Identification of Single-Cell RNA Sequencing Molecular Signatures for COVID-19 Infection Severity Classification.
2023 IEEE International Conference on Bioinformatics and Biomedicine (BIBM)(2023)
摘要
In this study, we propose a graph-based framework to identify scRNA-seq molecular signatures for COVID-19 infection severity identification. We conduct extensive experiments on scRNA-seq data from bronchoalveolar lavage fluid (BALF) with four machine learning models: Support Vector Machine, Random Forest, Graph Convolutional Network (GCN), and Graph Attention Network (GAT). In addition, we employ an explainable artificial intelligence approach, GNNExplainer, to interpret model predictions by identifying the top 15 features that contribute to the severity classification. Our finding suggests that graphical models could accurately distinguish healthy people from COVID-19 patients (F1-score > 0.9) based on patient scRNA-seq data, and traditional machine learning approaches could accurately distinguish COVID-19 patients with different severity (F1-score > 0.99), along with meaningful molecular signatures identification and discovery. Our implementation is available on a github repository: https://github.com/Da2f1eW/COVID19_Infection_Severity_Classification.
更多查看译文
关键词
COVID-19,single-cell RNA sequencing,classification,model interpretation,pathway analysis
AI 理解论文
溯源树
样例
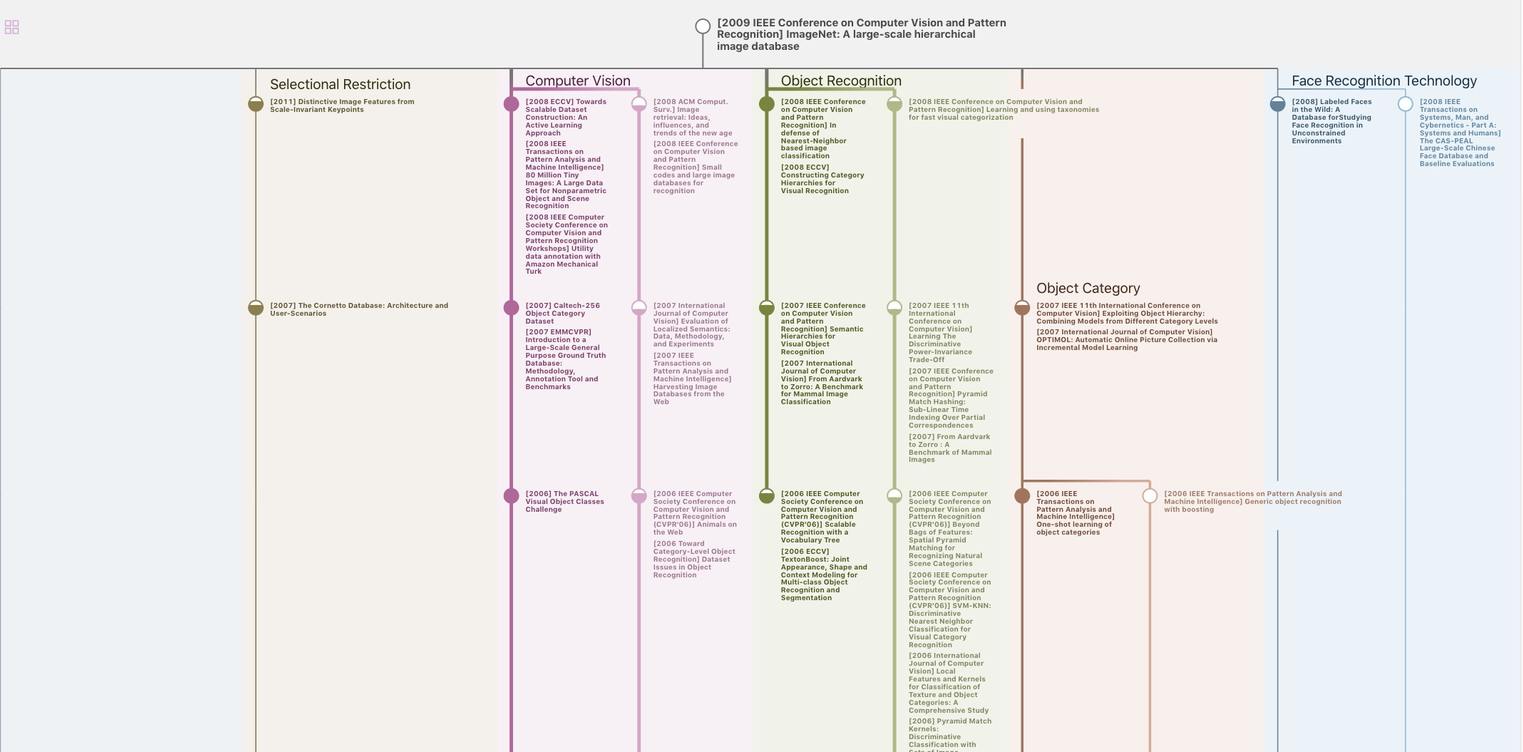
生成溯源树,研究论文发展脉络
Chat Paper
正在生成论文摘要