HC-MAE: Hierarchical Cross-attention Masked Autoencoder Integrating Histopathological Images and Multi-omics for Cancer Survival Prediction.
2023 IEEE International Conference on Bioinformatics and Biomedicine (BIBM)(2023)
摘要
Accurate cancer survival prediction enables clinicians to tailor treatment regimens based on individual patient prognoses, effectively mitigating over-treatment and inefficient medical resource allocation. Recently, the integration of histopathological images and multi-omics data, together with deep learning, has become increasingly applied to predict cancer survival. However, current deep learning-based integration methods ignore the spatial relationships across various fields of view within gigapixel histopathological images, since they mainly focus on a specific field of view. Inspired by the hierarchical image pyramid transformer (HIPT), we propose a hierarchical cross-attention masked autoencoder (HC-MAE) to integrate histopathological images and multi-omics data for cancer survival prediction. Specifically, HC-MAE aggregates the representations learned from different fields of view, effectively capturing the fine-grained details and the spatial relationships within histopathological images. We conduct experiments to compare the HC-MAE method with current state-of-the-art methods on six cancer datasets sourced from The Cancer Genome Atlas (TCGA). The experimental results demonstrate that HC-MAE achieves superior performance on five out of six cancer datasets, significantly outperforming the compared methods. The code is available at https://github.com/SuixueWang/HC-MAE.
更多查看译文
关键词
Hierarchical Cross-attention,Masked Autoencoder,Histopathological Image,Multi-omics,Survival Prediction
AI 理解论文
溯源树
样例
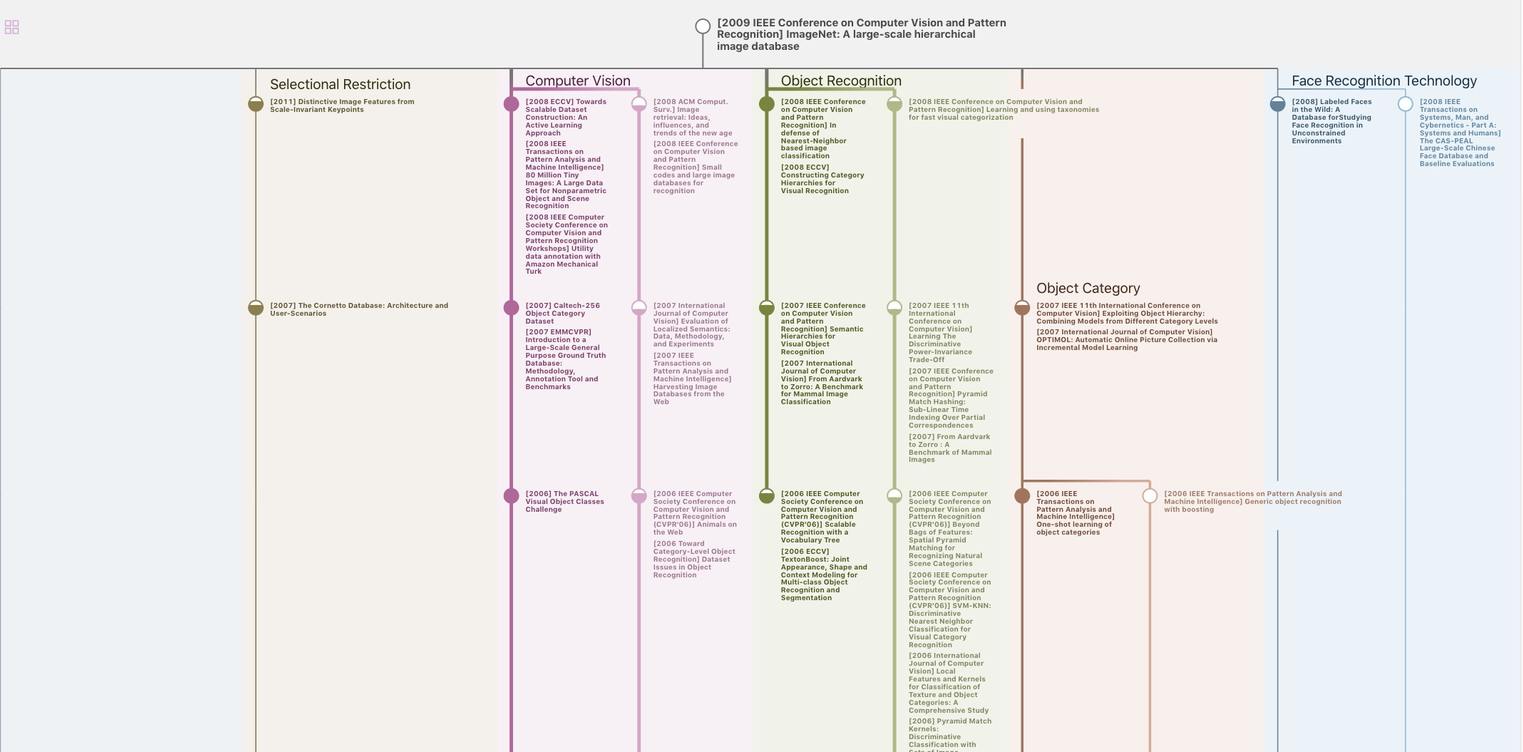
生成溯源树,研究论文发展脉络
Chat Paper
正在生成论文摘要