A Practical Approach to Disease Risk Prediction: Focus on High-Risk Patients via Highest-k Loss.
2023 IEEE International Conference on Bioinformatics and Biomedicine (BIBM)(2023)
摘要
Disease risk prediction models play an important role in preventing disease developments in modern healthcare. However, the lack of focus on high-risk patients has hindered the large-scale practical application of these models, especially considering the limitation of medical resources available for following up on patients who are deemed high-risk. In this study, we propose a novel and practical approach that focuses on minimizing the number of false positive observations among high-risk patients by introducing the Highest-k Loss. The solution is to estimate the weights of the highest k scores with a differentiable estimation of the sorting operation and apply the weights to the loss function. We extracted 253,680 survey responses from a public dataset of the U.S. health survey system to define a diabetes prediction task. This study employs nested cross-validation as well as an aggregated model applied to an independent test set to systematically evaluate the proposed method. Compared with traditional binary cross entropy loss and Focal loss, the Highest-k loss improved the precision (positive predictive value) for the highest 1% scores by 0.05 (95% CI: 0.041-0.055), the highest 5% scores by 0.03 (95% CI: 0.024-0.032), and the highest 10% scores by 0.02 (95% CI: 0.016-0.021). The introduced Highest-k loss function addresses the problem of prevailing risk prediction models and offers a practical solution that focuses on patients with the k highest predictive scores who can realistically receive an intervention as opposed to the entire patient population.
更多查看译文
关键词
disease risk prediction,highest risk,Highest-k Loss,soft sorting,highest k scores
AI 理解论文
溯源树
样例
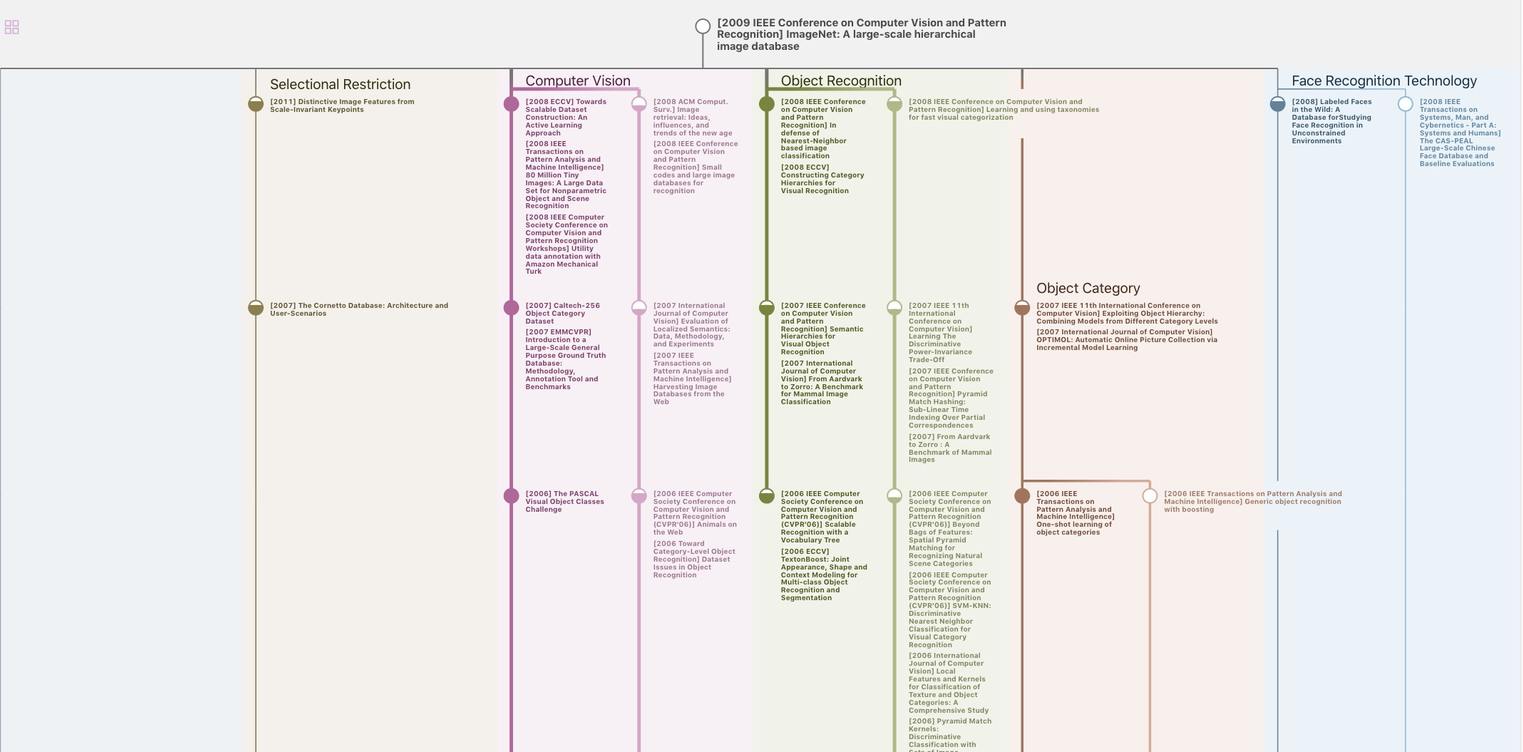
生成溯源树,研究论文发展脉络
Chat Paper
正在生成论文摘要