Dynamics and Perturbations of Overparameterized Linear Neural Networks
2023 62ND IEEE CONFERENCE ON DECISION AND CONTROL, CDC(2023)
摘要
Recent research in neural networks and machine learning suggests that using many more parameters than strictly required by the initial complexity of a regression problem can result in more accurate or faster-converging models - contrary to classical statistical belief. This phenomenon, sometimes referred to as "benign overfitting", raises questions regarding in what other ways might overparameterization affect the properties of a learning problem. In this work, we investigate the effects of overfitting on the robustness of gradient-descent training when subject to uncertainty on the gradient estimation, which arises naturally if the gradient is estimated from noisy data or directly measured. Our object of study is a linear neural network with a single, arbitrarily wide, hidden layer and an arbitrary number of inputs and outputs, which can be equivalently written as an overparameterized matrix factorization problem. In this paper we solve the problem for the case where the input and output of our neural network are one-dimensional, deriving sufficient conditions for the robustness of our system based on necessary and sufficient conditions for convergence in the undisturbed case. We then show that the general overparameterized formulation introduces a set of spurious equilibria that lay outside the set where the loss function is minimized, and discuss directions of future work that might extend our current results for more general settings.
更多查看译文
AI 理解论文
溯源树
样例
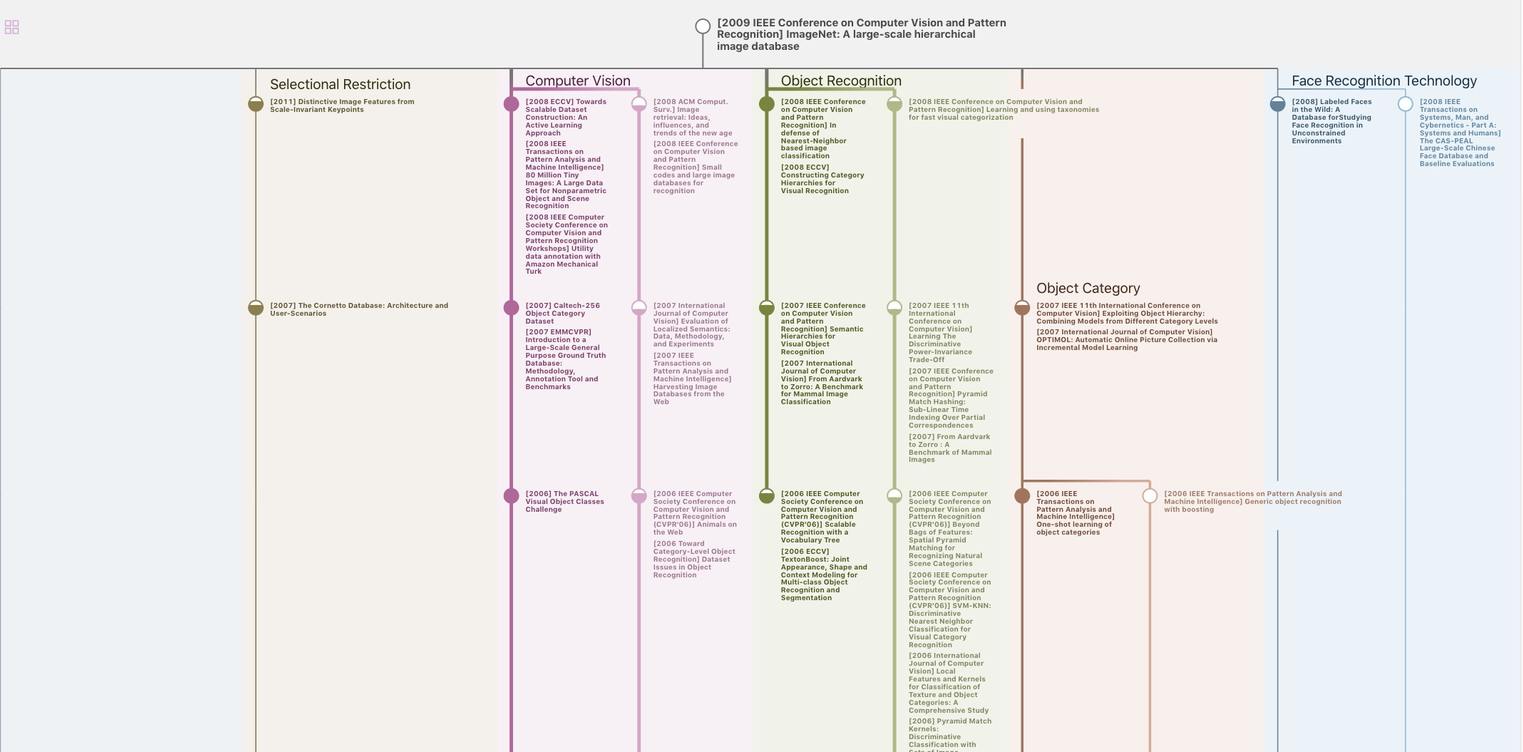
生成溯源树,研究论文发展脉络
Chat Paper
正在生成论文摘要