Identifying Main and Epistasis Effects of Genetic Variations on Neuroimaging Phenotypes Using Effective Feature Interaction Learning.
2023 IEEE International Conference on Bioinformatics and Biomedicine (BIBM)(2023)
摘要
Brain imaging genetics investigates the complex relationships between genetic variations and brain imaging quantitative traits (QTs). However, existing approaches primarily focus on the main effects of genetic variations, potentially neglecting the crucial role of epistasis that explains the missing heritability of brain disorders. Unfortunately, this issue has been largely unexploited. In this paper, to fill this gap, we present Multi-Task feature interaction-aware Sparse Canonical Correlation Analysis (MTfiSCCA) to identify disease-related main effect and epistasis of risk genetic factors on multimodal neuroimaging phenotypes simultaneously. To ensure stability and interpretation, we use innovative sparsity-inducing penalties to identify biomarkers that make significant contributions. Additionally, we develop an efficient optimization algorithm to solve the proposed method, which converges to a local optimum. Experimental results on the Alzheimer’s disease neuroimaging initiative (ADNI) dataset show that our MTfiSCCA method achieves higher canonical correlation coefficients (CCC) and better feature selection subsets such as disease-related biomarkers compared to the state-of-the-art methods. Furthermore, MTfiSCCA reveals interpretable epistasis among genetic variations implicated in AD, offering novel insights into the underlying pathogenic mechanisms of brain disorders such as Alzheimer’s disease (AD).
更多查看译文
关键词
Brain imaging genetics,genotype-phenotype correlation,biomarker identification,gene-gene interactions
AI 理解论文
溯源树
样例
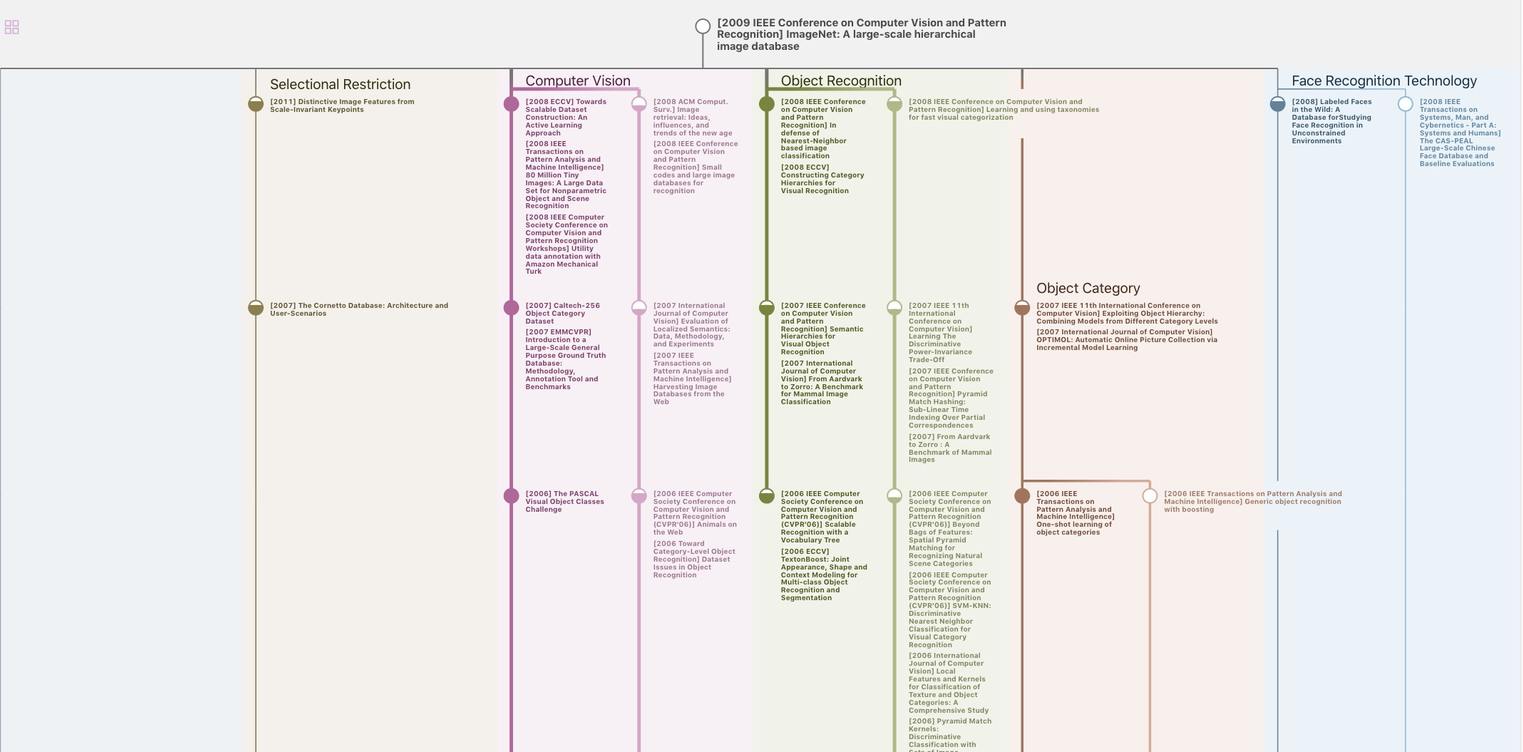
生成溯源树,研究论文发展脉络
Chat Paper
正在生成论文摘要