Tuning Convergence Rate via Non-Bayesian Social Learning: A Trade-Off between Internal Belief and External Information
2023 62ND IEEE CONFERENCE ON DECISION AND CONTROL, CDC(2023)
摘要
Social learning strategies have been recently developed for multi-agents to learn progressively an underlying state of nature by information communications and evolutions. Existing works define algorithms mainly by swapping the Bayesian update and belief aggregation steps and/or discovering diverse underlying network structures. Inspired by the diversity of agents when they are exposed to new information, this work designs a non-Bayesian learning strategy, named as Parametric Social Learning, by introducing an agent stubbornness parameter to trade-off the significance between its internal belief and external information. This strategy thus allows for tuning the convergence rate by adjusting the introduced parameter, which is consistent highly with the sociological intuition. Theoretical analyses and numerical examples are provided to illustrate several sociological insights. Our work therefore has appealing potential in practical tasks such as dispersed information aggregation and distributed parameter estimation.
更多查看译文
AI 理解论文
溯源树
样例
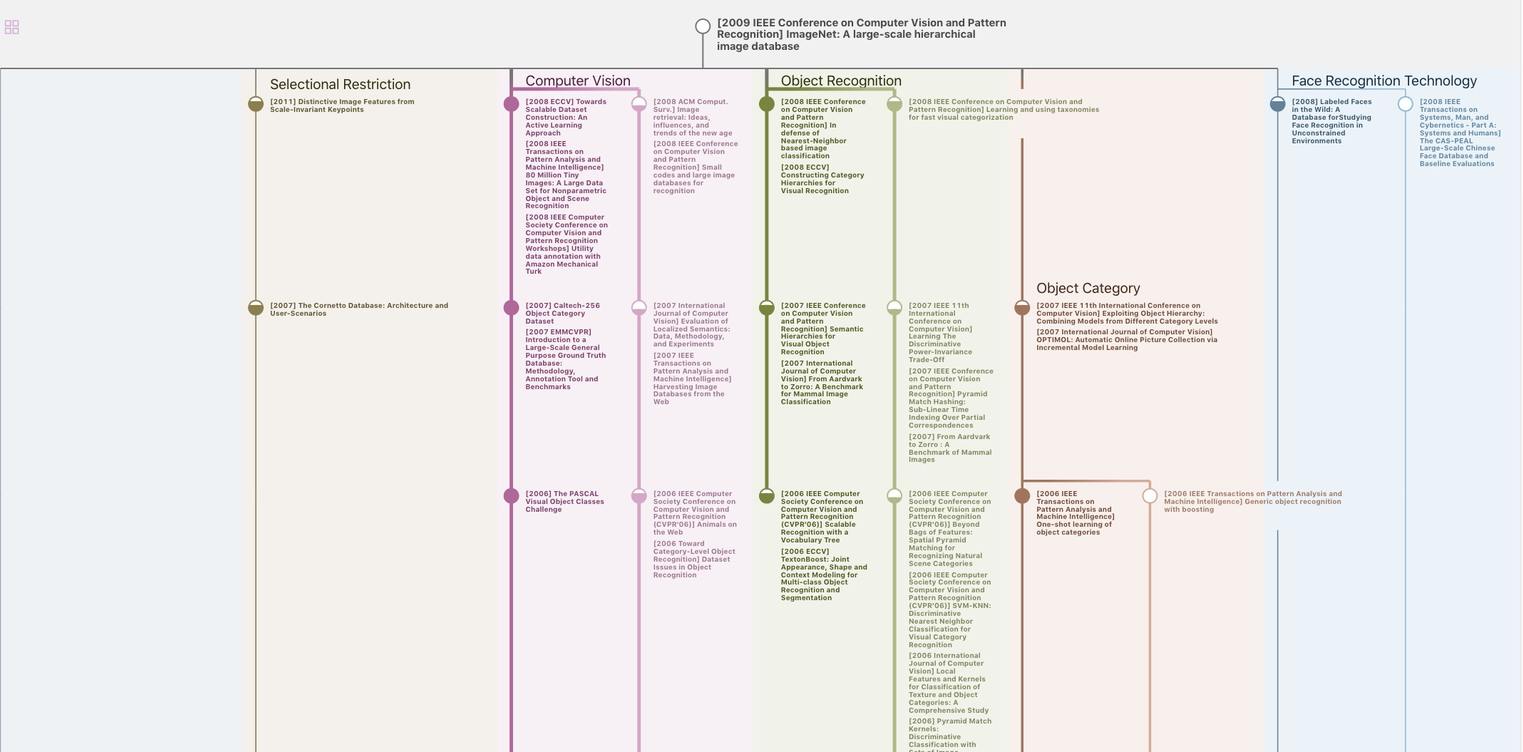
生成溯源树,研究论文发展脉络
Chat Paper
正在生成论文摘要