Counterfactual Inference-based Data Augmentation for Drug-side effect Associations Prediction.
2023 IEEE International Conference on Bioinformatics and Biomedicine (BIBM)(2023)
摘要
Detecting drug side effects is crucial in development of drugs. As publicly available biomedical data expands, researchers have devised numerous computational methods for predicting drug-side effect associations (DSAs). Among these, network-based approaches have gained significant attention in the biomedical field. However, the challenge of data scarcity poses a significant hurdle for existing DSAs prediction models. While various data augmentation methods have been created to solve the proble, most rely on random alterations to the original networks, neglecting the causality of DSAs’ existence, thus impacting the predictive performance negatively. In this paper, we introduce a counterfactual inference-based data augmentation method to enhance performance. First,a heterogeneous information network (HIN) is construct by integrating multiple biomedical data sources. We employ community detection on the HIN to preform a counterfactual inference-based method, deriving augmented links and an augmented HIN. Subsequently, we apply a meta-path-based graph neural network to obtain high-quality representations of drugs and side effects, enabling the prediction of DSAs. Our comprehensive experiments confirm the effectiveness of this counterfactual inference-based data augmentation for DSAs prediction.
更多查看译文
关键词
counterfactual inference,data augmentation,drug-side effect associations prediction,heterogeneous information network
AI 理解论文
溯源树
样例
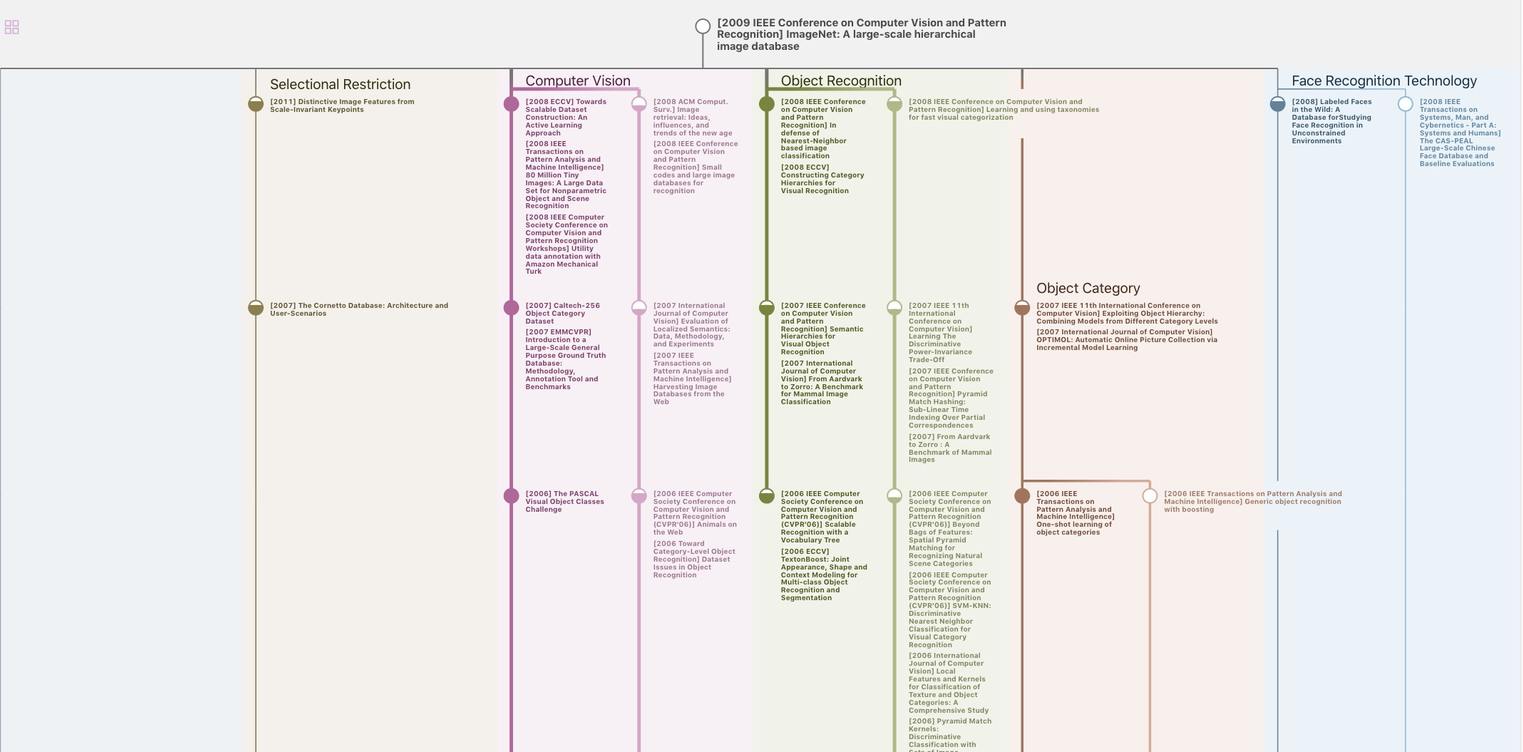
生成溯源树,研究论文发展脉络
Chat Paper
正在生成论文摘要