Lung Cancer Detection via Federated Learning.
2023 IEEE International Conference on Bioinformatics and Biomedicine (BIBM)(2023)
摘要
Lung cancer is one of the most common cancers worldwide. In 2020, there were an estimated 2.2 million new cases of lung cancer. It is often diagnosed at an advanced stage because in the early stages, it does not present particularly abnormal symptoms, such as cough and chest pain, which are often underestimated. Typically, it is detected through pronounced symptoms that lead to more in-depth diagnostic examinations or through other routine tests that reveal its presence almost by chance. The tools used for detection include chest X-rays, CT scans, and PET scans. Analyzing these allows the detection of abnormal formations in the early stages. From this perspective, artificial intelligence has set the goal, in recent years, of providing tools for image analysis capable of automatically, rigorously, and above all, promptly providing an initial classification of the detected formations. In particular, in the literature, it is possible to find various solutions that make use of Federated Learning. This method involves training deep learning models on expansive datasets distributed across multiple data centers. Significantly, it safeguards privacy by obviating the need to transmit sensitive patient data. The objective of this paper is to examine existing state-of-the-art solutions, emphasizing workflows and essential strategies. It considers the datasets employed and architectural decisions. The analysis extends to common issues encountered in the studied works, with a specific focus on challenges typical of the medical domain. The paper concludes by exploring potential future solutions to address these issues.
更多查看译文
关键词
Federated Learning,Medical Imaging,Lung Cancer Diagnosis
AI 理解论文
溯源树
样例
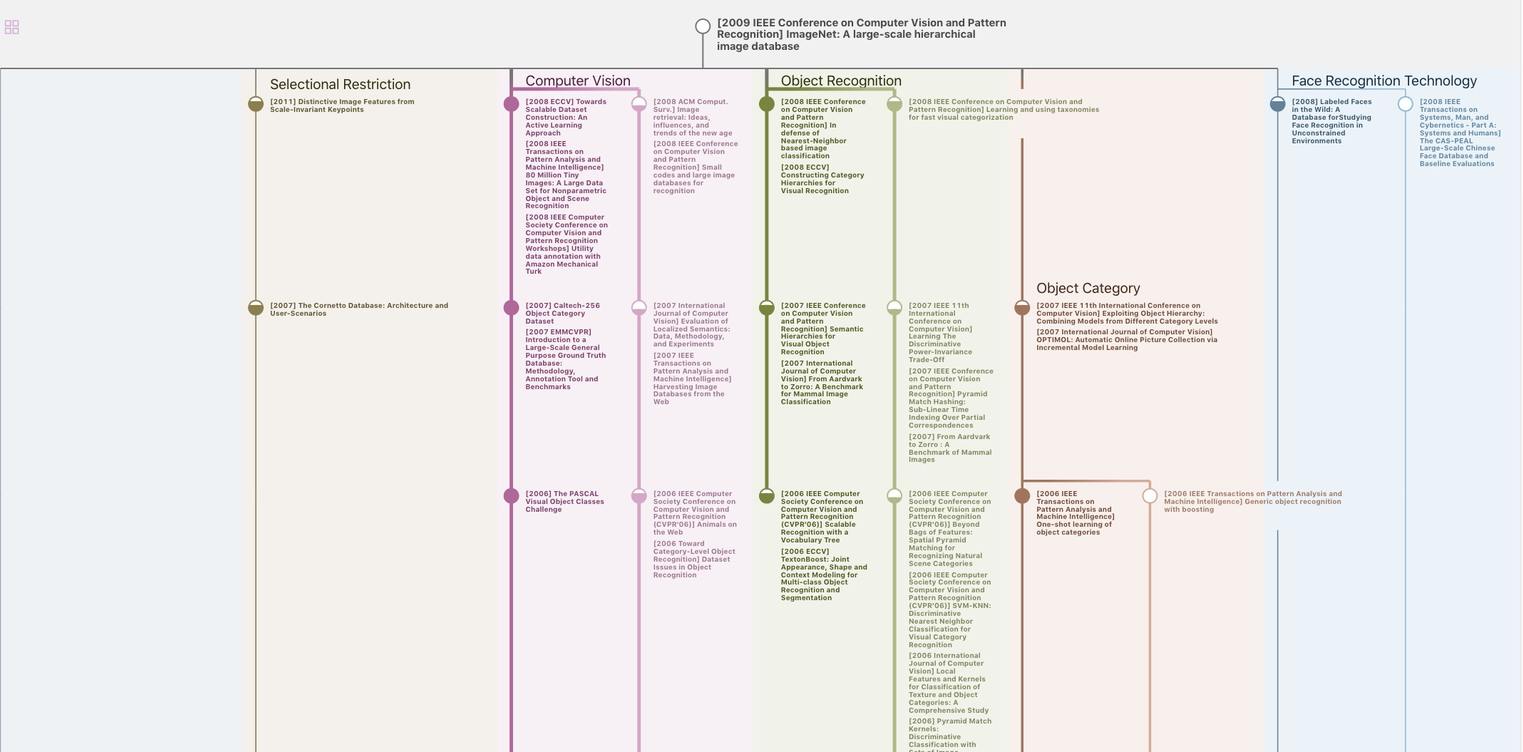
生成溯源树,研究论文发展脉络
Chat Paper
正在生成论文摘要