MT-LGSGCN: EEG-Based Emotion Recognition Using Multi-scale Temporal and Local-Global Spatial Graph Convolution Network.
2023 IEEE International Conference on Bioinformatics and Biomedicine (BIBM)(2023)
摘要
Recent research has shown that different cognitive tasks and processes involve coordinated activity among multiple brain regions. The diverse topological structures of the brain network describe the connectivity patterns and interaction modes between different brain regions. In order to study the brain activity associated with different interaction types in brain networks, we propose a novel graph neural network called MT-LGSGCN to learn the local-global graph representation of electroencephalogram (EEG) signals under different brain network topologies. The input layer of MT-LGSGCN consists of multi-scale one-dimensional convolutional kernels, which are used to capture the temporal-frequency dynamics of EEG signals and serve as inputs for local and global graph convolutions. MT-LGSGCN employs purely learnable sets of additional adjacency matrices to model the inter-region correlations, enabling the aggregation of high-level features with multiple topological structures. Through comparative experiments with other state-of-the-art methods, MT-LGSGCN achieves superior performance on the DEAP dataset. The results demonstrate that incorporating different brain network topologies into the design of graph neural networks can improve the final classification performance.
更多查看译文
关键词
emotion recognition,affective computing,deep learning
AI 理解论文
溯源树
样例
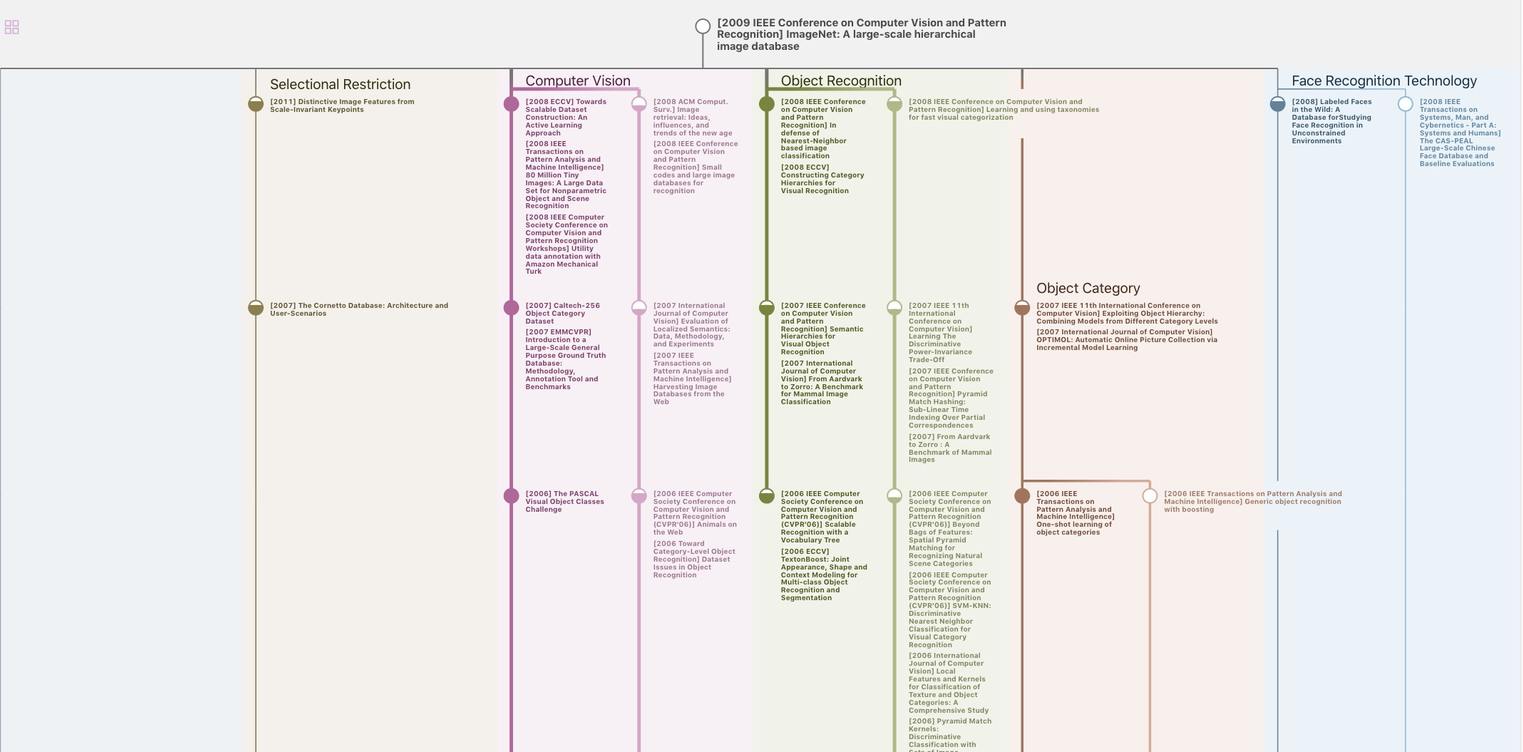
生成溯源树,研究论文发展脉络
Chat Paper
正在生成论文摘要