Not All Errors Are Created Equal: Evaluating The Impact of Model and Speaker Factors on ASR Outcomes in Clinical Populations.
2023 IEEE Automatic Speech Recognition and Understanding Workshop (ASRU)(2023)
摘要
Pathological speech analysis with Automatic Speech Recognition (ASR) is a long-standing research domain based on the expectation that there is a close relationship between pathological speech abnormalities and reduced ASR performance. This has led to interest in ASR-based clinical analyses such as intelligibility assessments and disfluency detection. However, these analyses are premised on transcriptions that accurately reflect abnormalities. We find that the choices of ASR model and speech task significantly affect transcription errors for both typical and pathological speech, which is reflected in the word error rate (WER). Notably, language restriction (e.g., dictionary of words) decreases a model’s ability to capture abnormalities while more complex tasks increase both model and speaker-driven transcription errors. These findings highlight possible variability in clinical analysis with ASR and suggest the importance of controlling for model type and task complexity.
更多查看译文
关键词
speech analysis,speech recognition,pathological speech,clinical analysis
AI 理解论文
溯源树
样例
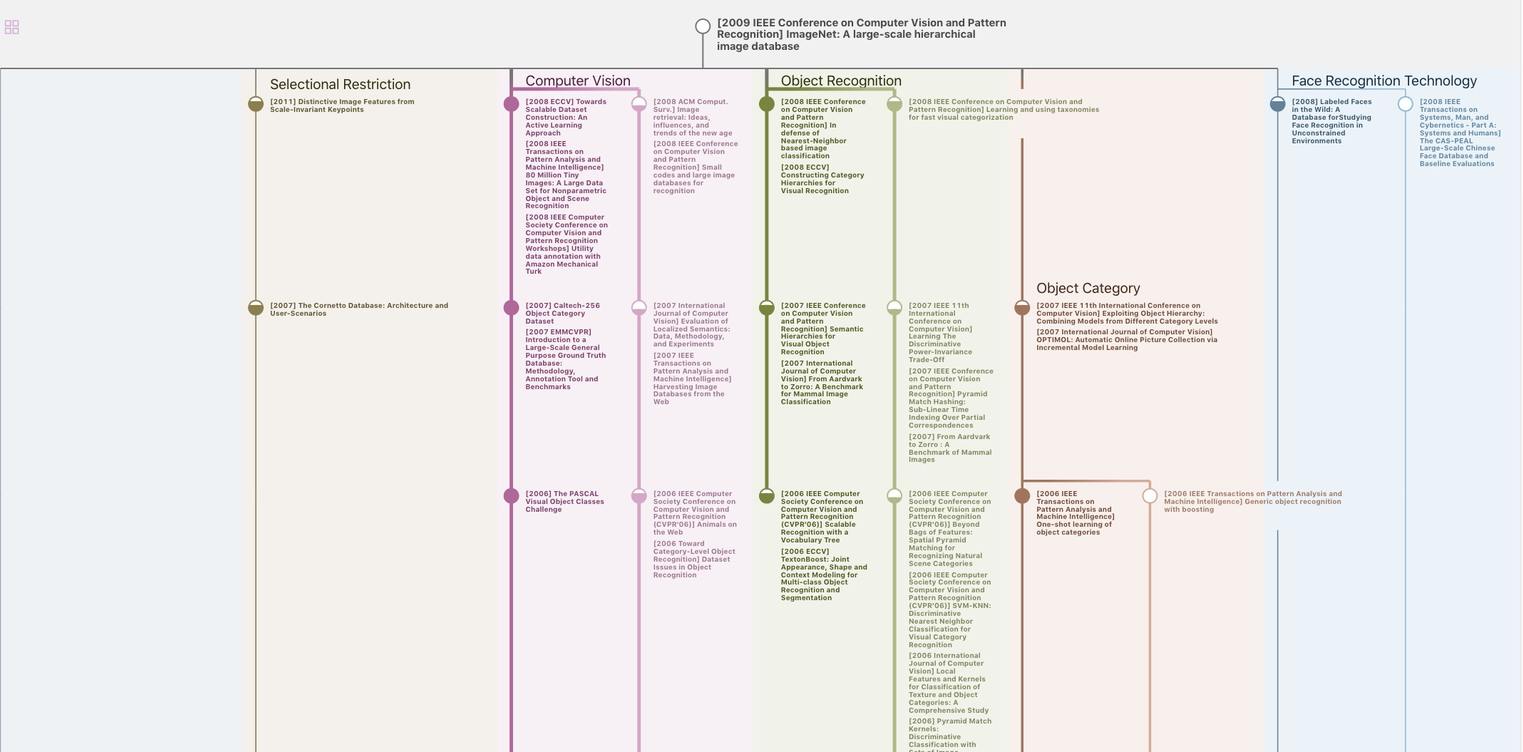
生成溯源树,研究论文发展脉络
Chat Paper
正在生成论文摘要