Progressive Fourier Transform (PFT): Enhancing Time-Frequency Representation of EEG signals for Stress and Seizure Detection.
2023 IEEE International Conference on Bioinformatics and Biomedicine (BIBM)(2023)
摘要
The detection and classification of neurological and psychological phenomena heavily rely on Electroencephalography (EEG). This study investigates the effectiveness of various feature extraction techniques and machine learning classifiers in EEG-based classification tasks. Stress detection using the Bird et al. dataset, which encompasses multiple emotional states, and seizure detection using the CHB-MIT dataset, known for its challenges in distinguishing seizure from non-seizure patterns, are specifically explored.The results highlight the crucial role of feature extraction methods in EEG-based classification. Among the techniques tested, our Progressive Fourier Transform (PFT) method consistently outperforms others, emerging as the superior choice.In stress detection, our proposed PFT achieves an outstanding accuracy of 98.41% on the Bird et al. dataset, surpassing existing methods based on statistical features. For seizure detection, our model attains a competitive accuracy of 96.88% on the CHBMIT dataset, showcasing efficiency even with a reduced number of channels.This study demonstrates the potential of EEG-based classification techniques in practical applications such as stress monitoring and seizure prediction. Furthermore, it emphasizes the significance of advanced feature extraction methods in achieving accurate results. Future research may involve refining these techniques further and expanding their applicability to diverse EEG datasets and other neurological and psychological disorders.
更多查看译文
关键词
Stress,Epilepsy,Progressive Fourier Transform (PFT),Electroencephalogram (EEG),Medical Diagnosis
AI 理解论文
溯源树
样例
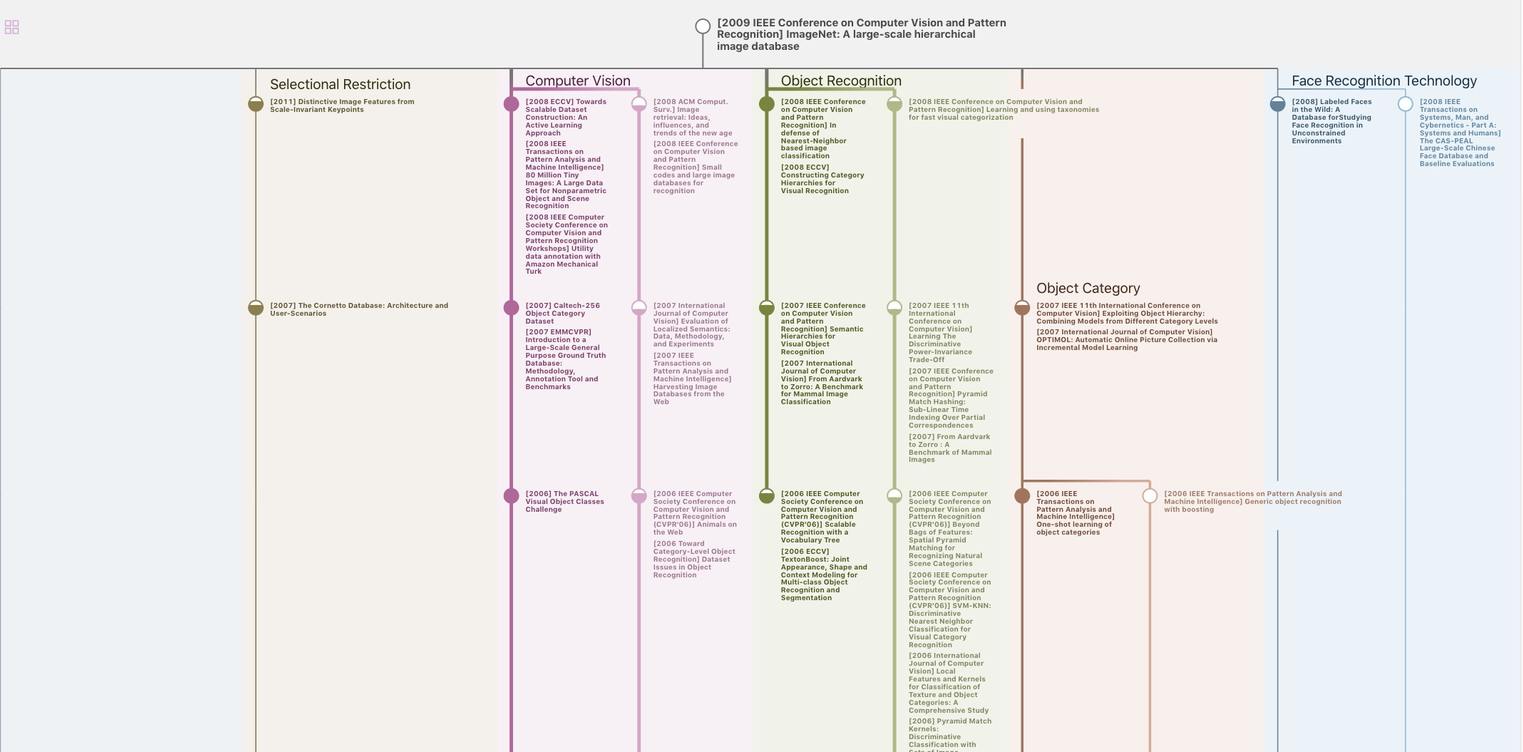
生成溯源树,研究论文发展脉络
Chat Paper
正在生成论文摘要