HSELDA: Heterogeneous Sub-Graph Learning for lncRNA-Disease Associations Prediction.
2023 IEEE International Conference on Bioinformatics and Biomedicine (BIBM)(2023)
摘要
Numerous studies have shown that long non-coding RNAs (lncRNAs) can be the key factors in causing a variety of human diseases, such as cancers. Predicting the potential associations between lncRNAs and diseases can be highly beneficial for pathogenesis investigation and understanding the functionalities of lncRNAs in disease development. Existing computational methods for lncRNAs-disease association (LDA) prediction focuses on integrating heterogeneous information from given data including associations of various biological elements. However, many of them do not fully utilize the topological information obtained from graph-structured frameworks, such as heterogeneous graphs consisting of different biological variables including lncRNA, disease, miRNA, and others. We proposed a novel method, named HSELDA, that utilized a relational graph convolutional neural network based on a link prediction technique via subgraphs extraction to predict the potential lncRNAs-disease associations. In this method, we first constructed a heterogeneous graph consisting of three types of nodes: lncRNAs, miRNAs, and disease, with their known associations between each node pair as edges. Then, we extracted a list of local subgraphs around each target link, where the subgraphs preserve rich heuristic information related to link existence with the neighbor nodes directly connected to the target links. We employed a relational graph convolutional network to identify the most promising lncRNAs-diseases associations based on local subgraphs. The experimental results showed that HSELDA was superior to several state-of-the-art methods. Of note, our proposed model shows significant superiority over other compared baseline approaches in AUPRC.
更多查看译文
关键词
lncRNA-disease associations,Graph neural network,Subgraph,Link prediction
AI 理解论文
溯源树
样例
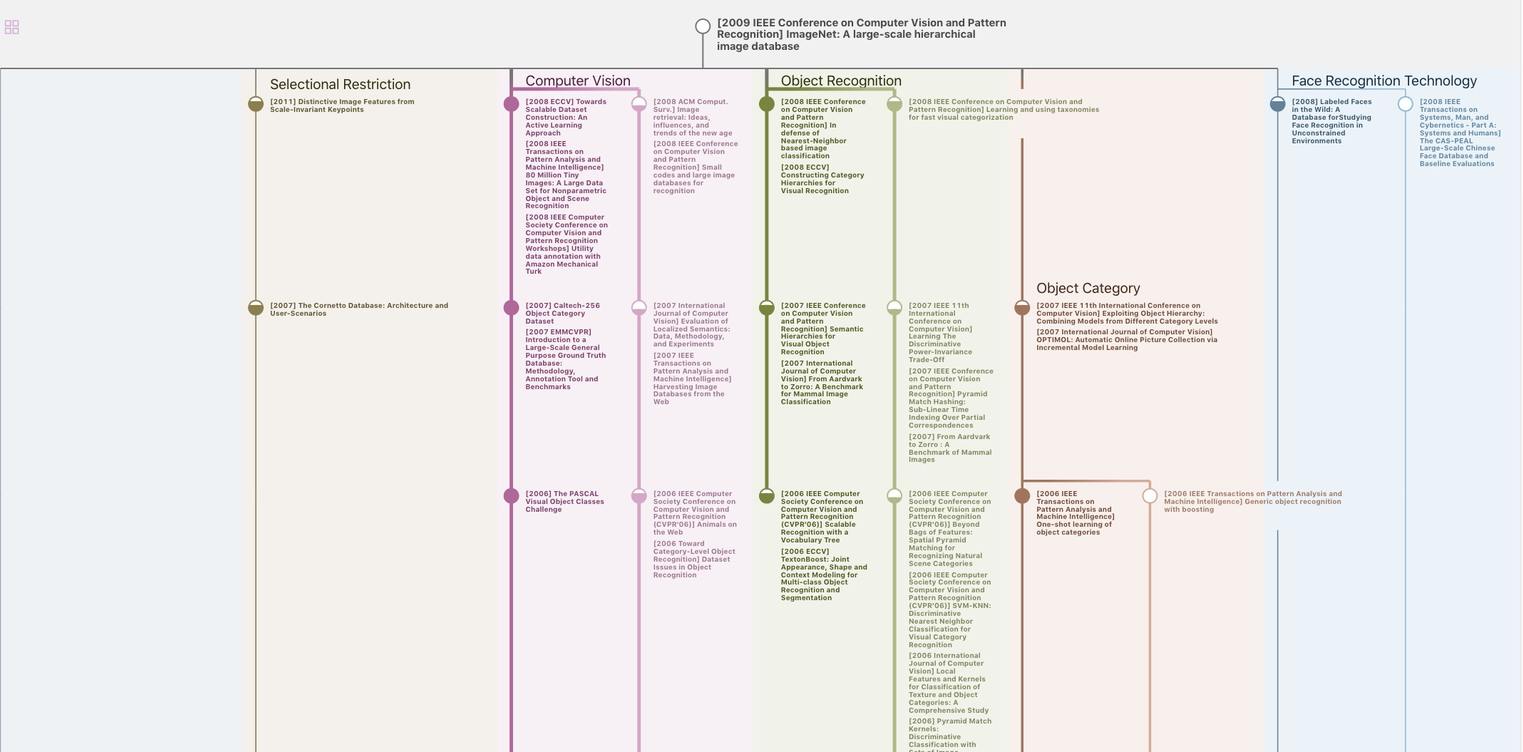
生成溯源树,研究论文发展脉络
Chat Paper
正在生成论文摘要