Scalable Robust Multi-Agent Reinforcement Learning for Model Uncertainty
2023 62ND IEEE CONFERENCE ON DECISION AND CONTROL, CDC(2023)
摘要
A robust multi-agent reinforcement learning (MARL) algorithm using a nature actor has been shown to be effective in finding a robust Nash equilibrium (NE) of a Markov game with model uncertainty. However, since a game-size scaling increases the search space and challenges reaching the NE, the robust property of the algorithm is reduced in environments with many agents. This paper proposes an evolutionary diversity-maintaining population curriculum (EDPC) framework with a robust attention-based multi-agent deep deterministic policy gradient (RA-MADDPG) algorithm, which enables an efficient robust NE search by a structured search space expansion. In the EDPC framework, the MARL divides into several stages, and when moving on to the next stage, a population consisting of larger games is made with two parent games from the previous stage. We introduce rewardproportionate parent selection and reward-guided mutation methods to continue reinforcing superior agents and maintain the diversity of the population. Furthermore, the RA-MADDPG is used to solve the robust Markov game at each stage with nature actors with attention-based architectures. The scalability and robustness of the proposed method are evaluated for different numbers of agents and levels of model uncertainty.
更多查看译文
AI 理解论文
溯源树
样例
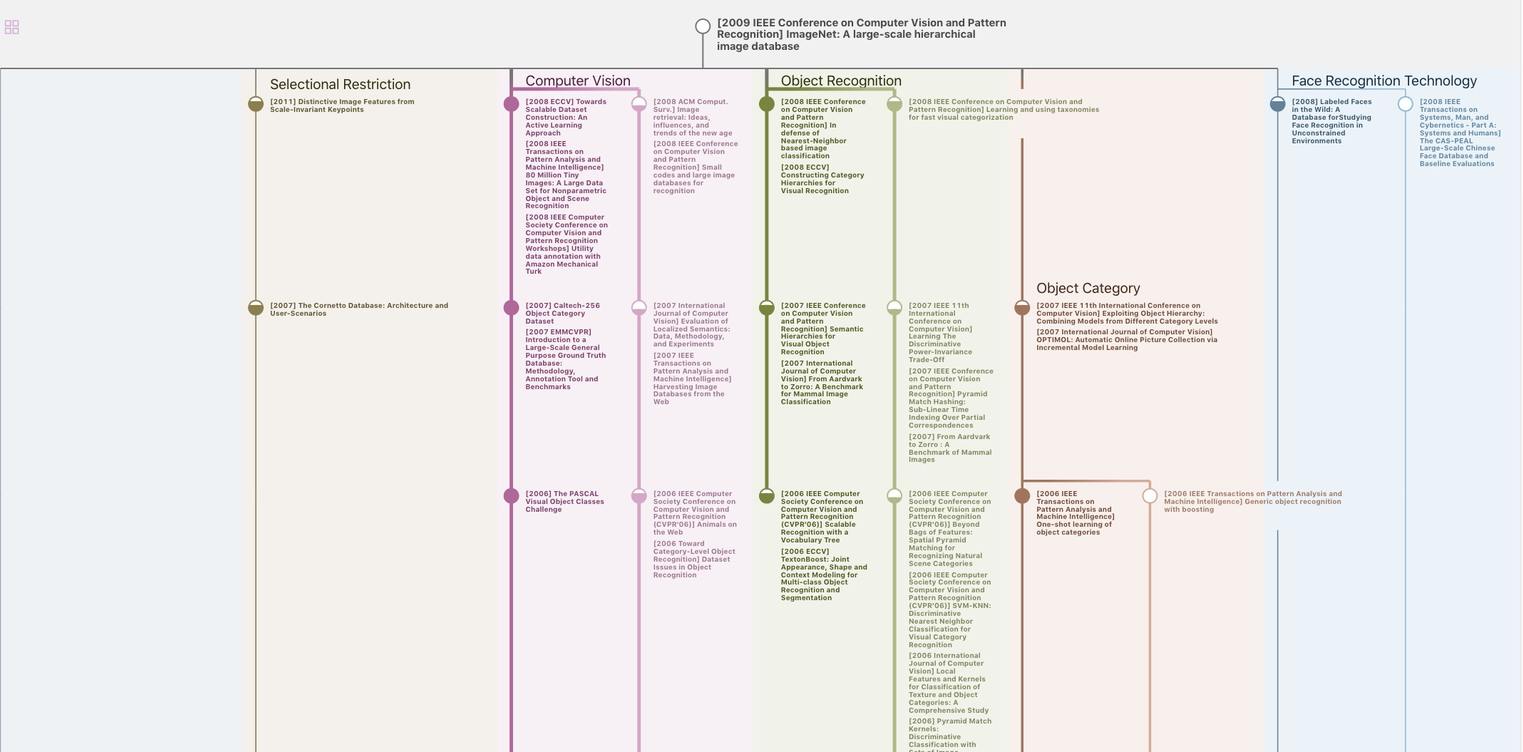
生成溯源树,研究论文发展脉络
Chat Paper
正在生成论文摘要