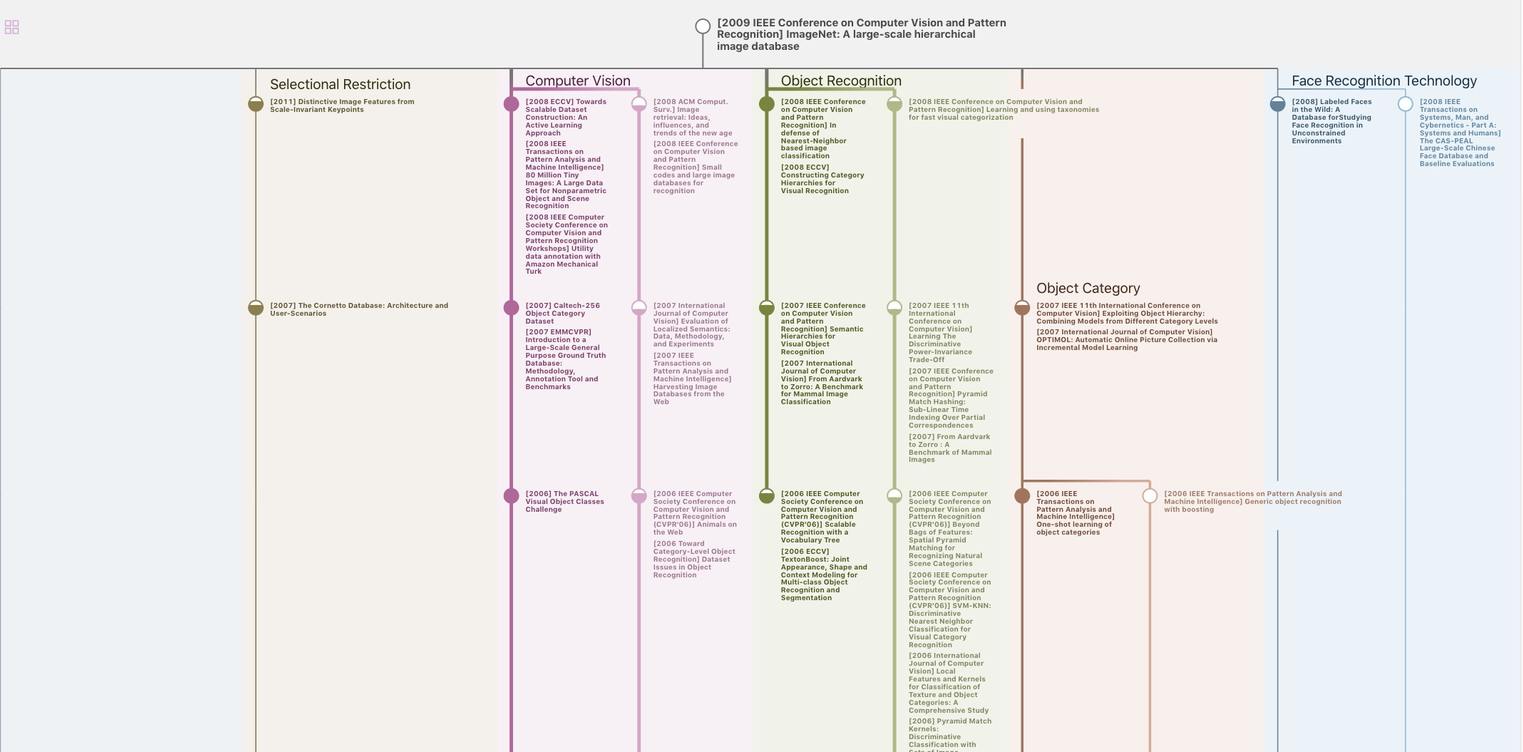
生成溯源树,研究论文发展脉络
Chat Paper
正在生成论文摘要