Experiment Design with Gaussian Process Regression with Applications to Chance-Constrained Control
2023 62ND IEEE CONFERENCE ON DECISION AND CONTROL, CDC(2023)
摘要
Learning for control in repeated tasks allows for well-designed experiments to gather the most useful data. We consider the setting in which we use a data-driven controller that does not have access to the true system dynamics. Rather, the controller uses inferred dynamics based on the available information. In order to acquire data that is beneficial for this controller, we present an experimental design approach that leverages the current data to improve expected control performance. We focus on the setting in which inference on the unknown dynamics is performed using Gaussian processes. Gaussian processes not only provide uncertainty quantification but also allow us to leverage structures inherit to Gaussian random variables. Through this structure, we design experiments via gradient descent on the expected control performance with respect to the experiment input. In particular, we focus on a chance-constrained minimum expected time control problem. Numerical demonstrations of our approach indicate our experimental design outperforms relevant benchmarks.
更多查看译文
AI 理解论文
溯源树
样例
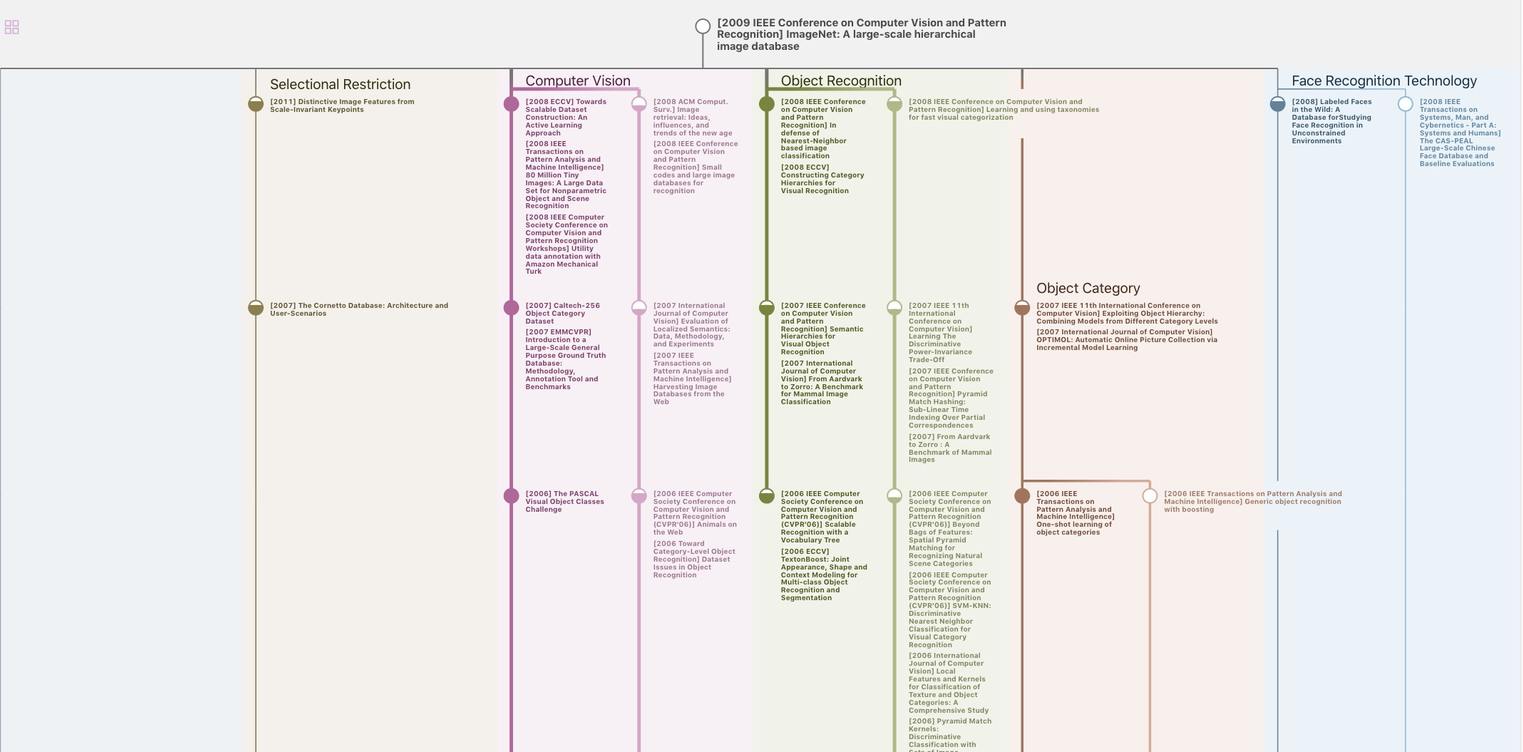
生成溯源树,研究论文发展脉络
Chat Paper
正在生成论文摘要